Beyond tree-shaped credal probabilistic circuits
International Journal of Approximate Reasoning(2023)
摘要
Probabilistic circuits are a class of probabilistic generative models that allow us to compute different types of probabilistic queries in polynomial time. Unlike many of the mainstream approaches for generative modeling, they can compute exact likelihoods, marginals, and expectations. Yet, assessing the reliability of their inferences is not straightforward. Credal probabilistic circuits are the imprecise counterpart of probabilistic circuits allowing, among other queries, computations of cautious inferences and sensitivity analyses. In this work, we propose an efficient algorithm to compute the lower and upper expectations for factorizing functions using a credal probabilistic circuit. We discuss under what structural assumptions and types of factorizing functions the algorithm works. We prove that such algorithm has polynomial time complexity in the input size. In the general case, we prove that computing cautious inferences using credal probabilistic circuits is an NP-hard problem, yet the proposed algorithm can be used as an approximation. Some experiments show how the approximation degrades with the complexity of the model structure.
更多查看译文
关键词
Credal probabilistic circuits,Sum-product networks,Imprecise probabilities,Exact inference,Generative models,Graphical models
AI 理解论文
溯源树
样例
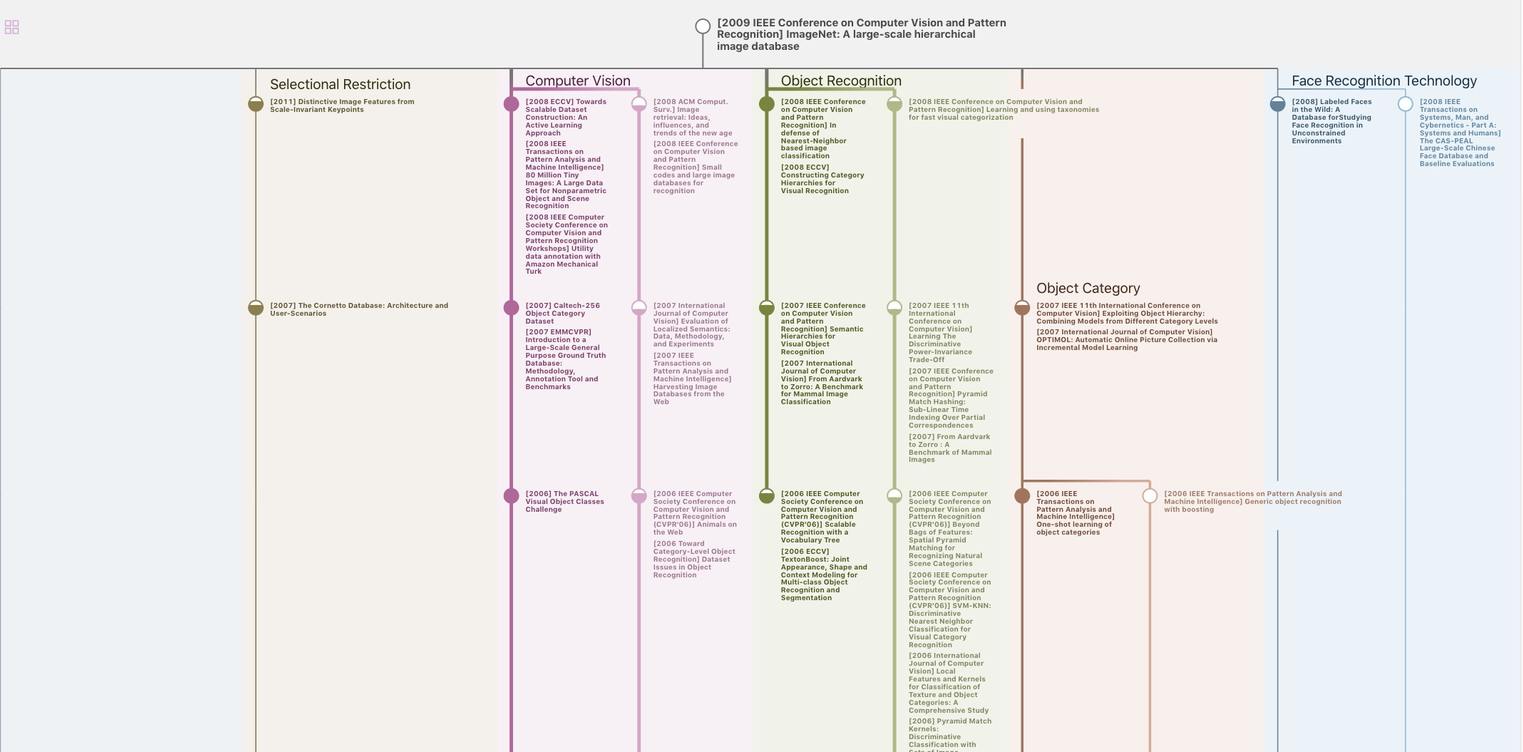
生成溯源树,研究论文发展脉络
Chat Paper
正在生成论文摘要