MPCT: Multiscale Point Cloud Transformer With a Residual Network.
IEEE Trans. Multim.(2024)
摘要
The self-attention (SA) network revisits the essence of data and has achieved remarkable results in text processing and image analysis. SA is conceptualized as a set operator that is insensitive to the order and number of data, making it suitable for point sets embedded in 3D space. However, working with point clouds still poses challenges. To tackle the issue of exponential growth in complexity and singularity induced by the original SA network without position encoding, we modify the attention mechanism by incorporating position encoding to make it linear, thus reducing its computational cost and memory usage and making it more feasible for point clouds. This paper presents a new framework called multiscale point cloud transformer (MPCT), which improves upon prior methods in cross-domain applications. The utilization of multiple embeddings enables the complete capture of the remote and local contextual connections within point clouds, as determined by our proposed attention mechanism. Additionally, we use a residual network to facilitate the fusion of multiscale features, allowing MPCT to better comprehend the representations of point clouds at each stage of attention. Experiments conducted on several datasets demonstrate that MPCT outperforms the existing methods, such as achieving accuracies of 94.2% and 84.9% in classification tasks implemented on ModelNet40 and ScanObjectNN, respectively.
更多查看译文
关键词
Point cloud transformer,multiscale generation,residual network,geometric and semantic features
AI 理解论文
溯源树
样例
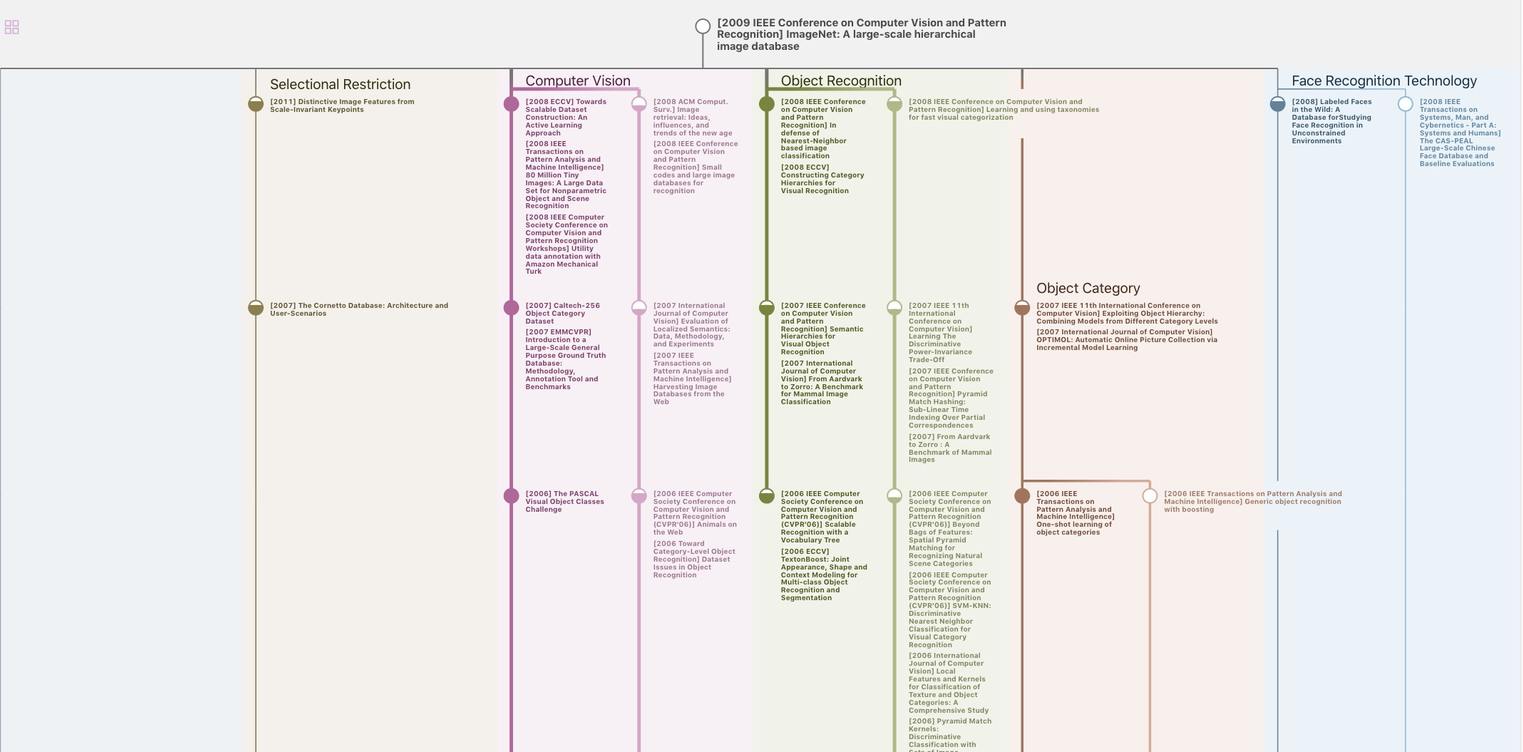
生成溯源树,研究论文发展脉络
Chat Paper
正在生成论文摘要