GAF-MAE: A Self-Supervised Automatic Modulation Classification Method Based on Gramian Angular Field and Masked Autoencoder
IEEE TRANSACTIONS ON COGNITIVE COMMUNICATIONS AND NETWORKING(2024)
摘要
With the development of deep learning (DL), several fields have ushered in leapfrog development, such as image classification and natural language processing. Combing the powerful feature extraction tool, DL-based Automatic Modulation Classification (AMC) emerges. However, most DL-based AMC methods require massive labeled samples for training, which is difficult for non-cooperative scenarios. In this paper, we propose a novel self-supervised AMC method called GAF-MAE. Gramian Angular Field (GAF) is applied for domain transformation from time series to images first, then a Masked Autoencoder (MAE) is built for self-supervised reconstruction tasks through unlabeled samples. After self-supervised pretrain, a small number of labeled samples are used for downstream classification finetuning. We conduct a comprehensive evaluation of a public dataset RadioML.2016.10a. The simulation results show that GAF-MAE can achieve a relatively high average accuracy of 54.85% even if the label proportion is 5% and 61.38% when the label proportion reaches 100%, which outperforms other well-known models.
更多查看译文
关键词
Automatic modulation classification,masked autoencoder,self-supervised
AI 理解论文
溯源树
样例
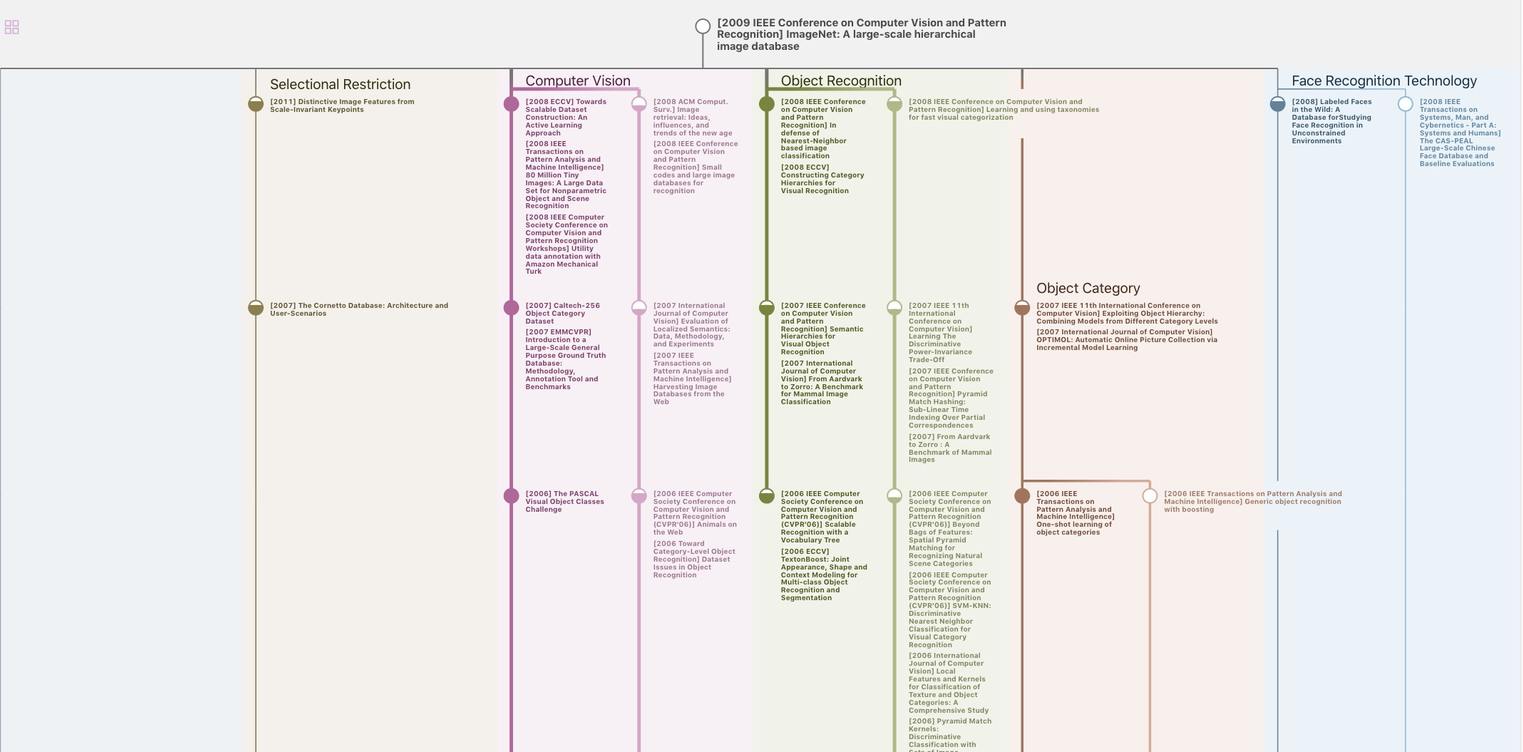
生成溯源树,研究论文发展脉络
Chat Paper
正在生成论文摘要