Adjustable Iterative Q-Learning Schemes for Model-Free Optimal Tracking Control
IEEE TRANSACTIONS ON SYSTEMS MAN CYBERNETICS-SYSTEMS(2024)
摘要
This article puts emphasis on the deterministic value-iteration-based Q-learning (VIQL) algorithm with adjustable convergence speed, followed by the application verification on trajectory tracking for completely unknown nonaffine systems. It is worth emphasizing that, under the effect of learning rates, the convergence speed can be adjusted and the new convergence criterion of the VIQL framework is investigated. The merit of the adjustable VIQL scheme is that it can quicken the learning speed and decrease the number of iterations, thereby reducing the computation burden. To carry out the model-free VIQL algorithm, the offline data of system states and reference trajectories are collected to provide the reference control, the tracking error, and the tracking control, which promotes the parameter updating of the adjustable VIQL algorithm via the off-policy learning scheme. By this updating operation, the convergent optimal tracking policy can guarantee that arbitrary initial state tracks the desired trajectory and can completely obviate the terminal tracking error. Finally, numerical simulations are conducted to indicate the validity of the designed tracking control algorithm.
更多查看译文
关键词
Adaptive critic control,adaptive dynamic programming (ADP),convergence speed,optimal tracking,Q-learning
AI 理解论文
溯源树
样例
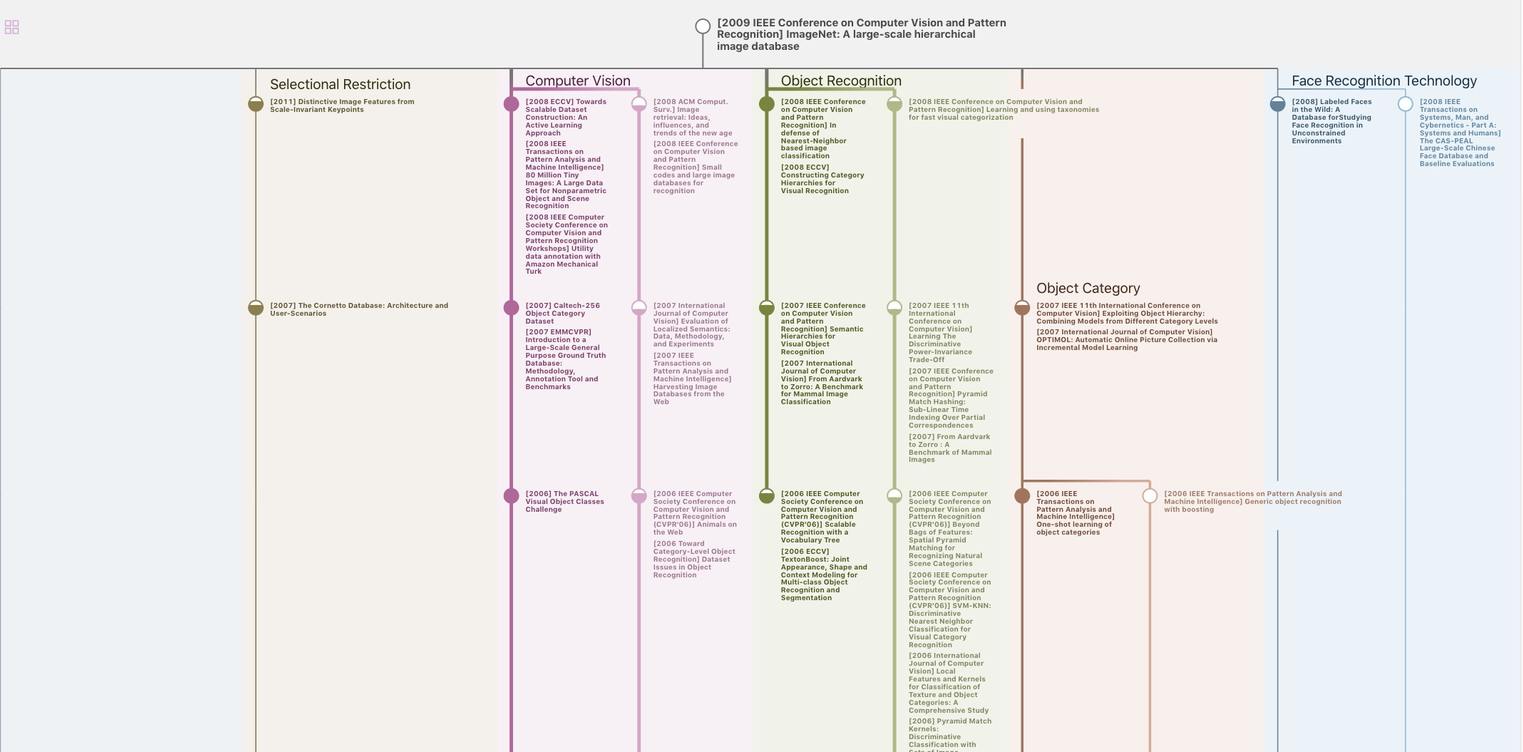
生成溯源树,研究论文发展脉络
Chat Paper
正在生成论文摘要