Practical Methods of Defective Input Feature Correction to Enable Machine Learning in Power Systems
IEEE TRANSACTIONS ON POWER SYSTEMS(2024)
摘要
In this research work, three practical correction methods are proposed to mitigate the impact of defective input features in power system data measurement for machine learning (ML) applications. A well-trained ML tool may become ineffective due to defective input features, which may originate from measurement issues, such as monitor malfunction, cyberattack, communication failure, or others. It is crucial to correct defective input features to enable ML tools with desirable performances. This letter first introduces the mechanism of three correction methods, i.e., statistical-value-based method, minimal-error-based method, and DNN-based adaptive method. Then, the methods are validated via a deep neural network (DNN) case for power system stability enhancement. Validation results demonstrate that the adaptive method achieves the best performance, enabling the well-trained ML tool with a similar accuracy level to the case of no data defects. Although actual measurements may have various data issues challenging ML applications in power systems, the proposed methods show promise for addressing these challenges.
更多查看译文
关键词
Power system stability,Phasor measurement units,Training,Power measurement,Artificial neural networks,Machine learning,Monitoring,Defective data,data correction,data preprocessing,measurement,machine learning,power system
AI 理解论文
溯源树
样例
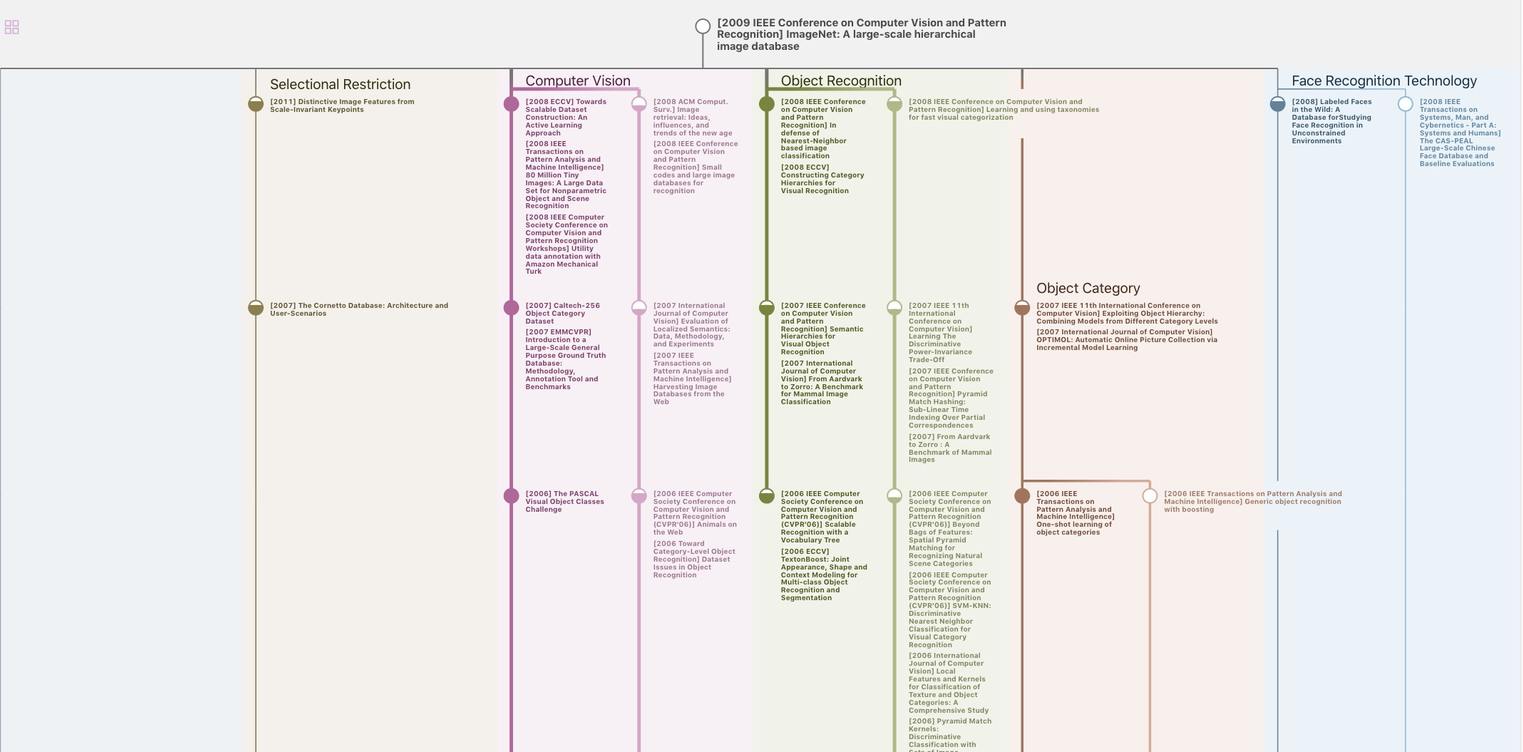
生成溯源树,研究论文发展脉络
Chat Paper
正在生成论文摘要