Risk predicting for acute coronary syndrome with conventional and unstable plaque radiomics features from serial coronary computed tomography angiography
European Heart Journal(2023)
摘要
Abstract Background Plaques with high-risk features are responsible for most acute cardiac events. However, there are still some lesions with high-risk plaque (HRP) morphology, which will not lead to cardiac events over time. It is crucial to precisely identify features of unstable plaque and to carry out individualized treatment, eventually aiming to reduce the likelihood of plaque rupture and lower the incidence of acute coronary events. Besides, the development of quantitative radiomics in recent years may further help with the diagnostic and predictive performance of coronary CTA. Purpose To establish machine learning models by combining traditional coronary CTA features of high-risk plaque and radiomics features of unstable plaque, so as to improve the efficiency of models and to predict acute cardiac events precisely. Methods A total of 133 elderly patients (407 lesions) were retrospectively enrolled in this cohort study who underwent serial CCTA with the interval ≥ 2 years between scans. The patients were divided into two groups according to the occurrence of acute coronary events within 1 year after coronary CTA scanning . We recorded anatomical characteristics, high-risk plaque characteristics, and acute coronary events of the elderly patients. Based on the coronary CTA image cohort, we applied 3D Slicer software to segment target lesions, delineate regions of interest, and extract radiomics features. After that, we used LASSO regression to reduce the dimensionality of the radiomics features, and finally screened out unstable radiomics features. Combining the HRP features with the selected radiomics features, we established a prediction model of acute cardiac events with machine learning method. The area under the receiver operating characteristic (ROC) curve (AUC) was analyzed to compare the classification ability of conventional and radiomics models in the validation set. Results 133 elderly patients were included, with an average age of 65.8 ± 5.3 years. A novel model has been established by combining HRP features and 4 selected unstable radiomics features (2 wavelet features, 1 shape feature and 1 GrayLevel feature) with SVM machine learning method. Compared with conventional HRP model, the novel model displayed a significantly improved predictive performance in the validation set (AUC 0.784 vs. 0.624, P < 0.001). Conclusions We have established a novel model by combining the traditional HRP features of coronary CTA and radiomics features of unstable plaque, assisting in better prediction of acute coronary events in elderly . Compared with traditional HRP feature model, the prediction efficiency of novel model was significantly improved.
更多查看译文
关键词
unstable plaque radiomics features,acute coronary syndrome,computed tomography
AI 理解论文
溯源树
样例
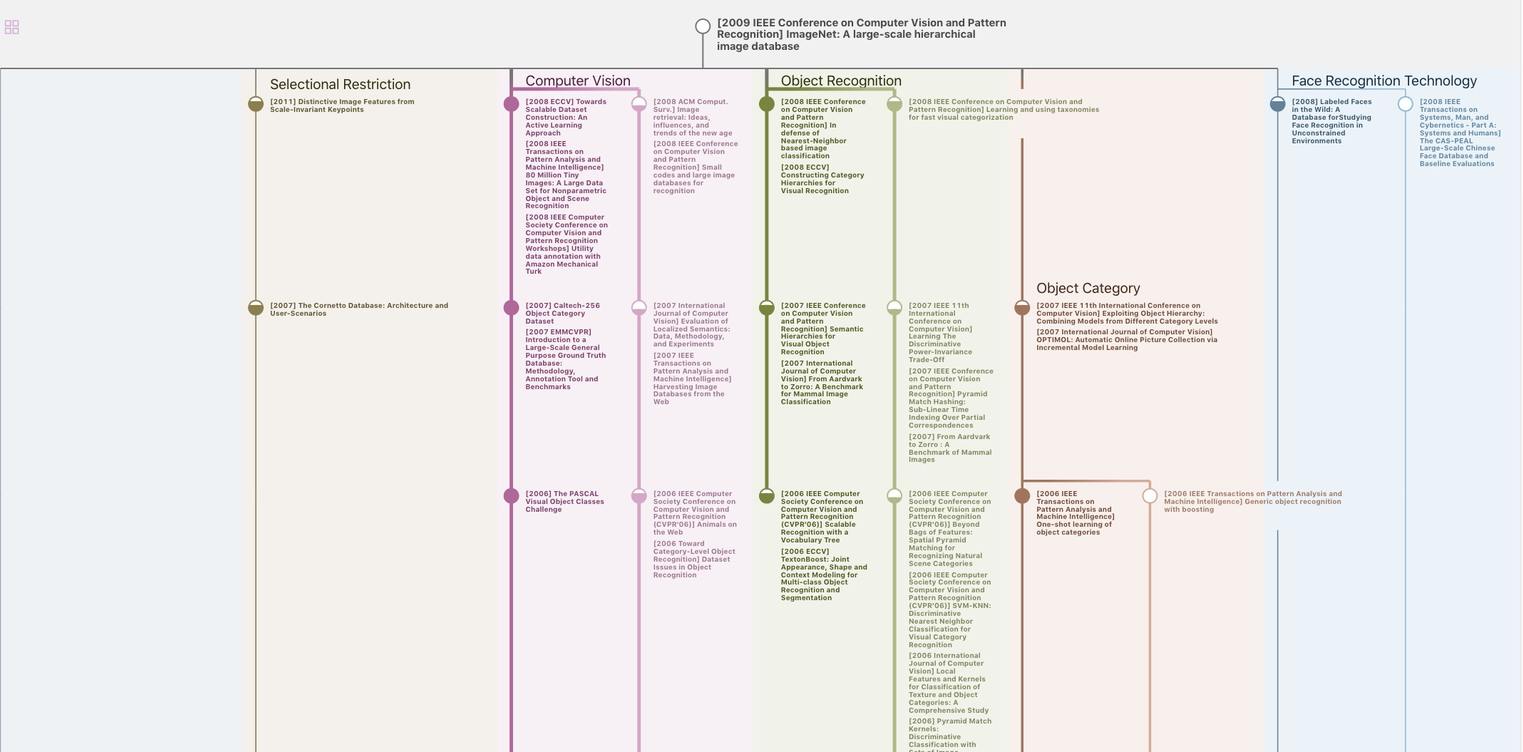
生成溯源树,研究论文发展脉络
Chat Paper
正在生成论文摘要