PDA: Progressive Domain Adaptation for Semantic Segmentation
KNOWLEDGE-BASED SYSTEMS(2024)
摘要
The unsupervised domain adaptation semantic segmentation task is challenging due to the distribution shift problem between the source and the target domains. In this paper, we provide a novel perspective to address this problem. Currently, in the literature, it is shown that output-level domain adaptation networks (OL-DAN) can generate outputs with smaller distribution shift. Motivated by this phenomenon, a Progressive Domain Adaptation (PDA) framework is proposed, which uses the outputs generated by OL-DAN as the auxiliary domain images to progressively align the distribution between the source and target domains. Unlike existing two-stage input-level domain adaptation methods which use an image translation network as a standalone model to generate the auxiliary domain images, the PDA is an end-to-end framework that contains an OL-DAN and a domain fusion domain adaptation network (DF-DAN). The OL-DAN aims to gradually generate the outputs with smaller distribution shift and more accurate semantic structures as the auxiliary domain images in every iteration optimization. The DF-DAN is proposed to further mine the domain-invariant information from the auxiliary images and then fuse the features learned from the original images and the auxiliary images to obtain a richer representation. Finally, the distribution between the source and target domains is aligned to optimize the OL-DAN and DF-DAN. Experiments demonstrate that the proposed PDA achieves superior performance on three cross-domain semantic segmentation benchmarks.
更多查看译文
关键词
Domain adaptation,Semantic segmentation,Progressive alignment,Auxiliary domain
AI 理解论文
溯源树
样例
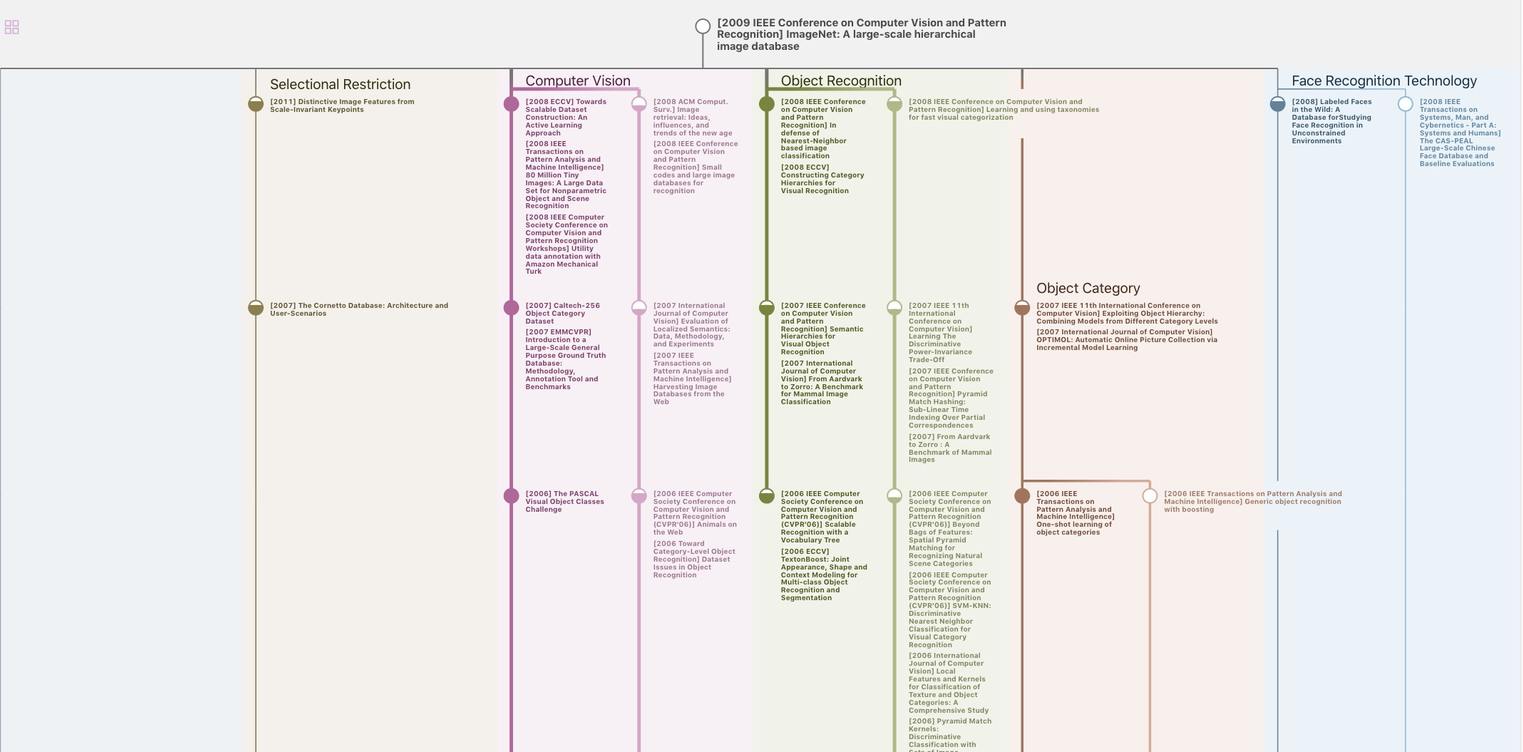
生成溯源树,研究论文发展脉络
Chat Paper
正在生成论文摘要