Analysis of Accelerometer Data Using Random Forest Models to Classify the Behavior of a Wild Nocturnal Primate: Javan Slow Loris (Nycticebus javanicus)
Ecologies(2023)
摘要
Accelerometers are powerful tools for behavioral ecologists studying wild animals, particularly species that are difficult to observe due to their cryptic nature or dense or difficult to access habitats. Using a supervised approach, e.g., by observing in detail with a detailed ethogram the behavior of an individual wearing an accelerometer, to train a machine learning algorithm and the accelerometer data of one individual from a wild population of Javan slow lorises (Nycticebus javanicus), we applied a Random Forest model (RFM) to classify specific behaviors and posture or movement modifiers automatically. We predicted RFM would identify simple behaviors such as resting with the greatest accuracy while more complex behaviors such as feeding and locomotion would be identified with lower accuracy. Indeed, resting behaviors were identified with a mean accuracy of 99.16% while feeding behaviors were identified with a mean accuracy of 94.88% and locomotor behaviors with 85.54%. The model identified a total of 21 distinct combinations of six behaviors and 18 postural or movement modifiers in this dataset showing that RFMs are effective as a supervised approach to classifying accelerometer data. The methods used in this study can serve as guidelines for future research for slow lorises and other ecologically similar wild mammals. These results are encouraging and have important implications for understanding wildlife responses and resistance to global climate change, anthropogenic environmental modification and destruction, and other pressures.
更多查看译文
关键词
animal behavior,supervised machine learning,random forest model
AI 理解论文
溯源树
样例
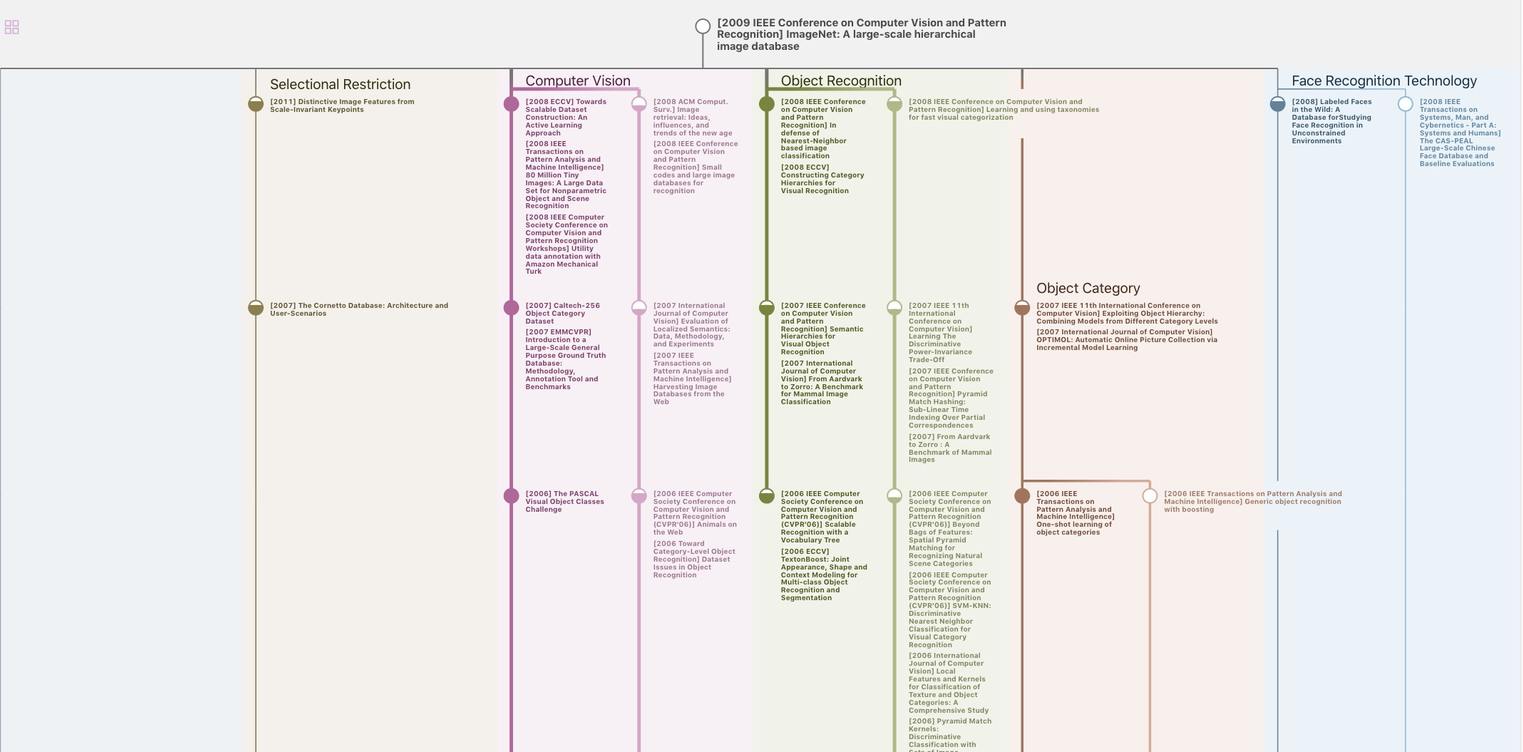
生成溯源树,研究论文发展脉络
Chat Paper
正在生成论文摘要