An attention-based deep learning method for right ventricular quantification using 2D echocardiography: Feasibility and accuracy
ECHOCARDIOGRAPHY-A JOURNAL OF CARDIOVASCULAR ULTRASOUND AND ALLIED TECHNIQUES(2024)
摘要
AimTo test the feasibility and accuracy of a new attention-based deep learning (DL) method for right ventricular (RV) quantification using 2D echocardiography (2DE) with cardiac magnetic resonance imaging (CMR) as reference.Methods and ResultsWe retrospectively analyzed images from 50 adult patients (median age 51, interquartile range 32-62 42% women) who had undergone CMR within 1 month of 2DE. RV planimetry of the myocardial border was performed in end-diastole (ED) and end-systole (ES) for eight standardized 2DE RV views with calculation of areas. The DL model comprised a Feature Tokenizer module and a stack of Transformer layers. Age, gender and calculated areas were used as inputs, and the output was RV volume in ED/ES. The dataset was randomly split into training, validation and testing subsets (35, 5 and 10 patients respectively).Mean RVEDV, RVESV and RV ejection fraction (EF) were 163 +/- 70 mL, 82 +/- 42 mL and 51% +/- 8% respectively without differences among the subsets. The proposed method achieved good prediction of RV volumes (R2 = .953, absolute percentage error [APE] = 9.75% +/- 6.23%) and RVEF (APE = 7.24% +/- 4.55%). Per CMR, there was one patient with RV dilatation and three with RV dysfunction in the testing dataset. The DL model detected RV dilatation in 1/1 case and RV dysfunction in 4/3 cases.Methods and ResultsWe retrospectively analyzed images from 50 adult patients (median age 51, interquartile range 32-62 42% women) who had undergone CMR within 1 month of 2DE. RV planimetry of the myocardial border was performed in end-diastole (ED) and end-systole (ES) for eight standardized 2DE RV views with calculation of areas. The DL model comprised a Feature Tokenizer module and a stack of Transformer layers. Age, gender and calculated areas were used as inputs, and the output was RV volume in ED/ES. The dataset was randomly split into training, validation and testing subsets (35, 5 and 10 patients respectively).Mean RVEDV, RVESV and RV ejection fraction (EF) were 163 +/- 70 mL, 82 +/- 42 mL and 51% +/- 8% respectively without differences among the subsets. The proposed method achieved good prediction of RV volumes (R2 = .953, absolute percentage error [APE] = 9.75% +/- 6.23%) and RVEF (APE = 7.24% +/- 4.55%). Per CMR, there was one patient with RV dilatation and three with RV dysfunction in the testing dataset. The DL model detected RV dilatation in 1/1 case and RV dysfunction in 4/3 cases.ConclusionsAn attention-based DL method for 2DE RV quantification showed feasibility and promising accuracy. The method requires validation in larger cohorts with wider range of RV size and function. Further research will focus on the reduction of the number of required 2DE to make the method clinically applicable.
更多查看译文
关键词
deep learning,echocardiography,machine learning,quantification,right ventricle
AI 理解论文
溯源树
样例
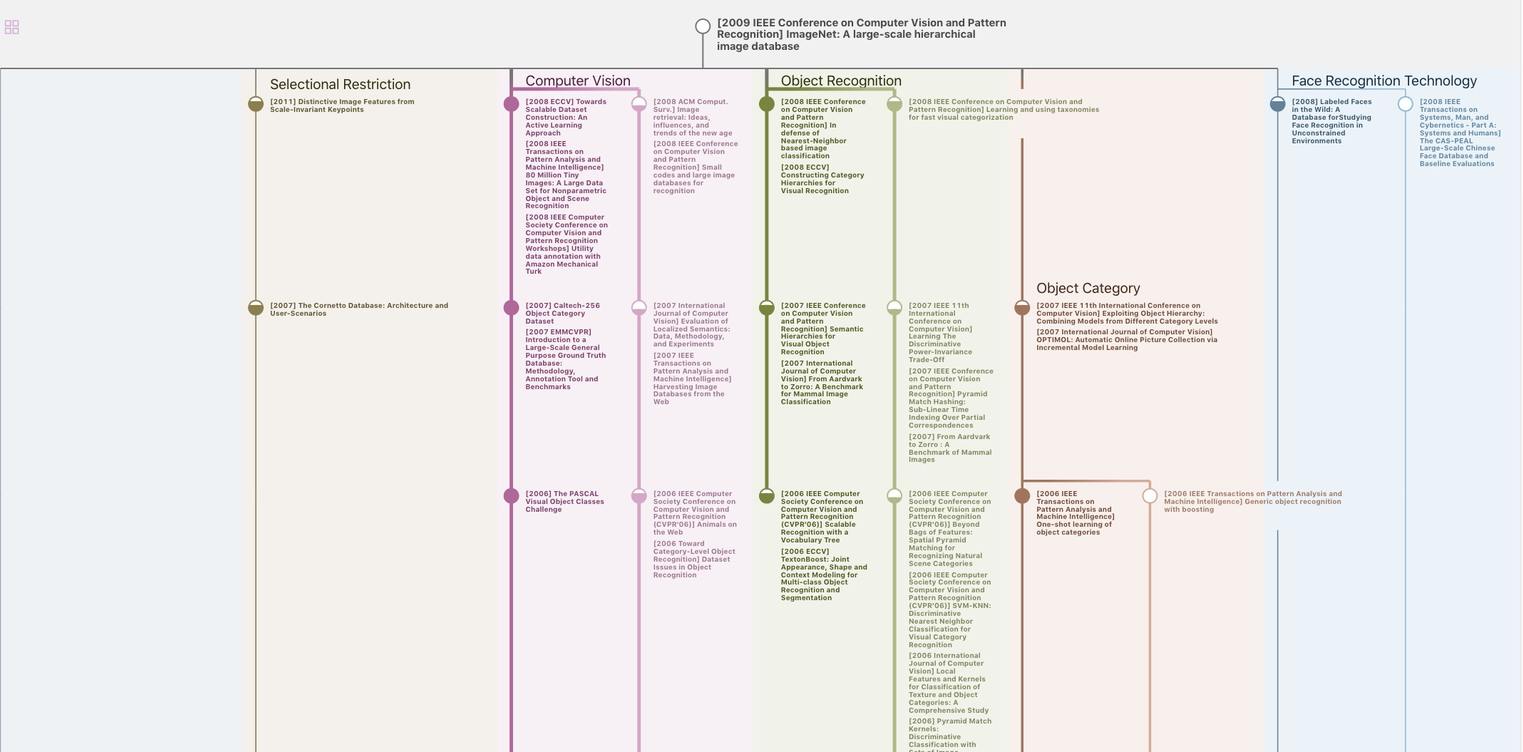
生成溯源树,研究论文发展脉络
Chat Paper
正在生成论文摘要