Predictive Multiplicity in Probabilistic Classification
Proceedings of the ... AAAI Conference on Artificial Intelligence(2023)
摘要
Machine learning models are often used to inform real world risk assessment tasks: predicting consumer default risk, predicting whether a person suffers from a serious illness, or predicting a person's risk to appear in court. Given multiple models that perform almost equally well for a prediction task, to what extent do predictions vary across these models? If predictions are relatively consistent for similar models, then the standard approach of choosing the model that optimizes a penalized loss suffices. But what if predictions vary significantly for similar models? In machine learning, this is referred to as predictive multiplicity i.e. the prevalence of conflicting predictions assigned by near-optimal competing models. In this paper, we present a framework for measuring predictive multiplicity in probabilistic classification (predicting the probability of a positive outcome). We introduce measures that capture the variation in risk estimates over the set of competing models, and develop optimization-based methods to compute these measures efficiently and reliably for convex empirical risk minimization problems. We demonstrate the incidence and prevalence of predictive multiplicity in real-world tasks. Further, we provide insight into how predictive multiplicity arises by analyzing the relationship between predictive multiplicity and data set characteristics (outliers, separability, and majority-minority structure). Our results emphasize the need to report predictive multiplicity more widely.
更多查看译文
关键词
probabilistic classification,multiplicity
AI 理解论文
溯源树
样例
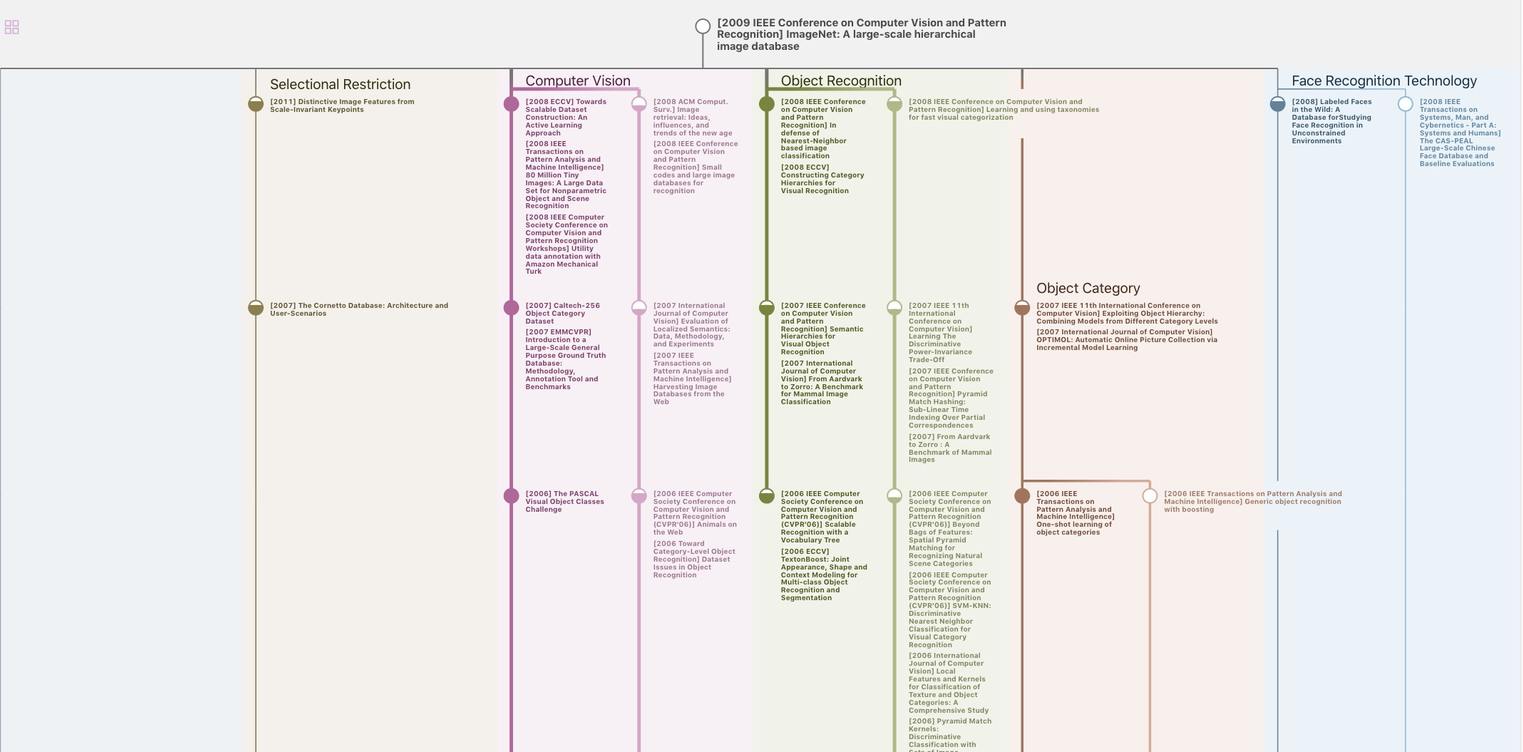
生成溯源树,研究论文发展脉络
Chat Paper
正在生成论文摘要