DomainFeat: Learning Local Features With Domain Adaptation
IEEE TRANSACTIONS ON CIRCUITS AND SYSTEMS FOR VIDEO TECHNOLOGY(2024)
摘要
Accurate and efficient keypoint detection and description is a fundamental step in various computer vision tasks. In this paper, we extract robust descriptors and detect accurate keypoints by learning local Features with Domain adaptation (DomainFeat). Specifically, our Domainfeat includes image-level domain invariance supervision, pixel-level domain consistency supervision, Pixel-Adaptive keypoint Detection(PA-Det), and cross-domain dataset with domain stable point supervision. First, we introduce the image-level domain invariance supervision to make the high-level feature distributions from different domains close by fusing domain-invariant representations in the decoder. Furthermore, to compensate for the inconsistency between descriptors corresponding to the keypoints at the pixel level, we propose the pixel-level domain consistency supervision. Then we present the Pixel-Adaptive keypoint Detection to efficiently detect accurate keypoints, which can improve accuracy by enhancing the local consistency of heatmaps. Finally, we propose an efficient approach to construct data and supervision labels in diverse domains, which can tackle complex application scenarios. With these novel modules and supervision methods, our DomainFeat can make feature detectors more accurate and descriptors more robust. Extensive experiments confirm that Domainfeat achieves state-of-the-art performance on benchmarks such as Aachen-Day-Night localization, HPatches image matching, and the challenging DNIM dataset.
更多查看译文
关键词
Feature extraction,Location awareness,Visualization,Robustness,Image matching,Detectors,Decoding,Local features,domain adaptation,cross-domain data,consistency loss
AI 理解论文
溯源树
样例
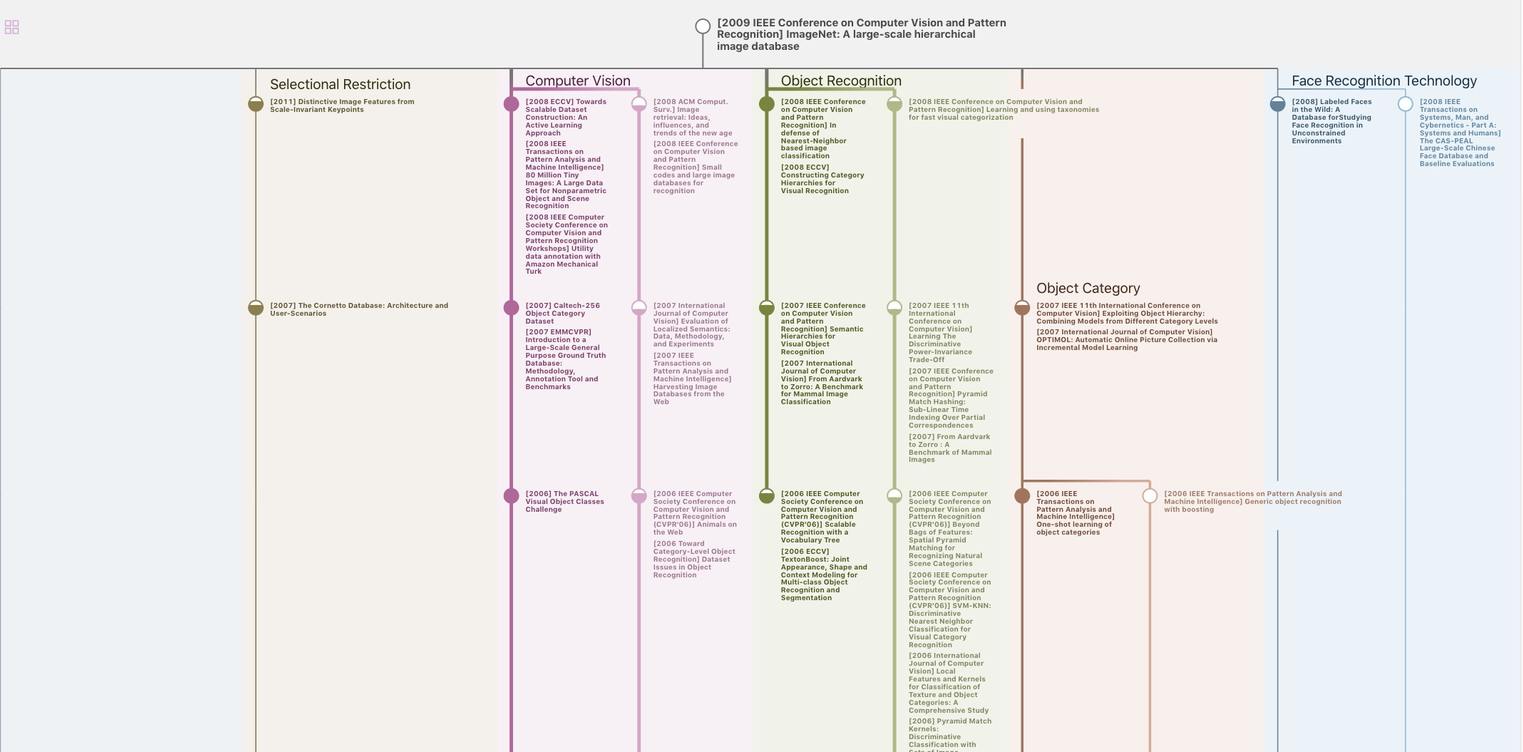
生成溯源树,研究论文发展脉络
Chat Paper
正在生成论文摘要