Improving Dialogue Summarization with Mixup Label Smoothing
Lecture notes in electrical engineering(2023)
摘要
The abstractive dialogue summarization models trained with Maximum Likelihood Estimation suffer from the overconfident issue because the training objective encourages the model to assign all probability to the hard target. Although Label Smoothing is widely adopted to prevent the models from being overconfident, it assumes a pre-defined uniform distribution that is not adaptive and is not an ideal soft target. Therefore, we propose a Mixup Label Smoothing method in this paper, which exploits the general knowledge from the language model to construct a flexible soft target to present diverse candidates. We conceptualize the hypothesis distribution obtained from a pretrained language model as the context-smoothing target, which encodes much knowledge through the massive pretraining corpus and implies more possible candidate summaries. Extensive experiments on three popular dialogue summarization datasets demonstrate that our method effectively outperforms various strong baselines, as well as in low-resource settings.
更多查看译文
关键词
dialogue summarization
AI 理解论文
溯源树
样例
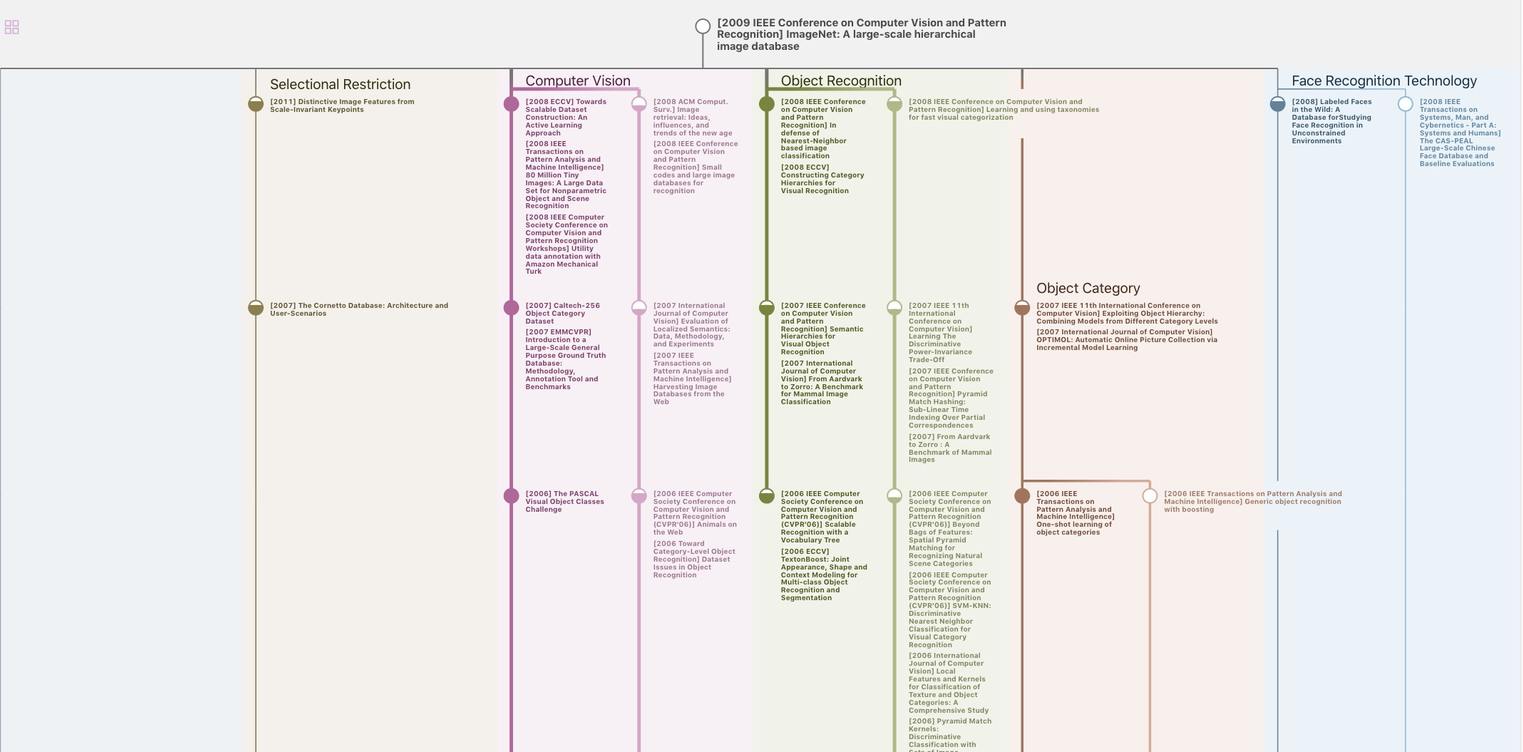
生成溯源树,研究论文发展脉络
Chat Paper
正在生成论文摘要