Both Spatial and Frequency Cues Contribute to High-Fidelity Image Inpainting
arXiv (Cornell University)(2023)
摘要
Deep generative approaches have obtained great success in image inpainting recently. However, most generative inpainting networks suffer from either over-smooth results or aliasing artifacts. The former lacks high-frequency details, while the latter lacks semantic structure. To address this issue, we propose an effective Frequency-Spatial Complementary Network (FSCN) by exploiting rich semantic information in both spatial and frequency domains. Specifically, we introduce an extra Frequency Branch and Frequency Loss on the spatial-based network to impose direct supervision on the frequency information, and propose a Frequency-Spatial Cross-Attention Block (FSCAB) to fuse multi-domain features and combine the corresponding characteristics. With our FSCAB, the inpainting network is capable of capturing frequency information and preserving visual consistency simultaneously. Extensive quantitative and qualitative experiments demonstrate that our inpainting network can effectively achieve superior results, outperforming previous state-of-the-art approaches with significantly fewer parameters and less computation cost. The code will be released soon.
更多查看译文
关键词
frequency cues,spatial,image
AI 理解论文
溯源树
样例
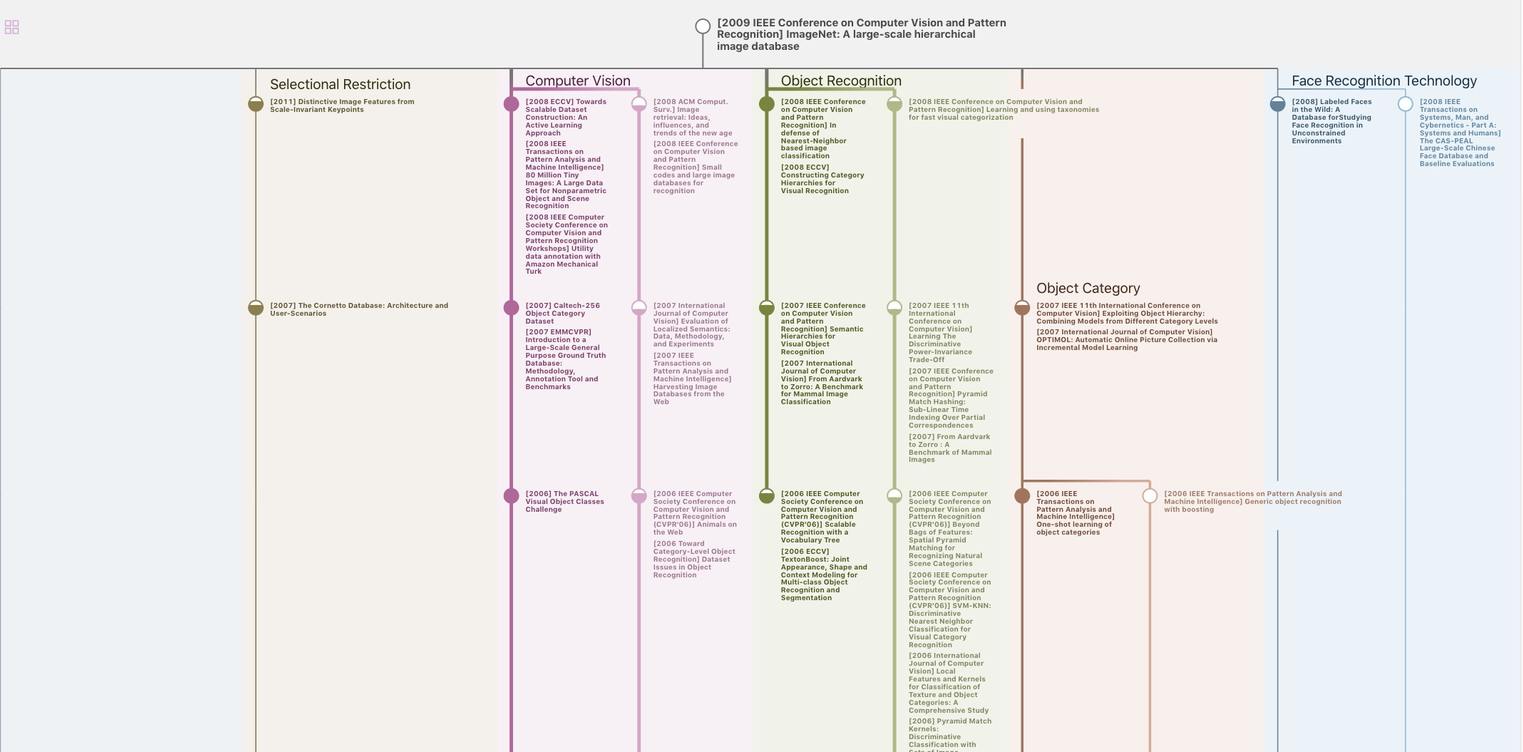
生成溯源树,研究论文发展脉络
Chat Paper
正在生成论文摘要