MonoSKD: General Distillation Framework for Monocular 3D Object Detection via Spearman Correlation Coefficient
Frontiers in artificial intelligence and applications(2023)
摘要
Monocular 3D object detection is an inherently ill-posed problem, as it is challenging to predict accurate 3D localization from a single image. Existing monocular 3D detection knowledge distillation methods usually project the LiDAR onto the image plane and train the teacher network accordingly. Transferring LiDAR-based model knowledge to RGB-based models is more complex, so a general distillation strategy is needed. To alleviate cross-modal problem, we propose MonoSKD, a novel Knowledge Distillation framework for Monocular 3D detection based on Spearman correlation coefficient, to learn the relative correlation between cross-modal features. Considering the large gap between these features, strict alignment of features may mislead the training, so we propose a looser Spearman loss. Furthermore, by selecting appropriate distillation locations and removing redundant modules, our scheme saves more GPU resources and trains faster than existing methods. Extensive experiments are performed to verify the effectiveness of our framework on the challenging KITTI 3D object detection benchmark. Our method achieves state-of-the-art performance until submission with no additional inference computational cost. Our codes are available at https://github.com/Senwang98/MonoSKD.
更多查看译文
关键词
monocular 3d object detection,correlation
AI 理解论文
溯源树
样例
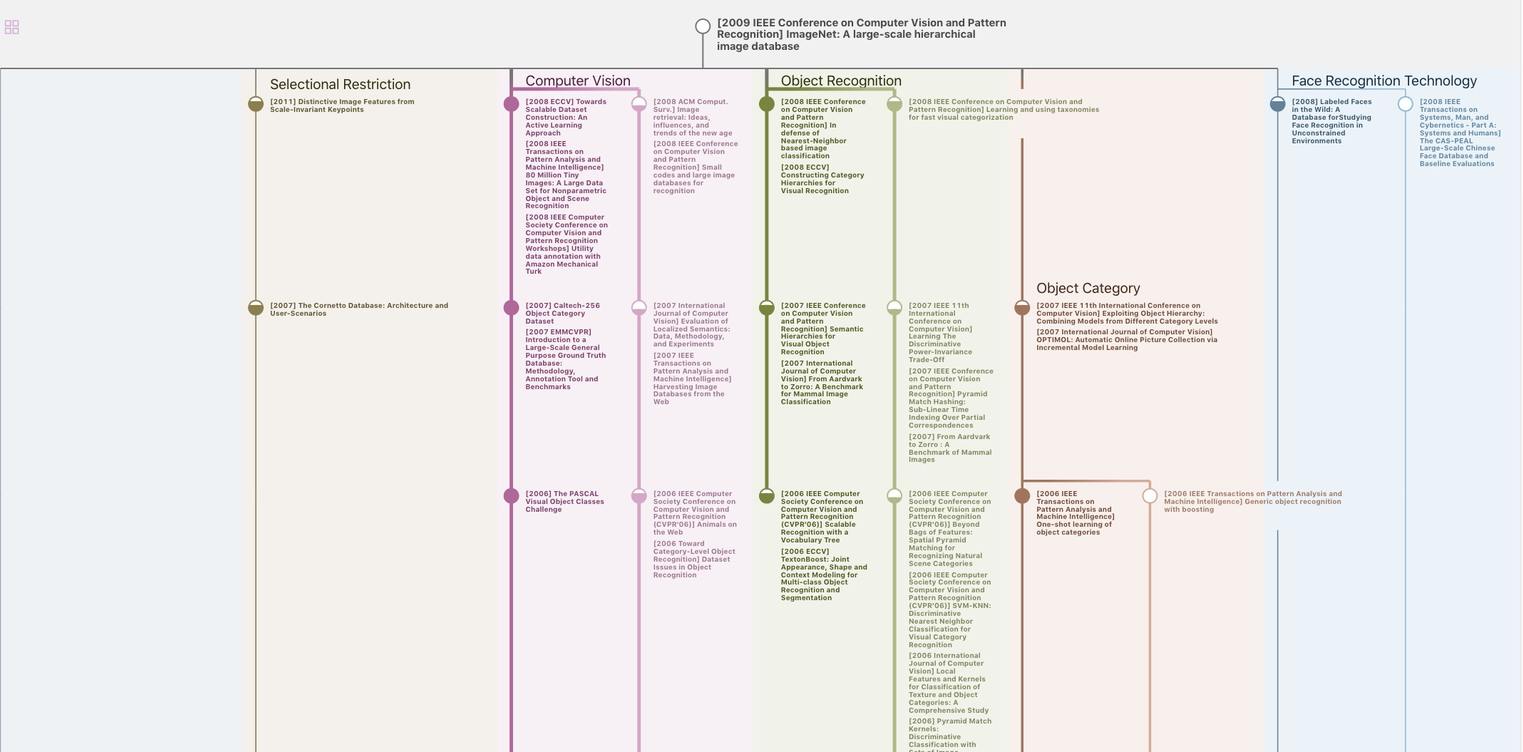
生成溯源树,研究论文发展脉络
Chat Paper
正在生成论文摘要