Learning Bottleneck Transformer for Event Image-Voxel Feature Fusion based Classification
arXiv (Cornell University)(2023)
摘要
Recognizing target objects using an event-based camera draws more and more attention in recent years. Existing works usually represent the event streams into point-cloud, voxel, image, etc, and learn the feature representations using various deep neural networks. Their final results may be limited by the following factors: monotonous modal expressions and the design of the network structure. To address the aforementioned challenges, this paper proposes a novel dual-stream framework for event representation, extraction, and fusion. This framework simultaneously models two common representations: event images and event voxels. By utilizing Transformer and Structured Graph Neural Network (GNN) architectures, spatial information and three-dimensional stereo information can be learned separately. Additionally, a bottleneck Transformer is introduced to facilitate the fusion of the dual-stream information. Extensive experiments demonstrate that our proposed framework achieves state-of-the-art performance on two widely used event-based classification datasets. The source code of this work is available at: \url{https://github.com/Event-AHU/EFV_event_classification}
更多查看译文
关键词
bottleneck transformer,fusion
AI 理解论文
溯源树
样例
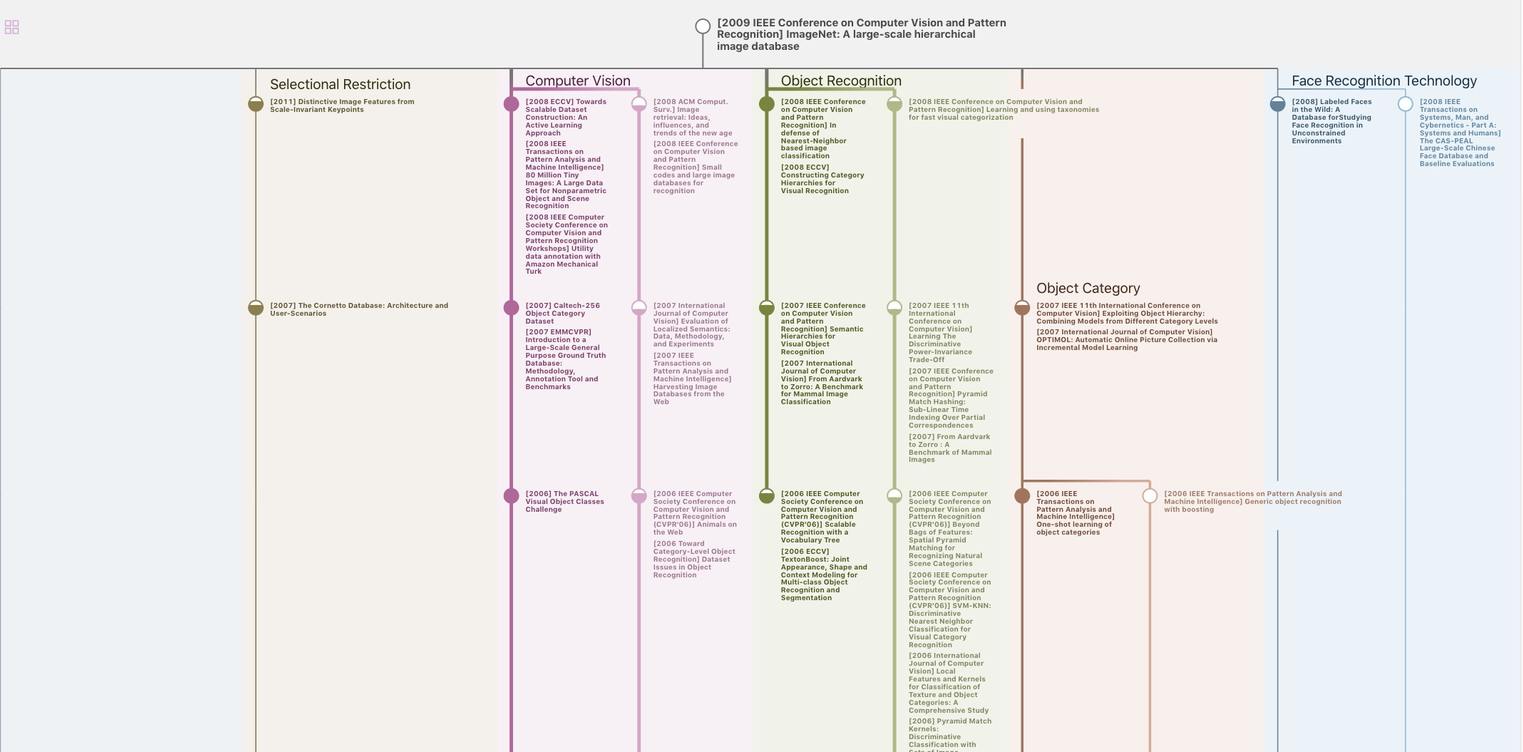
生成溯源树,研究论文发展脉络
Chat Paper
正在生成论文摘要