Towards Ranking of Gene Regulatory Network Inference Methods Based on Prediction Quality
Algorithms for intelligent systems(2023)
摘要
Gene Regulatory Networks (GRNs) equip researchers with pivotal information about the diverse cellular processes that occur in an organism. Therefore, the inference of GRNs is crucial for different biological research areas such as network medicine, drug design, and biomarker prediction. As a result, numerous methods have been developed over the years for inferring GRNs. Not much is known about these methods’ prediction quality except for characteristics like AUROC (Area Under Receiver Operating Characteristic) and AUPR (Area under Precision Recall). These characteristics, however, are insufficient to quantify the prediction quality of the GRNs effectively. Therefore, in this paper, we perform an extensive comparative analysis of ten popular inference methods and rank them based on their prediction quality. After computing the metrics and ranking the methods, we find MINE, CLR, G1DBN, and BC3NET to be the best-performing methods. We also observe that the popular methods analyzed herein show a dismal performance when applied to synthetic datasets. Despite including methods with different underlying mathematical rationales and assumptions, we could not infer most of the true interactions in all networks. The results of this work stress the need for enhanced inference methods that assure quality predictions.
更多查看译文
关键词
gene,regulatory,ranking,prediction
AI 理解论文
溯源树
样例
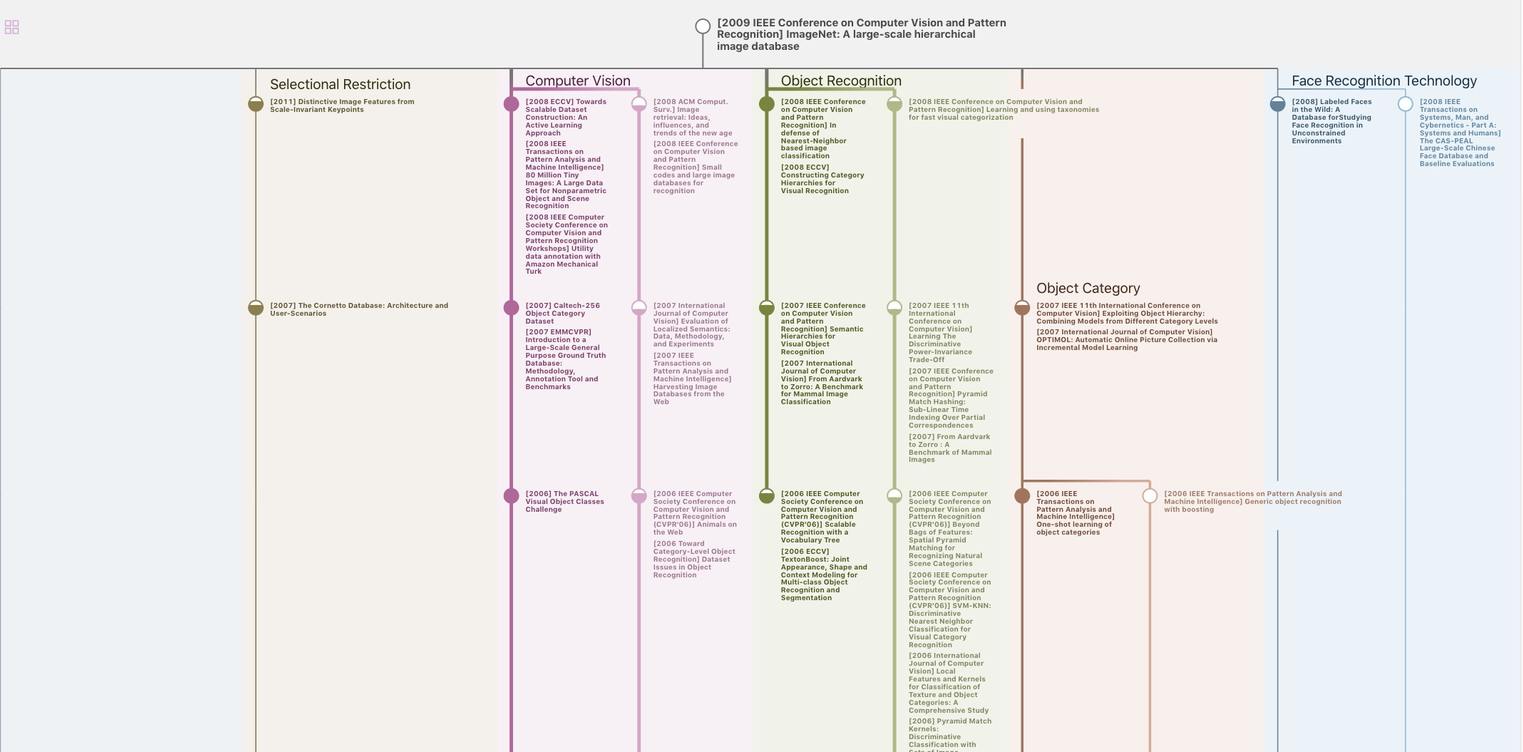
生成溯源树,研究论文发展脉络
Chat Paper
正在生成论文摘要