Robust Procedural Learning for Anomaly Detection and Observability in 5G RAN
IEEE Transactions on Network and Service Management(2023)
摘要
Most existing large distributed systems have poor observability and cannot use the full potential of machine learning-based behavior analysis. The system logs, which contain the primary source of information, are unstructured and lack the context needed to track procedures and learn the system’s behavior. This work presents a new trace guideline that enables a component-and procedure-based split of the system logs for the future 5G Radio Access Network (RAN). As the system can be broken into smaller pieces, models can more accurately learn the system’s behavior and use the context to improve anomaly detection and observability. The evaluation result is astonishing; where previously state-of-the-art methods struggle to learn the behavior, a fast, dictionary-based algorithm can detect all anomalies and keep false positives close to zero. Troubleshooters can also more quickly identify anomalies and gain useful insights into the component interaction in RAN.
更多查看译文
关键词
observability,trace guidelines,anomaly detection,Radio Access Network,5G
AI 理解论文
溯源树
样例
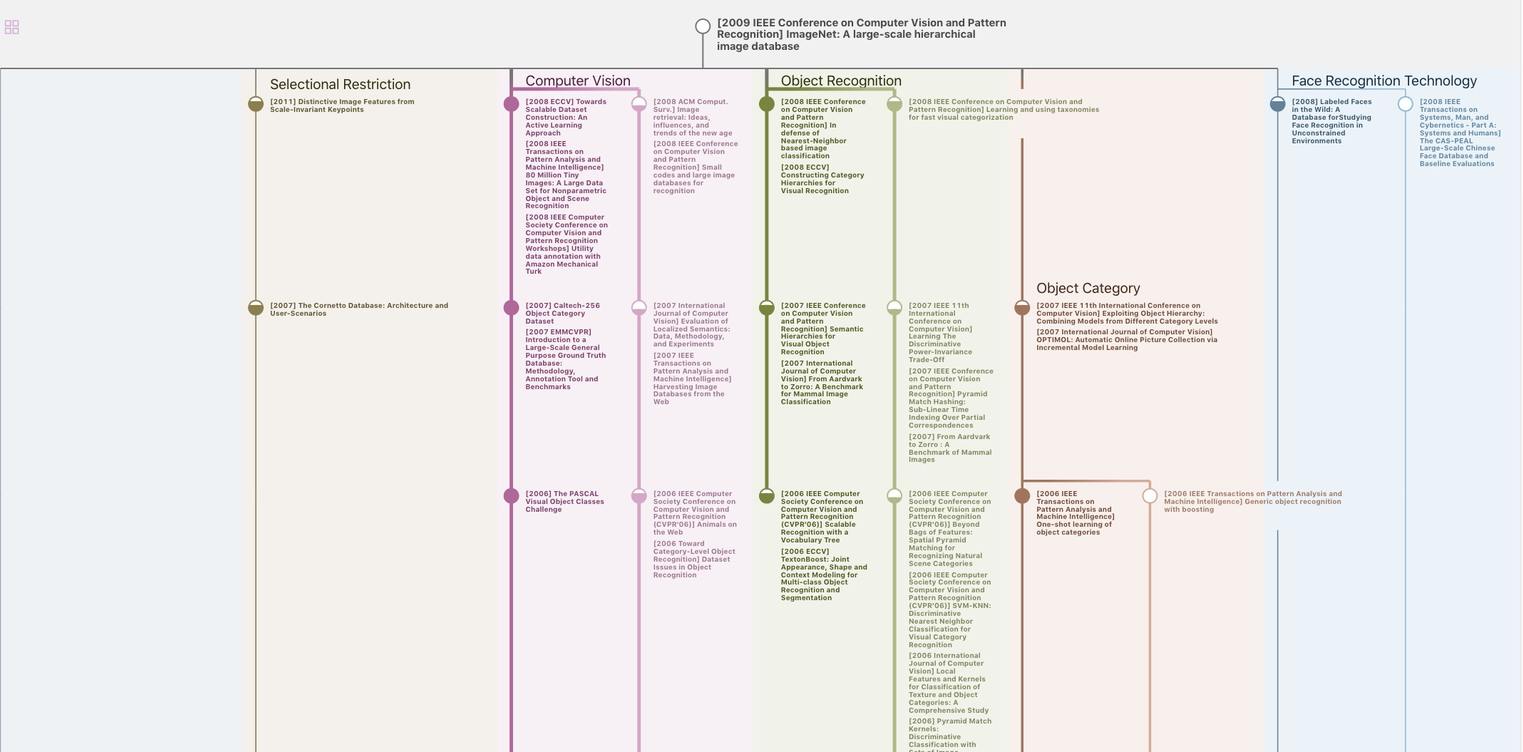
生成溯源树,研究论文发展脉络
Chat Paper
正在生成论文摘要