Dual Meta-Learning with Longitudinally Generalized Regularization for One-Shot Brain Tissue Segmentation Across the Human Lifespan
arXiv (Cornell University)(2023)
摘要
Brain tissue segmentation is essential for neuroscience and clinical studies. However, segmentation on longitudinal data is challenging due to dynamic brain changes across the lifespan. Previous researches mainly focus on self-supervision with regularizations and will lose longitudinal generalization when fine-tuning on a specific age group. In this paper, we propose a dual meta-learning paradigm to learn longitudinally consistent representations and persist when fine-tuning. Specifically, we learn a plug-and-play feature extractor to extract longitudinal-consistent anatomical representations by meta-feature learning and a well-initialized task head for fine-tuning by meta-initialization learning. Besides, two class-aware regularizations are proposed to encourage longitudinal consistency. Experimental results on the iSeg2019 and ADNI datasets demonstrate the effectiveness of our method. Our code is available at https://github.com/ladderlab-xjtu/DuMeta.
更多查看译文
关键词
longitudinally generalized regularization,dual,meta-learning,one-shot
AI 理解论文
溯源树
样例
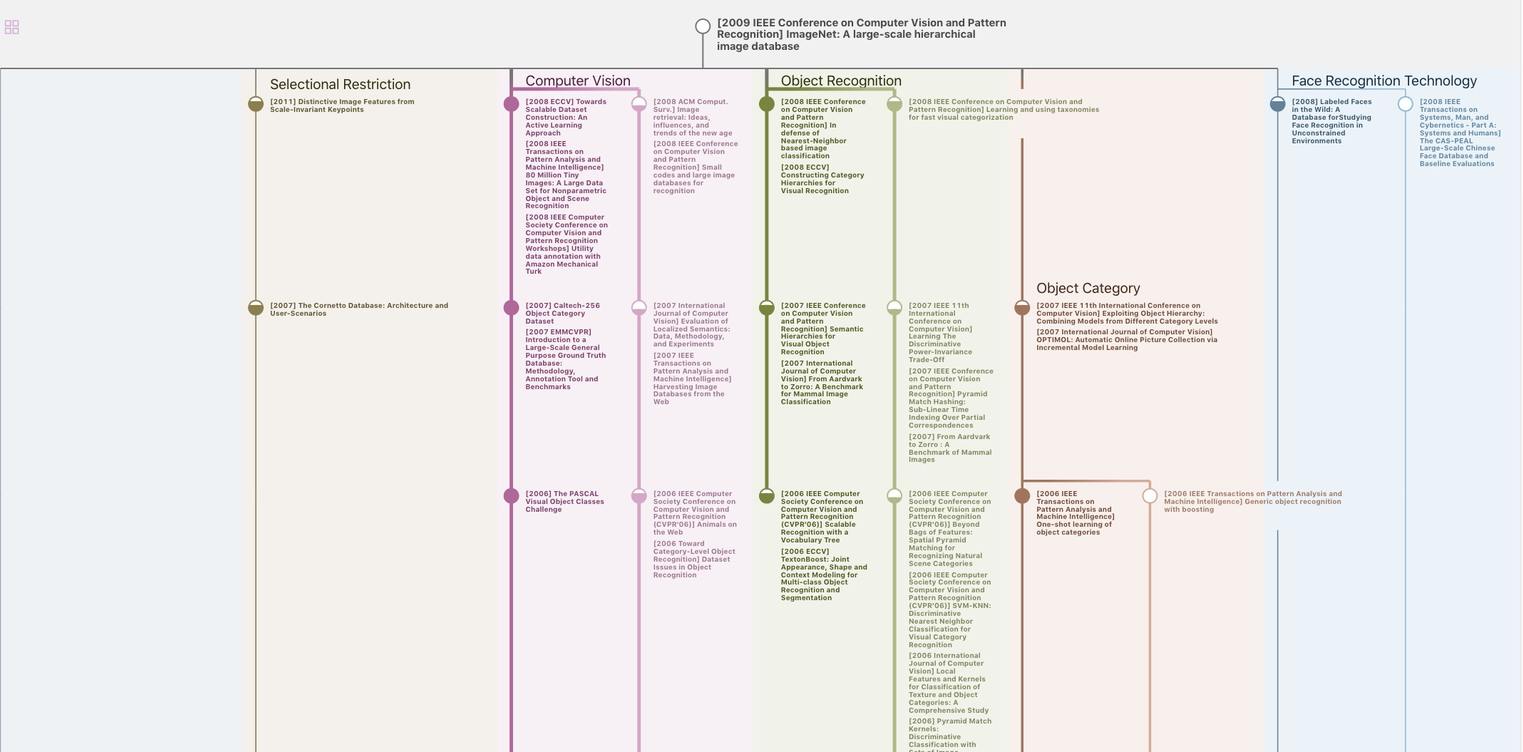
生成溯源树,研究论文发展脉络
Chat Paper
正在生成论文摘要