Machine learning score focused only on echocardiographic data to predict in-hospital outcomes in ICCU patients. A study from the ADDICT ICCU cohort
European Heart Journal(2023)
摘要
Abstract Background While several studies have shown the strong prognostic value of several transthoracic echocardiography (TTE) parameters in patients admitted in intensive cardiac care unit (ICCU), the interest of a severity score integrating these parameters has not been established. Machine learning (ML) represents an innovative and accurate statistical method to embed TTE parameters into a scoring system. Purpose To investigate the feasibility and accuracy of ML integrating TTE parameters measured at admission in ICCU to predict in-hospital major adverse cardiovascular events (MACE) and to compare its performance with existing scores. Methods In April 2021, 1,499 patients admitted to 39 French ICCU were included in the ADDICT-ICCU Cohort (NCT05063097). A comprehensive TTE was performed within the first 24 hours of admission. Twenty TTE parameters were systematically collected. ML involved automated feature selection by Boruta to select variables and prevent model overfitting. Several ML models (LASSO, Random Forest and XGBoost) were then trained on 70% of patients and evaluated on the other 30% as internal validation. Their performances were compared against standard logistic regression model, using receiver operating characteristics (ROC) curves, precision-recall (PR) curves and area-under-the curves (AUC). The primary composite outcome was in-hospital MACEs defined by all-cause mortality, cardiogenic shock (requiring medical or mechanical haemodynamic support) and resuscitated cardiac arrest. The external validation cohort of the ML score was performed in another center on 300 patients. Results Of 1,499 consecutive patients screened (63±15 years, 70% male), MACEs occurred in 67 patients (4.5%) including: 27 (1.8%) deaths, 38 (2.53%) cardiogenic shocks, and 14 (0.9%) resuscitated cardiac arrests. To build the ML models, 5 TTE parameters were selected as being the most important in predicting MACEs: LVOT VTI, E/e’ ratio, sPAP, TAPSE, and LVEF. The XGBoost model showed the best performance compared with the other ML models (AUROC for XGBoost: 0.83, Random-forest: 0.81, LASSO regression 0.79 and to a regression model: 0.76). Using the XGBoost model as our final ML-score, SHAP values for the parameters were: 0.047 for LVOT VTI, 0.041 for E/e’ ratio, 0.028 for SPAP, 0.021 for TAPSE and 0.01 for LVEF. Our ML-score exhibited a higher AUC compared with existing scores (TIMI, GRACE, qSOFA, Acute-HF) (Figure 1), and an incremental prognostic value for predicting in-hospital MACEs over and above clinical or biological data (Chi-square 59.72 p<0.001; C-Index 0.8 [0.71;0.89], p=0.012) (Figure 2). Conclusion The ML score including only TTE parameters exhibited a higher prognostic value to predict in-hospital MACE compared with any other existing traditional methods in patients admitted in ICCU. This ML score could be implemented in clinical practice given its ease of use (only 5 TTE parameters to analyze) and its prognostic implication.Figure 1.PerformanceFigure 2.Prognostic value
更多查看译文
关键词
echocardiographic data,iccu patients,machine learning,in-hospital
AI 理解论文
溯源树
样例
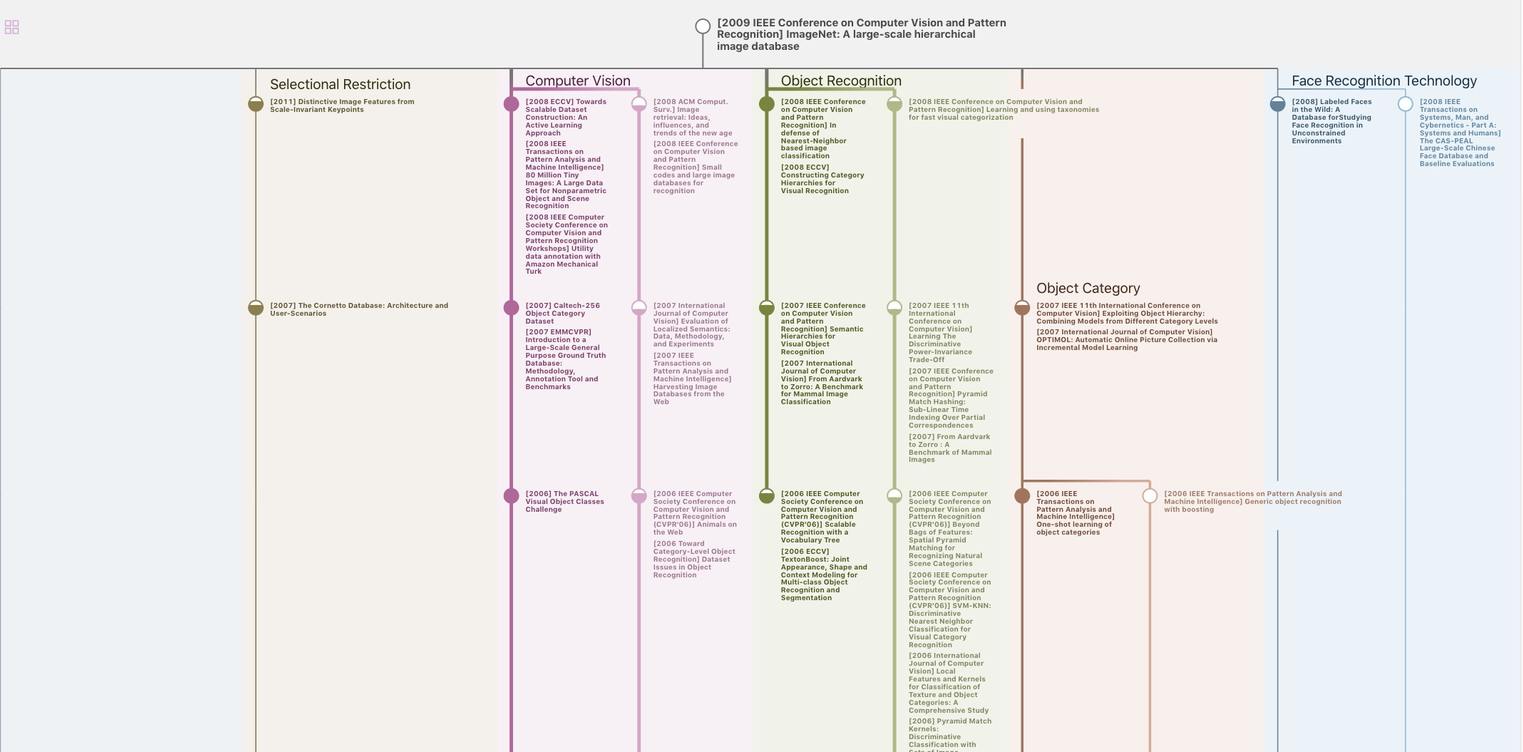
生成溯源树,研究论文发展脉络
Chat Paper
正在生成论文摘要