Lightweight LAE for Anomaly Detection With Sound-Based Architecture in Smart Poultry Farm.
IEEE Internet Things J.(2024)
摘要
In poultry farms, the internal environment and movement of birds directly impacts health of birds. Timely analysis of internal environment data is important as it may lead to an unhealthy environment for birds. Traditionally, data analysis techniques were performed on the data collected from the IoT devices in the cloud. However, cloud-based solutions are constrained by the lower data bandwidth available in the poultry farms situated in rural areas. Also, IoT devices have limited computational capabilities. The increase in processing capabilities of the IoT device facilitates the data analysis on the device itself termed as edge computing. Hence, an Edge-IoT-based model has been proposed to monitor and detect anomalies of the internal environment of the farm. Raspberry Pi 4 is used as an edge device in place of the high-cost edge GPUs (Graphics Processing Units). A light-weight deep learning (DL) algorithm, Long Short-Term Memory-based Autoencoder has been used for inferences on the multivariate dataset acquired from various installed sensors. The proposed model has outperformed several existing methods by achieving an F1 score and Recall of 0.9627 and 0.959, respectively, at edge platforms. The performance of light-weight DL model on edge devices is same as that of original model with inference time of 2ms per event. This leads to inclusion of Raspberry Pi 4 at edge nodes which can be a new opportunity for low-cost solutions. Furthermore, a novel sound-based architecture is proposed to increase the movement of birds inside the farm that directly improves the health of birds.
更多查看译文
关键词
LSTM,Autoencoder,Deep learning,Edge computing,Internet of Things,Smart Poultry Farming
AI 理解论文
溯源树
样例
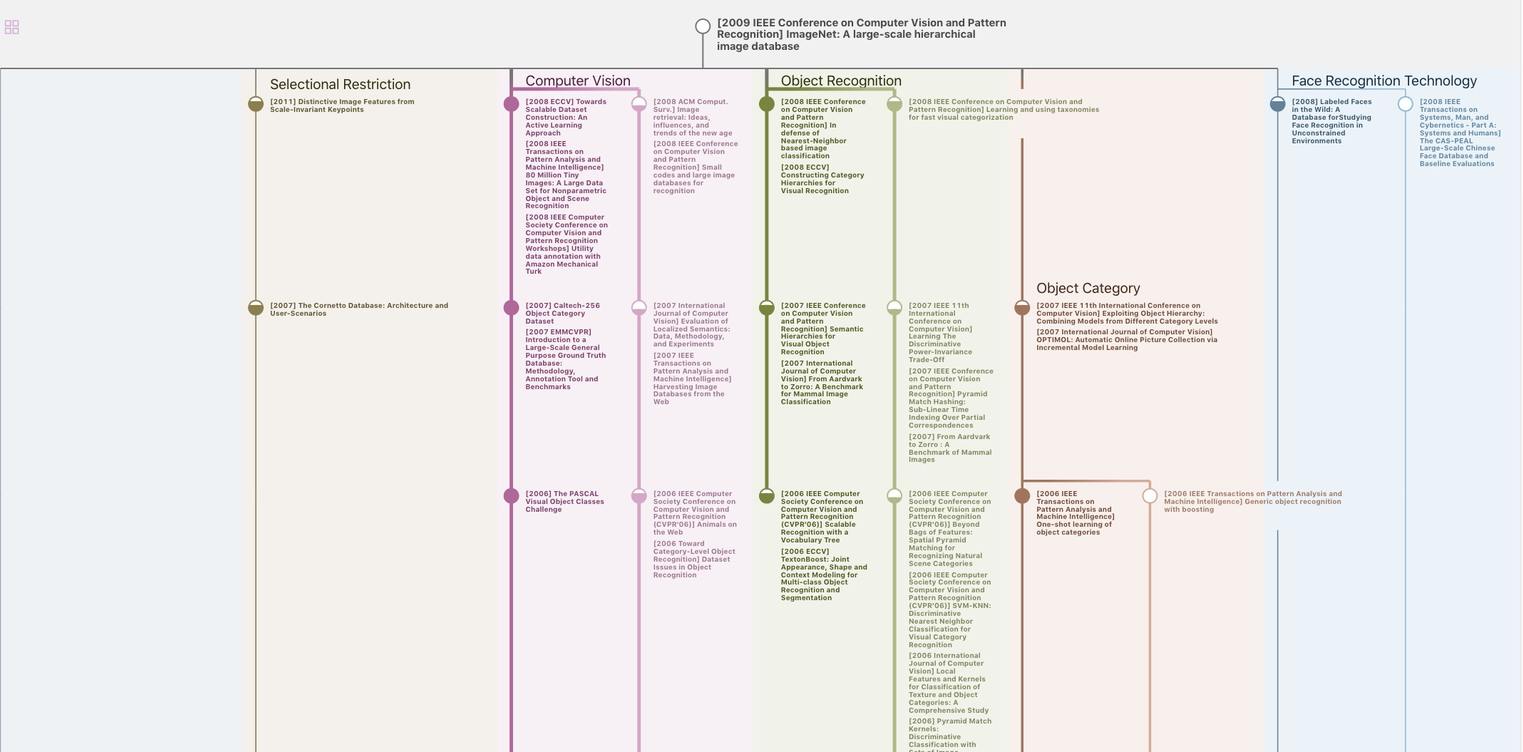
生成溯源树,研究论文发展脉络
Chat Paper
正在生成论文摘要