White-box Membership Inference Attacks against Diffusion Models
arXiv (Cornell University)(2023)
摘要
Diffusion models have begun to overshadow GANs and other generative models in industrial applications due to their superior image generation performance. The complex architecture of these models furnishes an extensive array of attack features. In light of this, we aim to design membership inference attacks (MIAs) catered to diffusion models. We first conduct an exhaustive analysis of existing MIAs on diffusion models, taking into account factors such as black-box/white-box models and the selection of attack features. We found that white-box attacks are highly applicable in real-world scenarios, and the most effective attacks presently are white-box. Departing from earlier research, which employs model loss as the attack feature for white-box MIAs, we employ model gradients in our attack, leveraging the fact that these gradients provide a more profound understanding of model responses to various samples. We subject these models to rigorous testing across a range of parameters, including training steps, sampling frequency, diffusion steps, and data variance. Across all experimental settings, our method consistently demonstrated near-flawless attack performance, with attack success rate approaching $100\%$ and attack AUCROC near $1.0$. We also evaluate our attack against common defense mechanisms, and observe our attacks continue to exhibit commendable performance.
更多查看译文
关键词
diffusion,attacks,models,white-box
AI 理解论文
溯源树
样例
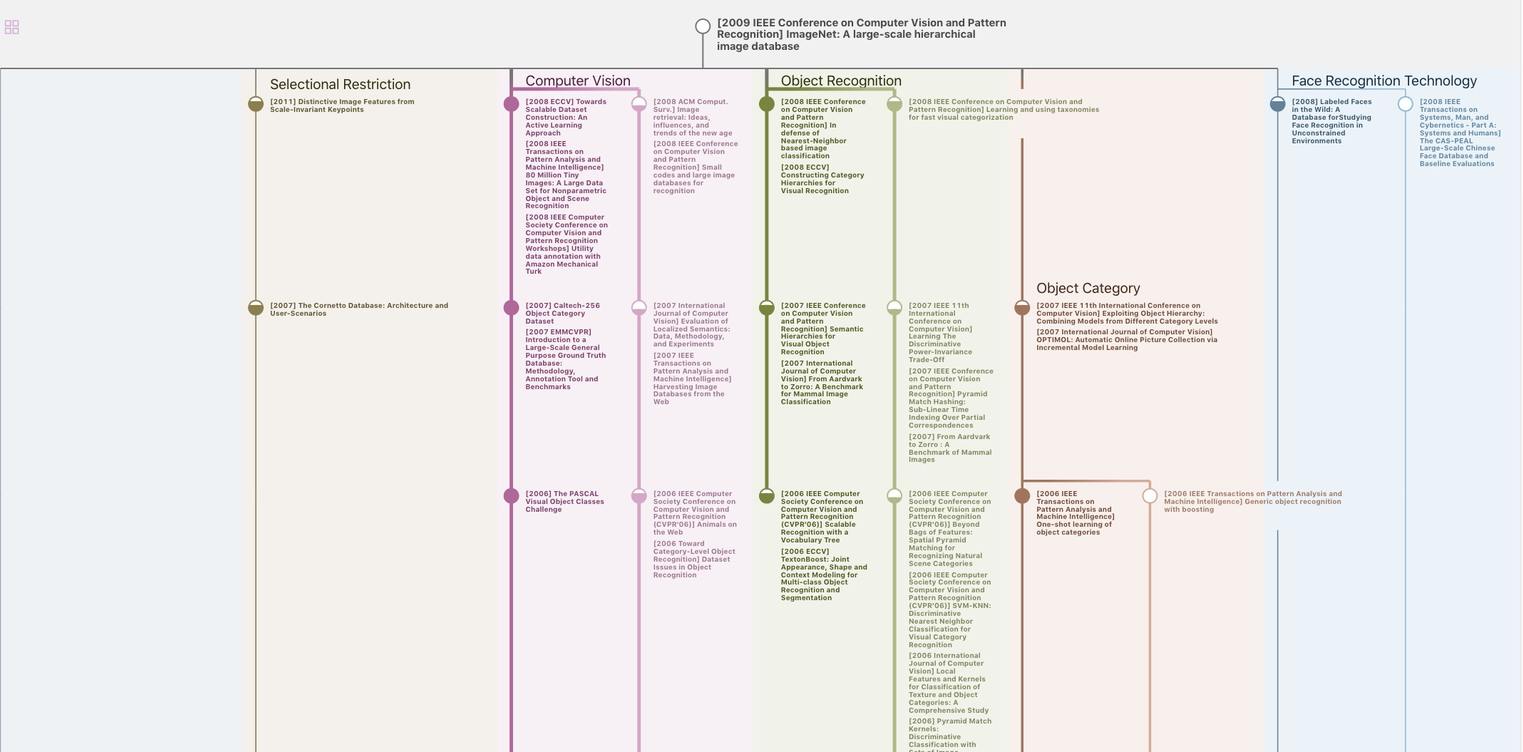
生成溯源树,研究论文发展脉络
Chat Paper
正在生成论文摘要