KuaiSAR: A Unified Search And Recommendation Dataset
arXiv (Cornell University)(2023)
摘要
The confluence of Search and Recommendation (S&R) services is vital to online services, including e-commerce and video platforms. The integration of S&R modeling is a highly intuitive approach adopted by industry practitioners. However, there is a noticeable lack of research conducted in this area within academia, primarily due to the absence of publicly available datasets. Consequently, a substantial gap has emerged between academia and industry regarding research endeavors in joint optimization using user behavior data from both S&R services. To bridge this gap, we introduce the first large-scale, real-world dataset KuaiSAR of integrated Search And Recommendation behaviors collected from Kuaishou, a leading short-video app in China with over 350 million daily active users. Previous research in this field has predominantly employed publicly available semi-synthetic datasets and simulated, with artificially fabricated search behaviors. Distinct from previous datasets, KuaiSAR contains genuine user behaviors, including the occurrence of each interaction within either search or recommendation service, and the users' transitions between the two services. This work aids in joint modeling of S&R, and utilizing search data for recommender systems (and recommendation data for search engines). Furthermore, due to the various feedback labels associated with user-video interactions, KuaiSAR also supports a broad range of tasks, including intent recommendation, multi-task learning, and modeling of long sequential multi-behavioral patterns. We believe this dataset will serve as a catalyst for innovative research and bridge the gap between academia and industry in understanding the S&R services in practical, real-world applications.
更多查看译文
关键词
recommendation dataset,unified search
AI 理解论文
溯源树
样例
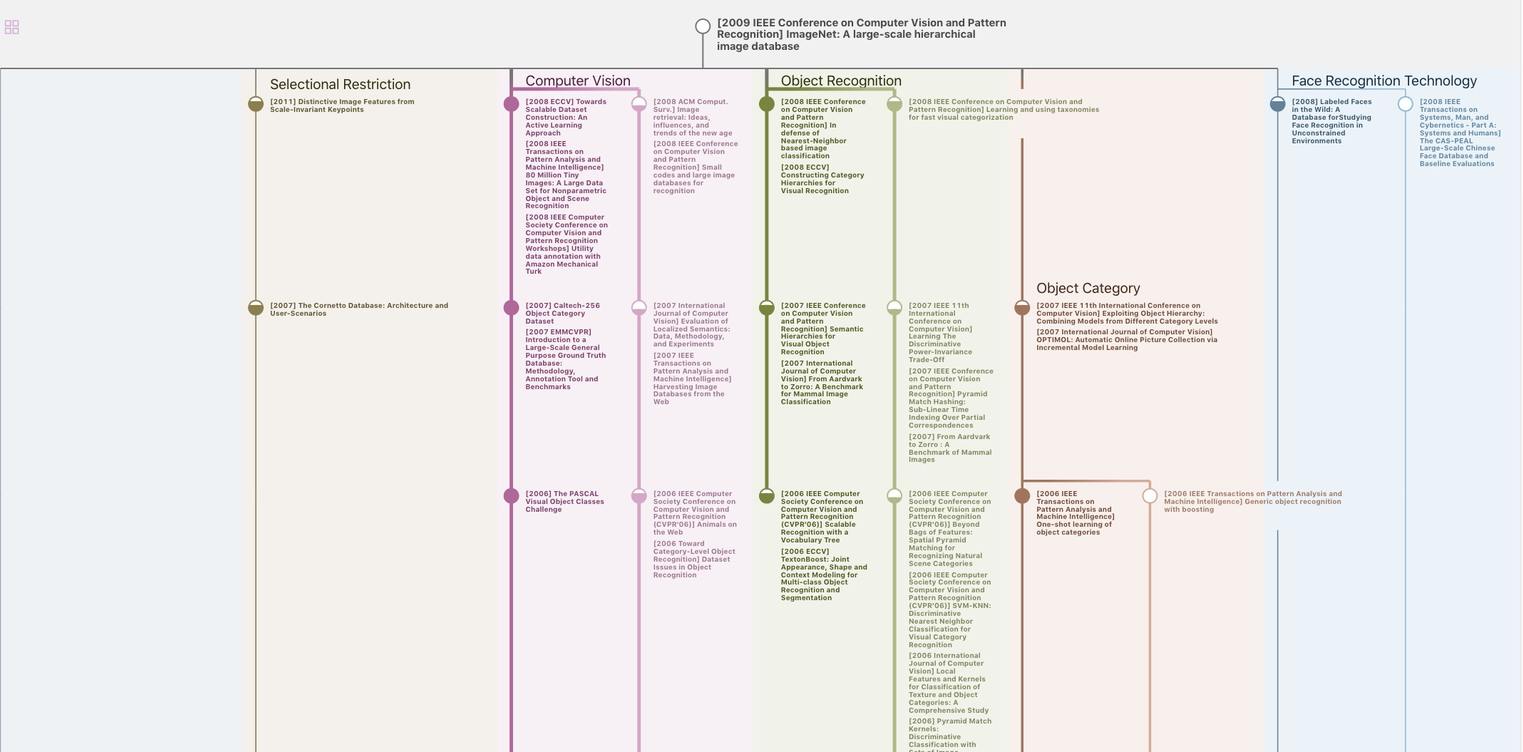
生成溯源树,研究论文发展脉络
Chat Paper
正在生成论文摘要