Mortar-FP8: Morphing the Existing FP32 Infrastructure for High-Performance Deep Learning Acceleration
IEEE TRANSACTIONS ON COMPUTER-AIDED DESIGN OF INTEGRATED CIRCUITS AND SYSTEMS(2024)
摘要
Vanilla deep neural networks (DNNs) after training are represented with native floating-point 32 (fp32) weights. We observe that the bit-level sparsity of these weights is very abundant in the mantissa and the distribution of exponent is aggregated, which can all be directly exploited to speed up model inference. In this article, we propose Mortar and Mortar-FP8, the offline/online software and hardware collaborative approaches for fp32 DNN acceleration. The proposed methods include the software algorithms to morph the mantissa and convert fp32 weights to fp8 format, as well as associated hardware accelerator architecture to accelerate general-purpose deep learning through optimized algorithm and specialized hardware. We highlight the following results by evaluating various deep learning tasks, including image classification, object detection, video understanding, video, and image super-resolution: 1) Mortar increase mantissa sparsity up to 1.58 x -2.09x with only a negligible similar to 0.2% accuracy loss; 2) Mortar-FP8 morph the fp32 weights to fp8 format with a minimal accuracy loss of similar to 0.3%; and 3) the corresponding hardware accelerator significantly outperforms baselines, achieving up to 6.032x and 6.99x performance improvements. The area and power of Mortar are 0.031 mm2 and 68.58 mW. Those metrics are 0.0505 mm2 and 25.16 mW for Mortar-FP8.
更多查看译文
关键词
Deep learning accelerator,deep neural network (DNN),fp8 format
AI 理解论文
溯源树
样例
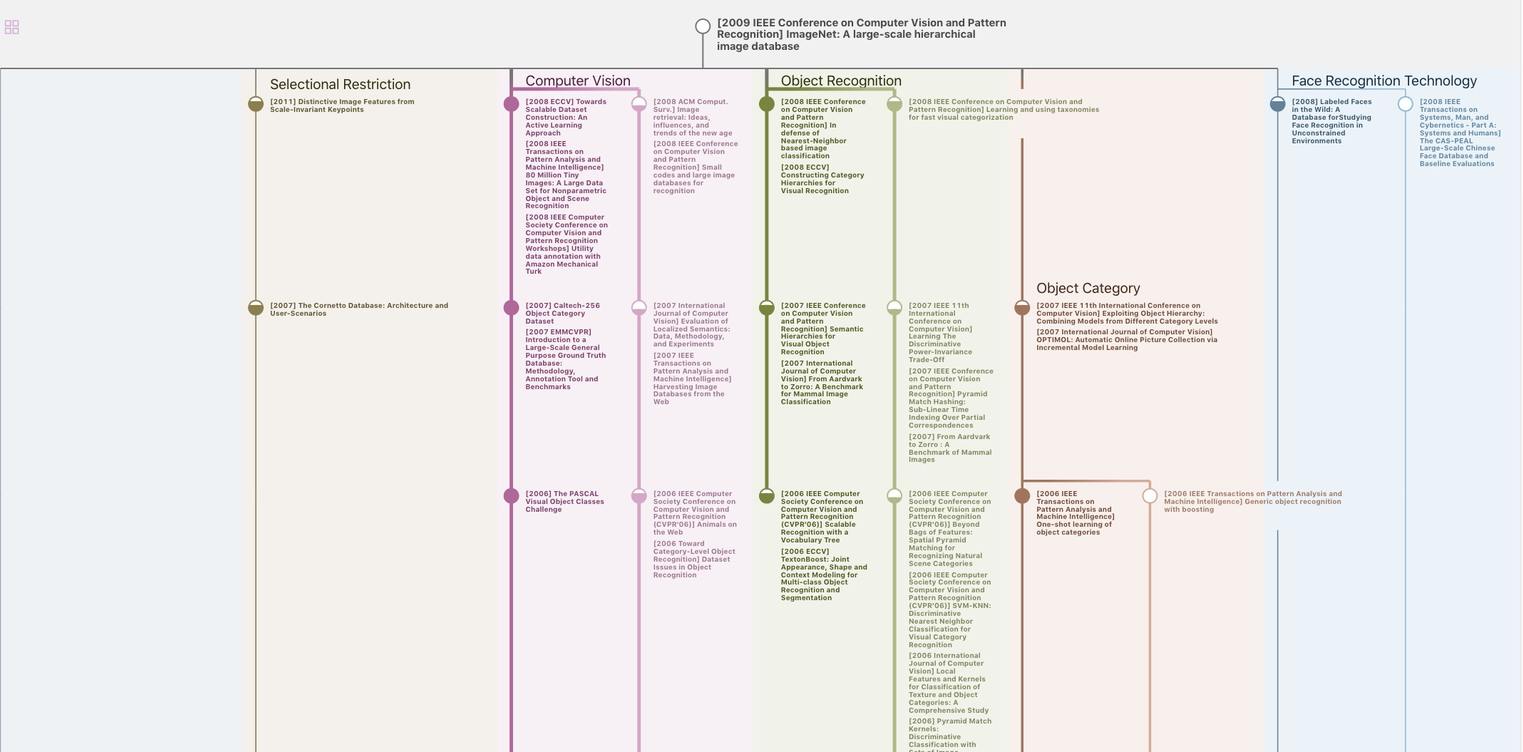
生成溯源树,研究论文发展脉络
Chat Paper
正在生成论文摘要