Prediction and optimization of 3D-printed sandwich beams with chiral cores
INTERNATIONAL JOURNAL OF MECHANICAL SCIENCES(2024)
摘要
This study pursues two primary objectives concerning sandwich structures with chiral cores. Firstly, it delves into the realm of machine learning-assisted predictions regarding the behavior of these structures under compressive loads. In this way, three key geometric parameters of the repetitive chiral unit cells-thickness, angle, and diameter-are considered as highly influential design factors. Accordingly, a custom method for designing ex-periments was employed, resulting in achieving 27 totally different sandwich beams. These beam structures were made up of Poly Lactic Acid (PLA) for both their face sheets and cores, employing Fused Deposition Modeling (FDM) printing technology. Subsequently, they underwent compressive loading, where the resulting mechanical responses forming the foundational dataset for training Deep Neural Networks (DNNs). Remarkably, the DNNs were trained utilizing both Bayesian and conjugate gradient algorithms. The outcomes notably demonstrated that DNNs trained with the Bayesian algorithm exhibited superior accuracy in predicting stress-strain responses of the beams. Transitioning to the study's second objective, Response Surface Methodology (RSM) was harnessed to optimize Young's modulus and Specific Energy Absorption (SEA). The application of RSM impressively showcased its robustness in attaining optimal design values for these mechanical properties over the defined range of design parameters. This comprehensive exploration effectively reveals the potential of machine learning predictions and optimization techniques, providing valuable insights into the intricate mechanical behaviors of sandwich structures featuring innovative cores.
更多查看译文
关键词
Sandwich beams,Chiral unit cell,3D printing,Compressive test,Deep neural networks,Response surface methodology
AI 理解论文
溯源树
样例
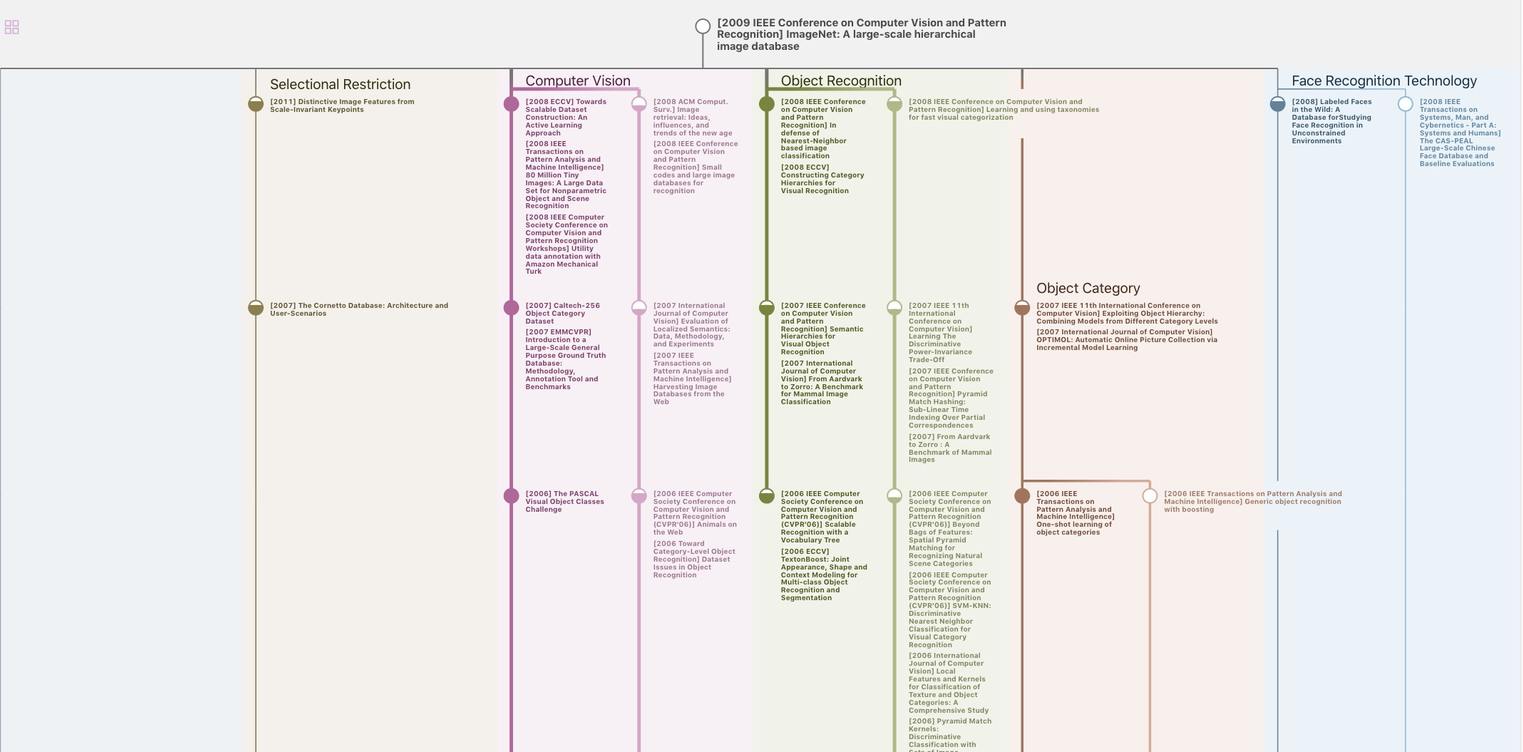
生成溯源树,研究论文发展脉络
Chat Paper
正在生成论文摘要