Deep-learning-based radiointerferometric imaging with GAN-aided training
Astronomy and Astrophysics(2023)
摘要
Context. The incomplete coverage of the spatial Fourier space, which leads to imaging artifacts, has been troubling radio interferometry for a long time. The currently best technique is to create an image for which the visibility data are Fourier-transformed and to clean the systematic effects originating from incomplete data in Fourier space. We have shown previously how super-resolution methods based on convolutional neural networks can reconstruct sparse visibility data. Aims. The training data in our previous work were not very realistic. The aim of this work is to build a whole simulation chain for realistic radio sources that then leads to an improved neural net for the reconstruction of missing visibilities. This method offers considerable improvements in terms of speed, automatization, and reproducibility over the standard techniques. Methods. We generated large amounts of training data by creating images of radio galaxies with a generative adversarial network that was trained on radio survey data. Then, we applied the radio interferometer measurement equation in order to simulate the measurement process of a radio interferometer. Results. We show that our neural network can faithfully reconstruct images of realistic radio galaxies. The reconstructed images agree well with the original images in terms of the source area, integrated flux density, peak flux density, and the multiscale structural similarity index. Finally, we show that the neural net can be adapted for estimating the uncertainties in the imaging process.
更多查看译文
关键词
radiointerferometric imaging,deep-learning-based deep-learning-based,gan-aided
AI 理解论文
溯源树
样例
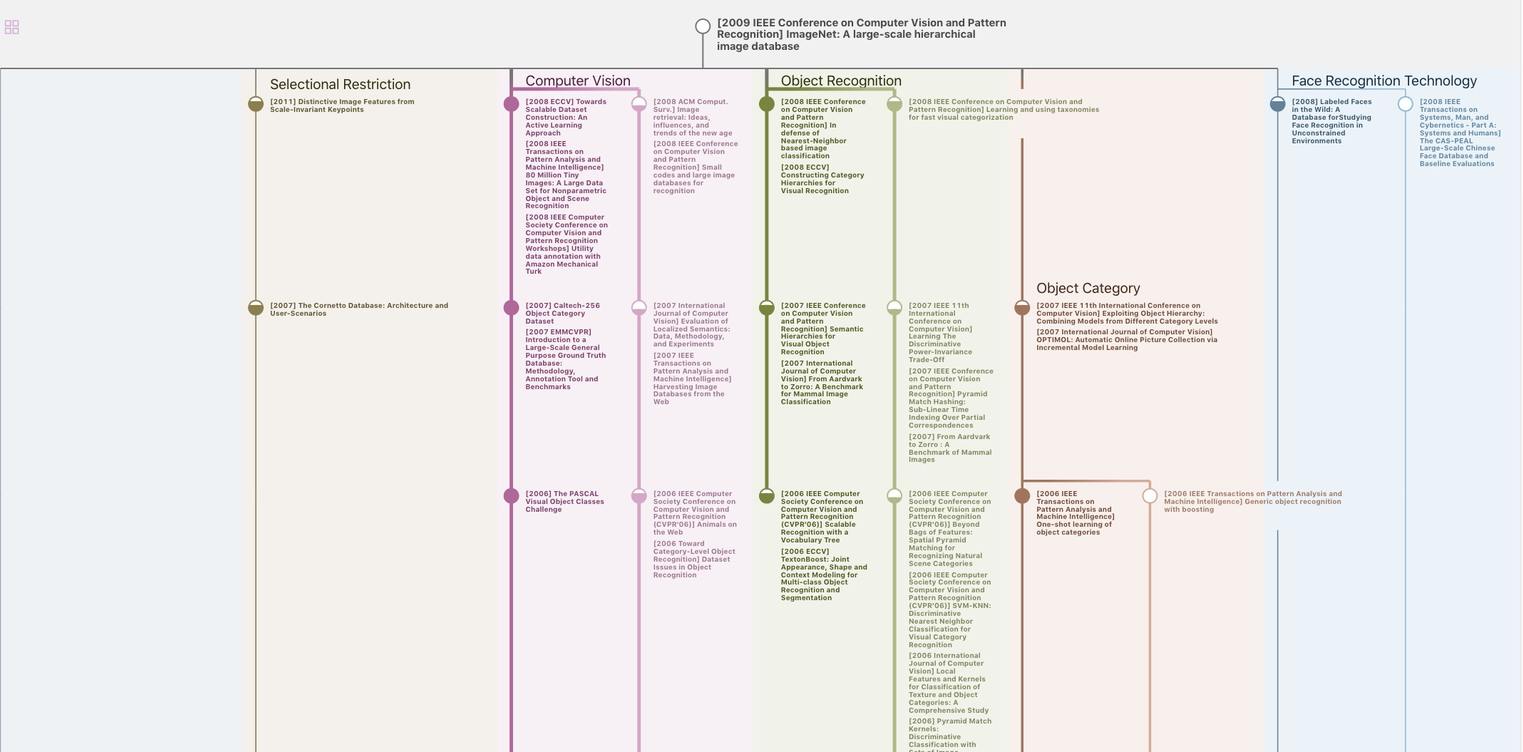
生成溯源树,研究论文发展脉络
Chat Paper
正在生成论文摘要