P1246: cell type identification using multiplex immunofluorescence (mif) guided machine learning in dlbcl
HemaSphere(2023)
摘要
Topic: 20. Lymphoma Biology & Translational Research Background: Diffuse large B-cell lymphoma (DLBCL), the most common form of Non-Hodgkin’s Lymphoma, is very aggressive and heterogeneous. Up to 40% of patients develop relapsed/refractory disease after the standard treatment of rituximab, cyclophosphamide, doxorubicin, vincristine, and prednisone (R-CHOP). Importantly, levels of lymphocytes and macrophages correlate with response to R-CHOP, so the ability to accurately detect and quantify these cells is critical. However, manual cell type classification on hematoxylin and eosin (H&E)-stained whole slide images (WSIs) is challenging. To this end, deep learning-based computational pathology has been proposed to characterize the tumor microenvironment for DLBCL patients and predict progression risk. Aims: Accurate quantification of the cell types in DLBCL specimens may enable prediction of relapse risk or response to therapy and reveal potential prognostic and predictive biomarkers. We sought to develop precise cell detection models based on multiplex immunofluorescence (mIF)-guided annotations in DLBCL. Methods: DLBCL samples (N=166 cases and 331 slides; commercially sourced) were serially sectioned to generate two distinctly stained datasets. One was stained only with H&E; the other was stained using mIF against CD3, CD335, CD8, FOXP3, CD68, and PAX5 followed by terminal, post-mIF H&E staining. Cellular annotations were collected by expert hematopathologists on the conventional H&E and were guided using aligned mIF images for terminal H&E. The cases were split into training (104; 62.7%), validation (31; 18.7%) and test (31; 18.7%) sets. A deep convolutional neural network was trained on each set of annotations for the detection and classification of cell types (e.g., lymphocytes, macrophages, and lymphoma cells) on the H&E WSIs. For the validation set, 240 image frames from the terminal H&E-stained WSIs were exhaustively annotated by 5 hematopathologists, first without and then with the guidance of the mIF images, to obtain the H&E and mIF-guided annotator consensus for each cell location, respectively. For the test set, mIF-guided annotator consensus was obtained on 235 image frames from the terminal H&E-stained WSIs. Consensus was reached when ≥3 hematopathologists agreed on a given cell type and location. Results: We assessed the impact of mIF guidance on collected annotations and annotator and model performance using frames from H&E- and terminal H&E-stained WSIs. Annotator agreement with consensus for the identification of lymphocytes and macrophages was higher when annotating with mIF guidance than without (ΔF1 = +18.5% and +12.6%, respectively). Notably, when comparing the H&E and mIF-guided annotator consensus labels, more cells were identified as lymphocytes and macrophages with mIF guidance, while fewer were identified as lymphoma cells; thus, cells originally labeled as lymphoma cells were relabeled as lymphocytes or macrophages with mIF guidance (Fig. 1). In addition, the model trained using mIF-guided annotations improved the detection of lymphocytes and macrophages compared to mIF-guided annotator consensus (ΔF1 = +21.1% and +5.5%, respectively). Summary/Conclusion: mIF guidance enhances the ability of hematopathologists to properly identify lymphoma cells, lymphocytes, and macrophages in DLBCL clinical specimens, leading to fewer cell type misclassifications and better model performance. Given the association of immune cells with response to therapy in DLBCL, these models have the potential to impact biomarker discovery and clinical decision making. Figure 1. Cell identification using H&E vs. mIF guidance in DLBCL. The number of cells per consensus label on terminal H&E-stained validation frames for annotators with and without mIF guidance are shown.Keywords: Diffuse large B cell lymphoma, Machine learning, Pathology
更多查看译文
关键词
multiplex immunofluorescence,cell,guided machine learning,machine learning
AI 理解论文
溯源树
样例
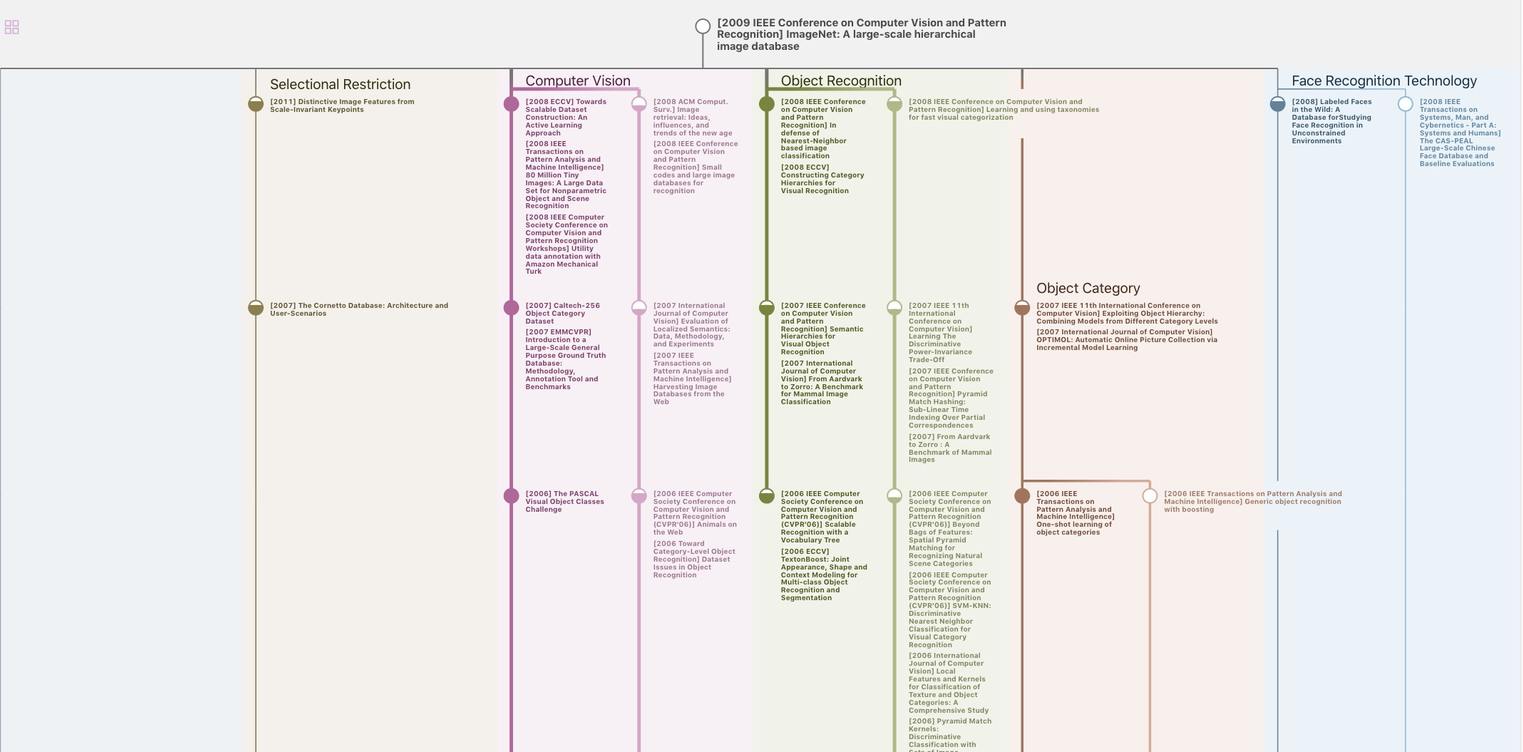
生成溯源树,研究论文发展脉络
Chat Paper
正在生成论文摘要