An Evolution Kernel Method for Graph Classification through Heat Diffusion Dynamics
arXiv (Cornell University)(2023)
摘要
Autonomous individuals establish a structural complex system through pairwise connections and interactions. Notably, the evolution reflects the dynamic nature of each complex system since it recodes a series of temporal changes from the past, the present into the future. Different systems follow distinct evolutionary trajectories, which can serve as distinguishing traits for system classification. However, modeling a complex system's evolution is challenging for the graph model because the graph is typically a snapshot of the static status of a system, and thereby hard to manifest the long-term evolutionary traits of a system entirely. To address this challenge, we suggest utilizing a heat-driven method to generate temporal graph augmentation. This approach incorporates the physics-based heat kernel and DropNode technique to transform each static graph into a sequence of temporal ones. This approach effectively describes the evolutional behaviours of the system, including the retention or disappearance of elements at each time point based on the distributed heat on each node. Additionally, we propose a dynamic time-wrapping distance GDTW to quantitatively measure the distance between pairwise evolutionary systems through optimal matching. The resulting approach, called the Evolution Kernel method, has been successfully applied to classification problems in real-world structural graph datasets. The results yield significant improvements in supervised classification accuracy over a series of baseline methods.
更多查看译文
关键词
graph classification,evolution kernel method,diffusion,dynamics
AI 理解论文
溯源树
样例
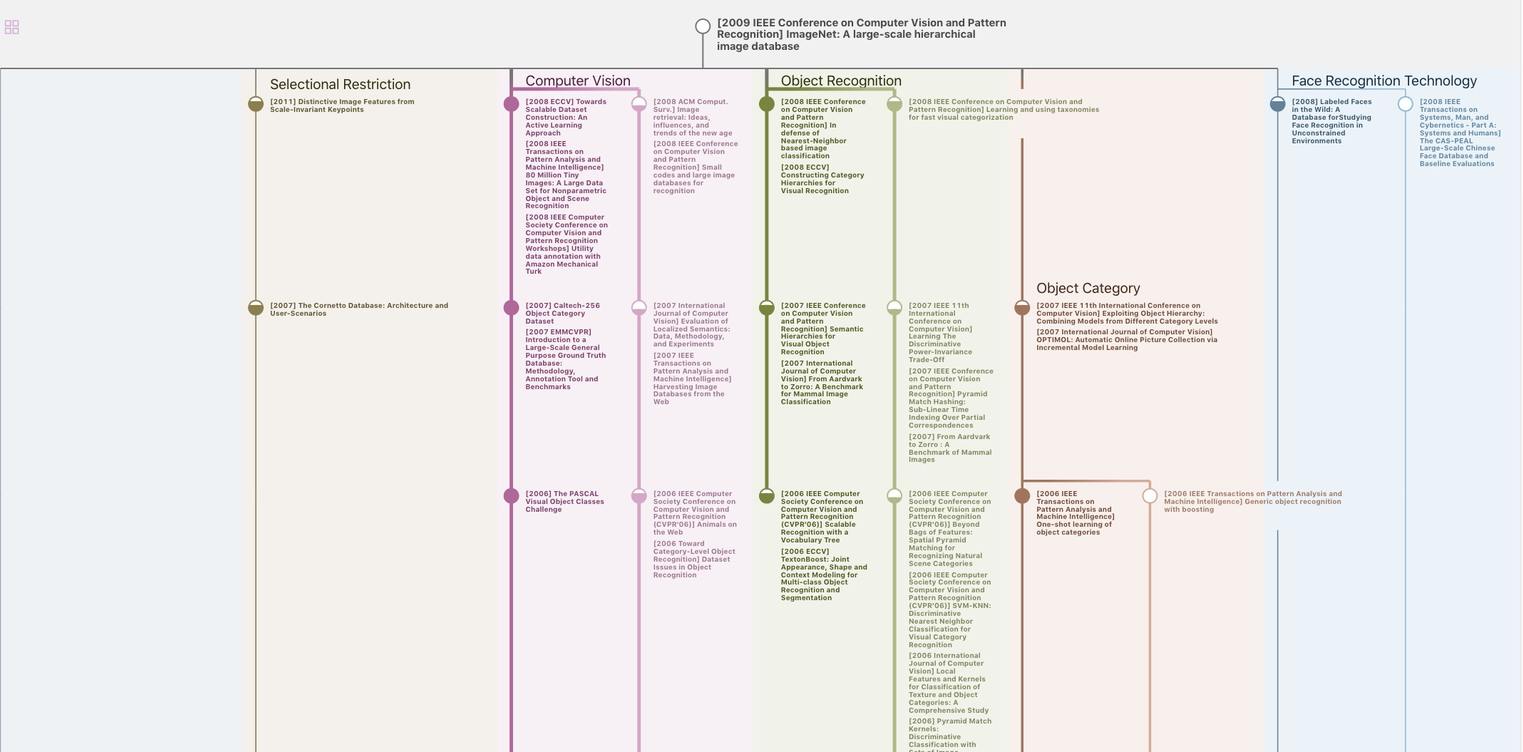
生成溯源树,研究论文发展脉络
Chat Paper
正在生成论文摘要