Response
Chest(2023)
摘要
We thank Dr McGaghie for his insightful comments regarding our articles published in CHEST.1Gefter W.B. Hatabu H. Reducing errors resulting from commonly missed chest radiography findings.Chest. 2023; 163: 634-649Abstract Full Text Full Text PDF PubMed Scopus (0) Google Scholar,2Gefter W.B. Post B.A. Hatabu H. Commonly missed findings on chest radiographs: causes and consequences.Chest. 2023; 163: 650-661Abstract Full Text Full Text PDF PubMed Scopus (0) Google Scholar Because perceptual errors are the predominant cause of missed findings on chest radiographs (CXR), we agree that perceptual training methods, although largely investigational to date, offer another important approach to reducing these errors.3Waite S. Farooq Z. Grigorian A. et al.A review of perceptual expertise in radiology: how it develops, how we can test it, and why humans still matter in the era of artificial intelligence.Acad Radiol. 2020; 27: 26-38Abstract Full Text Full Text PDF Scopus (21) Google Scholar Supervised reading at the workstation by trainees (the “apprentice-style” approach) traditionally has been used to develop perceptual skills in CXR interpretation and the ability to detect abnormalities. However, it is a slow and incomplete process. This can be intensified and accelerated by “deliberate practice.” The latter is characterized by focused, repetitive, rapid, and purposeful training to improve a new skill, with immediate expert feedback that allows for behavioral modification, and with specific measurable results. Perceptual training in radiology was suggested 40 years ago by Kundel and Nodine,4Kundel H.L. Nodine C.F. A visual concept shapes image perception.Radiology. 1983; 146: 363-368Crossref PubMed Scopus (0) Google Scholar who are pioneers in medical image perception. Subsequent studies have confirmed that methods specifically designed for perceptual training and testing can result in measurable improvements in accuracy and reduction of detection errors. These techniques have used computer-based learning and simulations that replicate the clinical reading environment. The training uses large numbers of cases with the goal of identifying images with and without specific radiographic abnormalities, presented in a rapid-fire manner with immediate feedback. No specific strategies for the detection of abnormalities are suggested to the trainee. This purposeful practice can be presented in a game-like fashion analogous to the training of machine learning algorithms with the use of reinforcement learning.5Chen H.-P. Roth H. Galperin-Aizenberg M. Ruutianen A.T. Gefter W. Cook T.S. Improving abnormality detection on chest radiography using game-like reinforcement mechanics.Acad Radiol. 2017; 24: 1428-1435Abstract Full Text Full Text PDF PubMed Scopus (21) Google Scholar The benefit of perceptual training is illustrated in a study of the detection of femoral neck fractures, in which medically naive subjects who underwent deliberate perceptual training for less than 1 hour could achieve performance levels comparable to board-certified radiologists and to artificial intelligence deep-learning algorithms (with the use of an equivalent number of training images).6Adams M. Chen W. Holcdorf D. McCusker M.W. Hove P.D.L. Gaillard F. Computer vs human: deep learning versus perceptual training for the detection of neck of femur fractures.J Med Imaging Radiat Oncol. 2019; 63: 27-32Crossref PubMed Scopus (67) Google Scholar Further studies will need to assess retention of the acquired perceptual skills. The remarkable ability of humans to learn to detect abnormalities in a short amount of time and with limited numbers of cases is often overshadowed by our current enthusiasm for artificial intelligence. Cases collected to train artificial intelligence algorithms could also be used to train radiologists with the use of such purposeful, focused practice methods. Thus, as Dr McGaghie has advocated, deliberate practice to develop expert lesion detection skills should be included among the armamentarium of approaches that we have proposed to reduce errors caused by missed findings in CXR interpretation.1Gefter W.B. Hatabu H. Reducing errors resulting from commonly missed chest radiography findings.Chest. 2023; 163: 634-649Abstract Full Text Full Text PDF PubMed Scopus (0) Google Scholar,2Gefter W.B. Post B.A. Hatabu H. Commonly missed findings on chest radiographs: causes and consequences.Chest. 2023; 163: 650-661Abstract Full Text Full Text PDF PubMed Scopus (0) Google Scholar See earlier cited article for author conflicts of interest. Education for Chest Radiograph Interpretation Performance ImprovementCHESTVol. 164Issue 2PreviewA recent article authored by Gefter and Hatabu1 was published in CHEST (March 2023). The authors educated readers about “reducing errors from commonly missed chest radiography findings.” In a companion article, the authors point out that, despite longitudinal training, medical radiology is a human system in which a margin of chest radiograph (CXR) interpretation error that ranges from 4% to 30% is always present.2 The sources of radiology interpretation error include cognitive biases, image quality, clinical volume, and hospital custom. Full-Text PDF Reducing Errors Resulting From Commonly Missed Chest Radiography FindingsCHESTVol. 163Issue 3PreviewChest radiography (CXR), the most frequently performed imaging examination, is vulnerable to interpretation errors resulting from commonly missed findings. Methods to reduce these errors are presented. A practical approach using a systematic and comprehensive visual search strategy is described. The use of a checklist for quality control in the interpretation of CXR images is proposed to avoid overlooking commonly missed findings of clinical importance. Artificial intelligence is among the emerging and promising methods to enhance detection of CXR abnormalities. Full-Text PDF Commonly Missed Findings on Chest Radiographs: Causes and ConsequencesCHESTVol. 163Issue 3PreviewChest radiography (CXR) continues to be the most frequently performed imaging examination worldwide, yet it remains prone to frequent errors in interpretation. These pose potential adverse consequences to patients and are a leading motivation for medical malpractice lawsuits. Commonly missed CXR findings and the principal causes of these errors are reviewed and illustrated. Perceptual errors are the predominant source of these missed findings. The medicolegal implications of such errors are explained. Full-Text PDF
更多查看译文
关键词
response
AI 理解论文
溯源树
样例
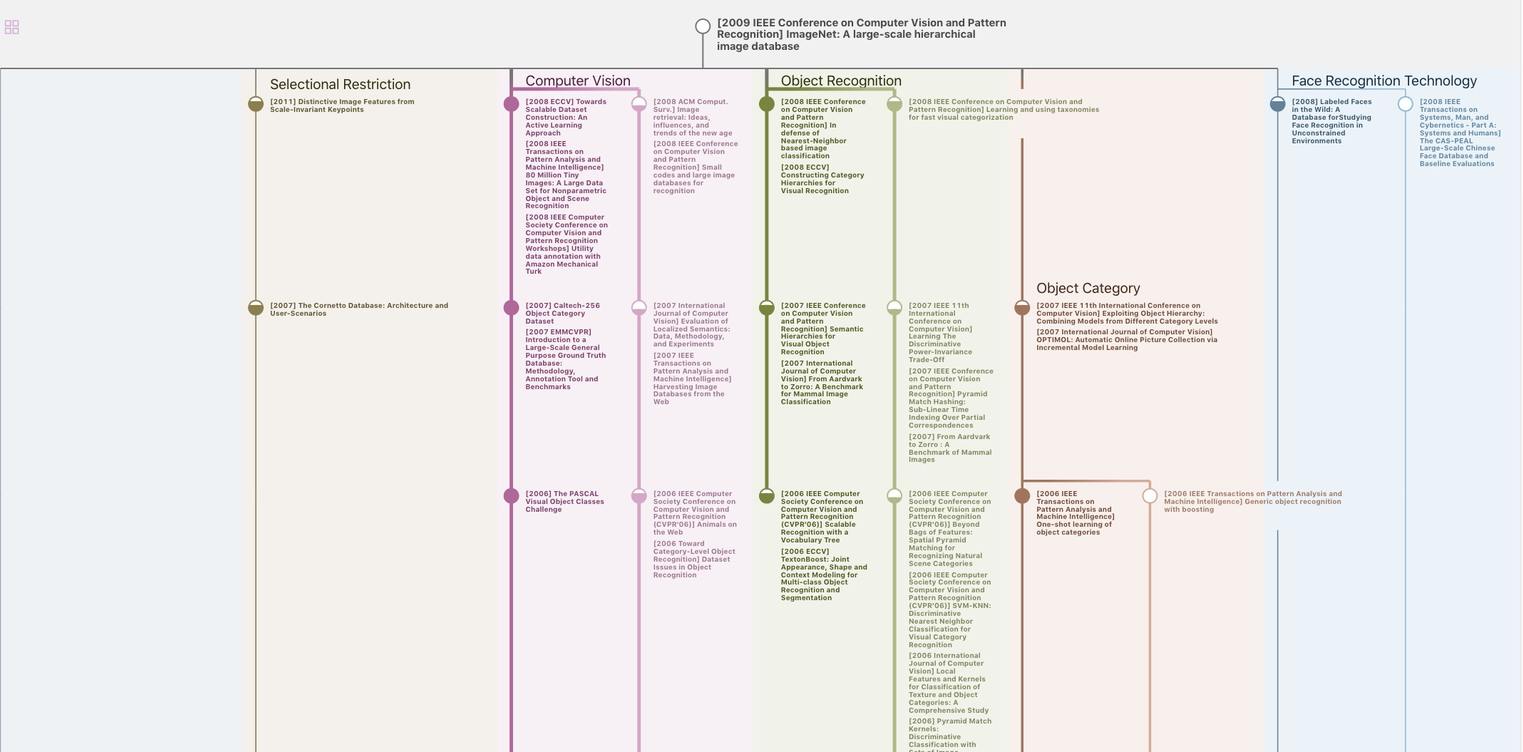
生成溯源树,研究论文发展脉络
Chat Paper
正在生成论文摘要