Detecting Facial Action Units From Global-Local Fine-Grained Expressions.
IEEE Trans. Circuits Syst. Video Technol.(2024)
摘要
Since Facial Action Unit (AU) annotations require domain expertise, common AU datasets only contain a limited number of subjects. As a result, a crucial challenge for AU detection is addressing identity overfitting. We find that AUs and facial expressions are highly associated, and existing facial expression datasets often contain a large number of identities. In this paper, we aim to utilize the expression datasets without AU labels to facilitate AU detection. Specifically, we develop a novel AU detection framework aided by the Global-Local facial Expressions Embedding, dubbed GLEE-Net. Our GLEE-Net consists of three branches to extract identity-independent expression features for AU detection. We introduce a global branch for modeling the overall facial expression while eliminating the impacts of identities. We also design a local branch focusing on specific local face regions. The combined output of global and local branches is firstly pre-trained on an expression dataset as an identity-independent expression embedding, and then finetuned on AU datasets. Therefore, we significantly alleviate the issue of limited identities. Furthermore, we introduce a 3D global branch that extracts expression coefficients through 3D face reconstruction to consolidate 2D AU descriptions. Finally, a Transformer-based multi-label classifier is employed to fuse all the representations for AU detection. Extensive experiments demonstrate that our method significantly outperforms the state-of-the-art on the widely-used DISFA, BP4D and BP4D+ datasets.
更多查看译文
关键词
Action Units,facial expression,expression embedding,deep learning
AI 理解论文
溯源树
样例
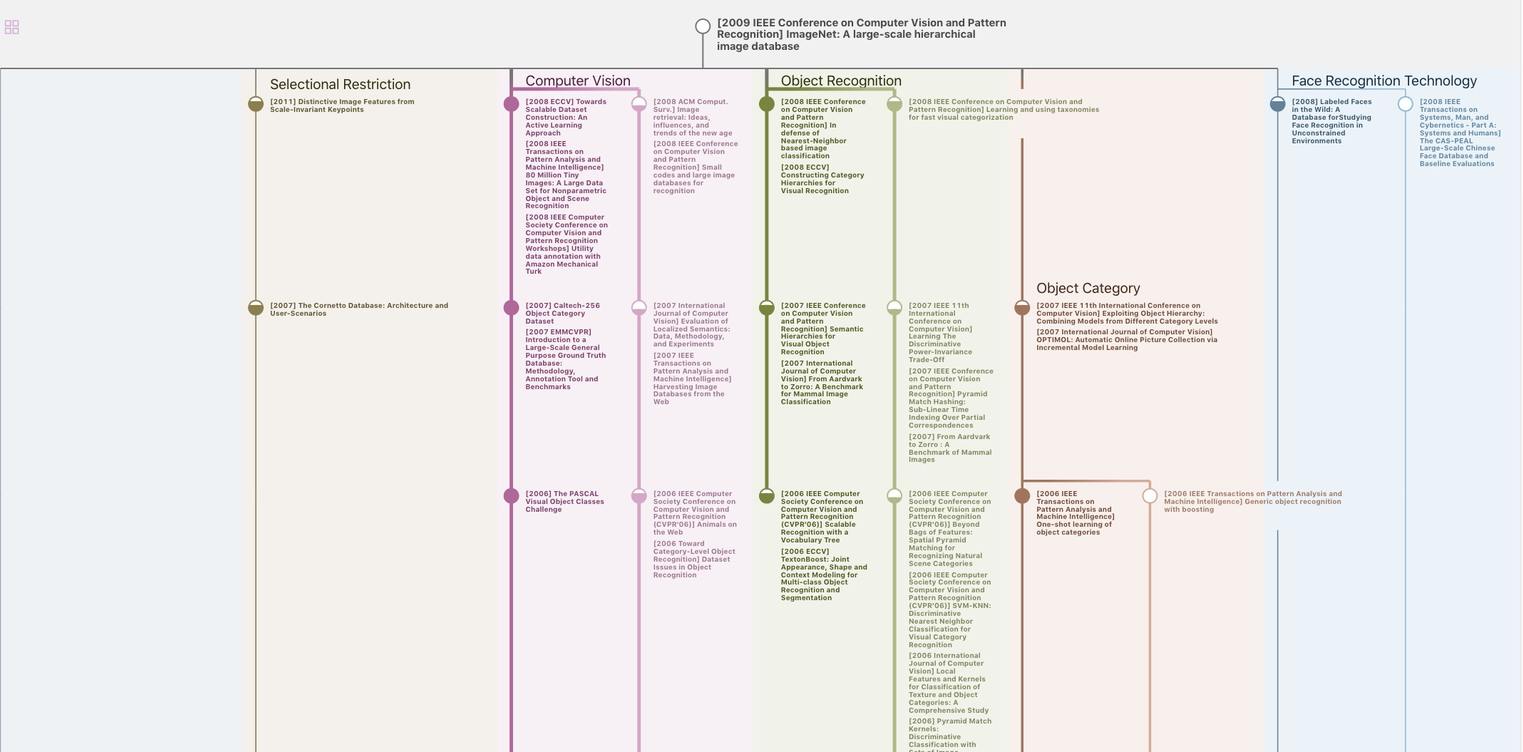
生成溯源树,研究论文发展脉络
Chat Paper
正在生成论文摘要