Concept and dependencies enhanced graph convolutional networks for short text classification
JOURNAL OF INTELLIGENT & FUZZY SYSTEMS(2023)
摘要
Short text classification task is a special kind of text classification task in that the text to be classified is generally short, typically generating a sparse text representation that lacks rich semantic information. Given this shortcoming, scholars worldwide have explored improved short text classification methods based on deep learning. However, existing methods cannot effectively use concept knowledge and long-distance word dependencies. Therefore, based on graph neural networks from the perspective of text composition, we propose concept and dependencies enhanced graph convolutional networks for short text classification. First, the co-occurrence relationship between words is obtained by sliding window, the inclusion relationship between documents and words is obtained by TF-IDF, long-distance word dependencies is obtained by Stanford CoreNLP, and the association relationship between concepts in the concept graph with entities in the text is obtained through Microsoft Concept Graph. Then, a text graph is constructed for an entire text corpus based on the four relationships. Finally, the text graph is input into graph convolutional neural networks, and the category of each document node is predicted after two layers of convolution. Experimental results demonstrate that our proposed method overall best on multiple classical English text classification datasets.
更多查看译文
关键词
Short text classification,Knowledge graph,Graph convolutional neural networks,Long-distance dependency,Building graph for text
AI 理解论文
溯源树
样例
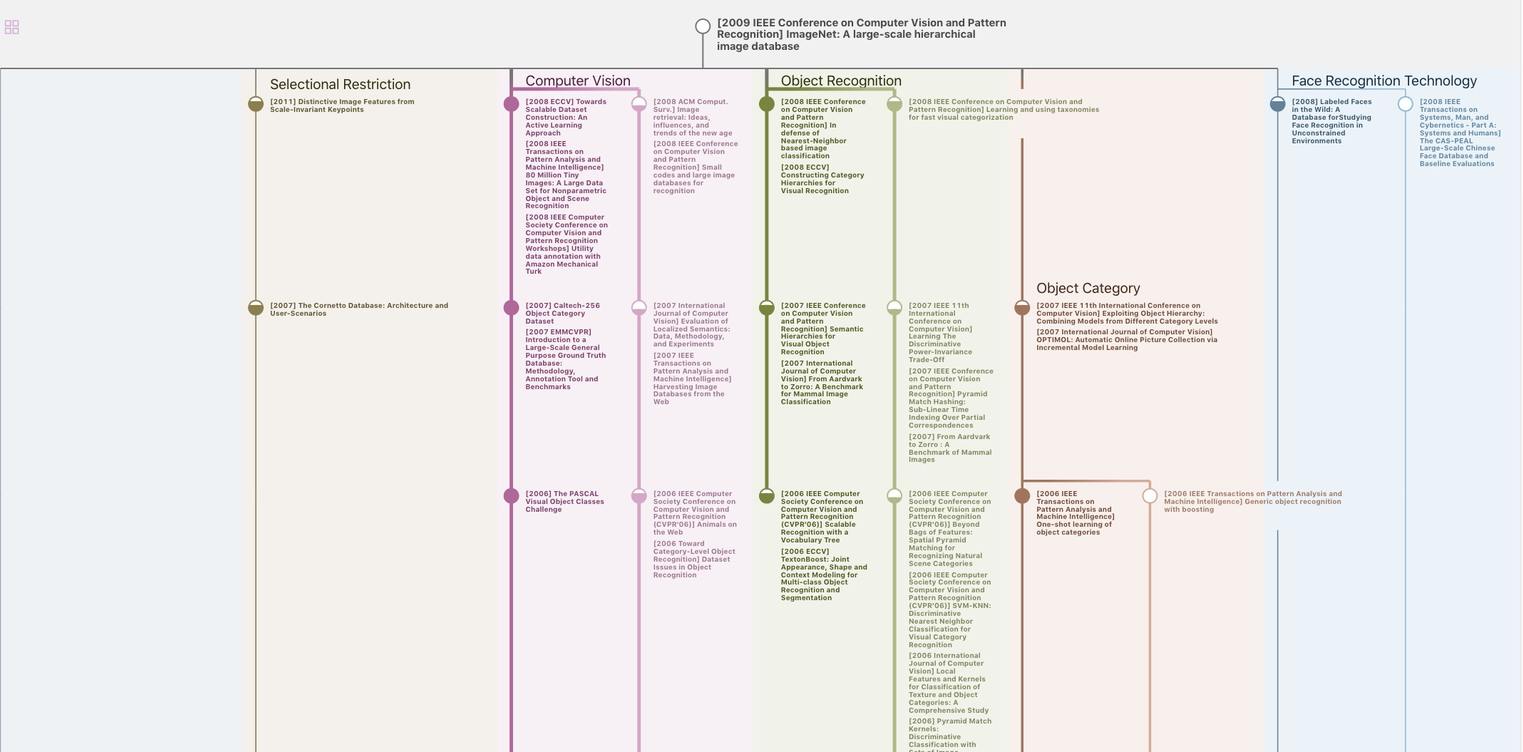
生成溯源树,研究论文发展脉络
Chat Paper
正在生成论文摘要