Nimg-12. spatially mapping treatment-effect in recurrent glioma with physiologic mri and deep learning
Neuro-oncology(2023)
摘要
INTRODUCTION Discriminating between glioma tumor recurrence (rTumor) and treatment-induced effects (TxE) from chemo and radiotherapy using MRI is an ongoing challenge in neuro-oncology. Although deep learning has been used to characterize suspicious lesions, these models typically do not account for lesions that are comprised of mixtures of rTumor and TxE components. We hypothesize that using an approach that includes using tissue samples with known location on MRI, incorporating perfusion-weighted and diffusion-weighted with anatomical MRI, self-supervised pretraining, and a novel ensembling strategy will improve performance and attain spatial maps of rTumor and TxE for determining subsequent treatment. METHODS Using 218 pathology confirmed tissue samples with coordinates on multi-parametric MRI from 111 patients with suspected tumor progression, we developed a deep learning approach to spatially map intratumoral regions of rTumor and TxE. Our model leveraged 10mm isotropic cubes centered on the tissue sample coordinates that includes Diffusion-Weighted and Dynamic Susceptibility-Contrast Perfusion MRI and large amounts of pathologically unlabeled data from the lesion in self-supervised pretraining. The multiple networks of the ensemble were generated through repeated constrained cross validation (RC-CV) which enabled unique repetitions of training with more variation in class proportions. RESULTS Our best classifier performed with a RC-CV/test AUROC of 0.85/0.81 and was able to generate maps of tumor probability as well as ensenble variance and uncertainty. Ensemble variance was significantly higher in prediction failures of rTumor (P=.006) and epistemic uncertainty was significantly higher (P=.002) in prediction failures of TxE in training. Test Performance dropped by 14.7% when removing self-supervised pretraining, 21.3% when removing physiological imaging contrasts, and 1.2% when ensembling across standard 4-fold CV. CONCLUSION The AUROC of .81 supports the feasibility of discriminating between TxE and rTumor within a single lesion non-invasively. Physiologic MRI, pretraining on pathology unlabeled data, and ensembling networks, improve performance and help characterize model failures.
更多查看译文
关键词
recurrent glioma,physiologic mri,treatment-effect
AI 理解论文
溯源树
样例
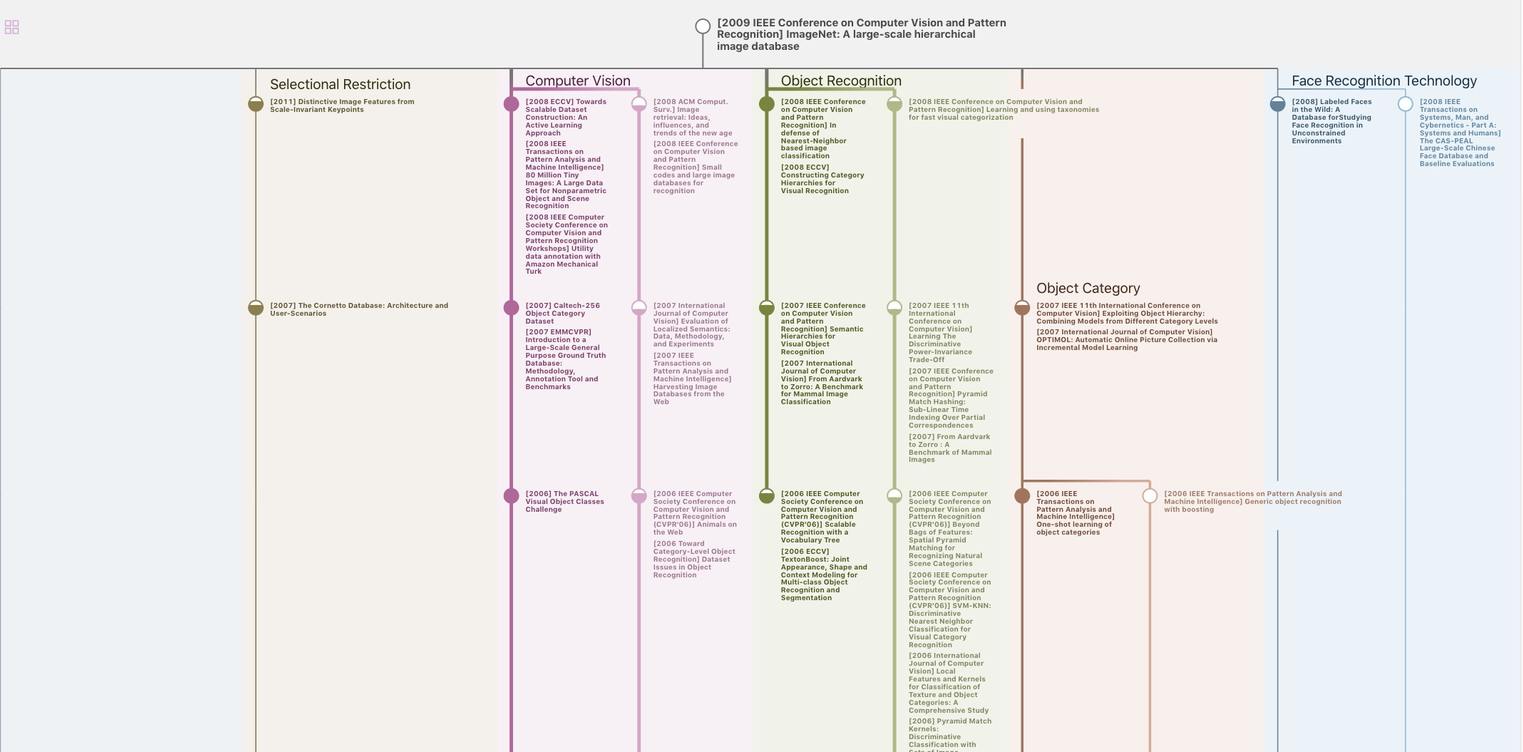
生成溯源树,研究论文发展脉络
Chat Paper
正在生成论文摘要