Cross-network Embeddings Transfer for Traffic Analysis
IEEE Transactions on Network and Service Management(2023)
摘要
Artificial Intelligence (AI) approaches have emerged as powerful tools to improve traffic analysis for network monitoring and management. However, the lack of large labeled datasets and the ever-changing networking scenarios make a fundamental difference compared to other domains where AI is thriving. We believe the ability to transfer the specific knowledge acquired in one network (or dataset) to a different network (or dataset) would be fundamental to speed up the adoption of AI-based solutions for traffic analysis and other networking applications (e.g., cybersecurity). We here propose and evaluate different options to transfer the knowledge built from a provider network, owning data and labels, to a customer network that desires to label its traffic but lacks labels. We formulate this problem as a domain adaptation problem that we solve with embedding alignment techniques and canonical transfer learning approaches. We present a thorough experimental analysis to assess the performance considering both supervised (e.g., classification) and unsupervised (e.g., novelty detection) downstream tasks related to darknet and honeypot traffic. Our experiments show the proper transfer techniques to use the models obtained from a network in a different network. We believe our contribution opens new opportunities and business models where network providers can successfully share their knowledge and AI models with customers.
更多查看译文
关键词
Darknets,network monitoring,transfer learning,representation learning,domain adaptation
AI 理解论文
溯源树
样例
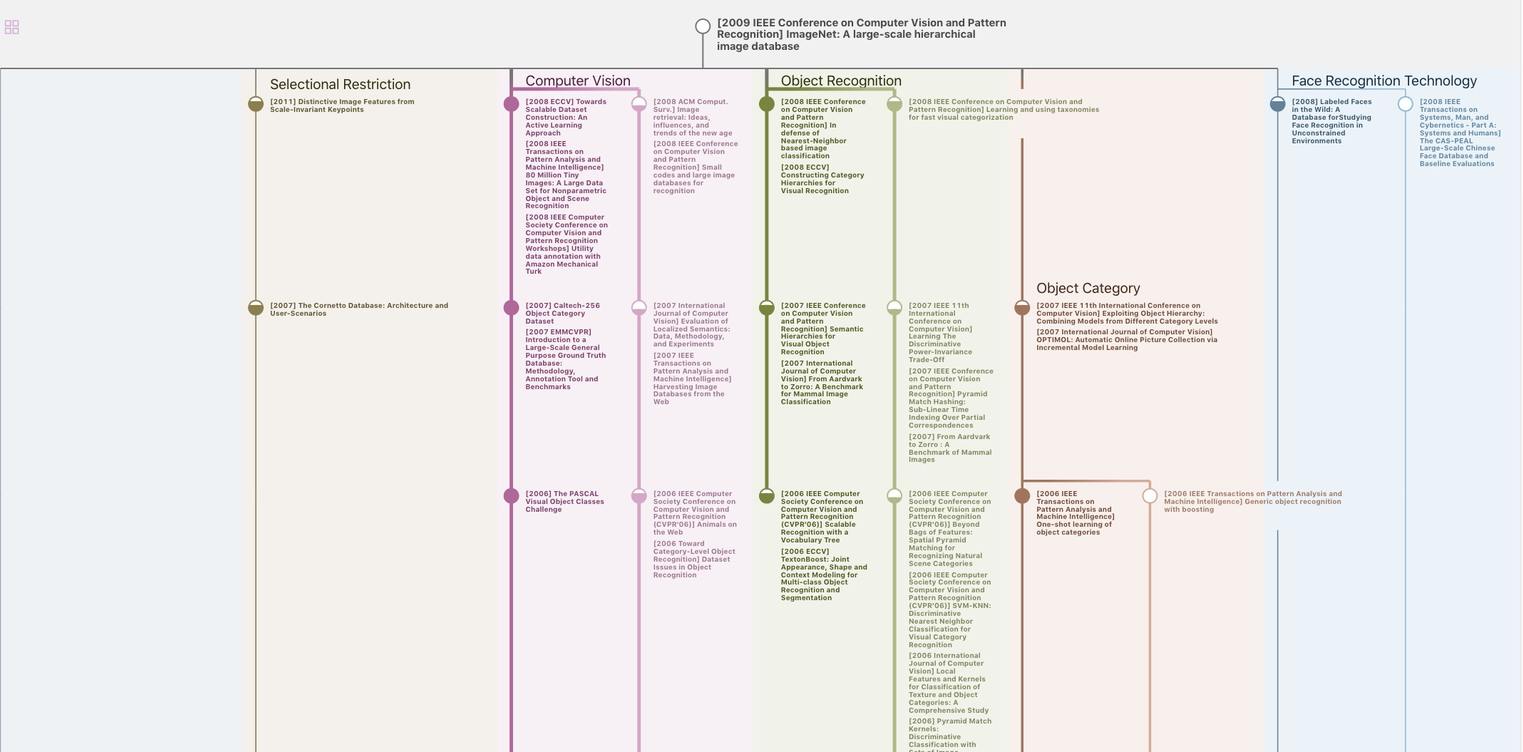
生成溯源树,研究论文发展脉络
Chat Paper
正在生成论文摘要