Generating Images of Rare Concepts Using Pre-trained Diffusion Models
AAAI 2024(2024)
摘要
Text-to-image diffusion models can synthesize high quality images, but they have various limitations. Here we highlight a common failure mode of these models, namely, generating uncommon concepts and structured concepts like hand palms. We show that their limitation is partly due to the long-tail nature of their training data: web-crawled data sets are strongly unbalanced, causing models to under-represent concepts from the tail of the distribution. We characterize the effect of unbalanced training data on text-to-image models and offer a remedy. We show that rare concepts can be correctly generated by carefully selecting suitable generation seeds in the noise space, using a small reference set of images, a technique that we call SeedSelect. SeedSelect does not require retraining or finetuning the diffusion model. We assess the faithfulness, quality and diversity of SeedSelect in creating rare objects and generating complex formations like hand images, and find it consistently achieves superior performance. We further show the advantage of SeedSelect in semantic data augmentation. Generating semantically appropriate images can successfully improve performance in few-shot recognition benchmarks, for classes from the head and from the tail of the training data of diffusion models.
更多查看译文
关键词
CV: Computational Photography, Image & Video Synthesis,CV: Large Vision Models
AI 理解论文
溯源树
样例
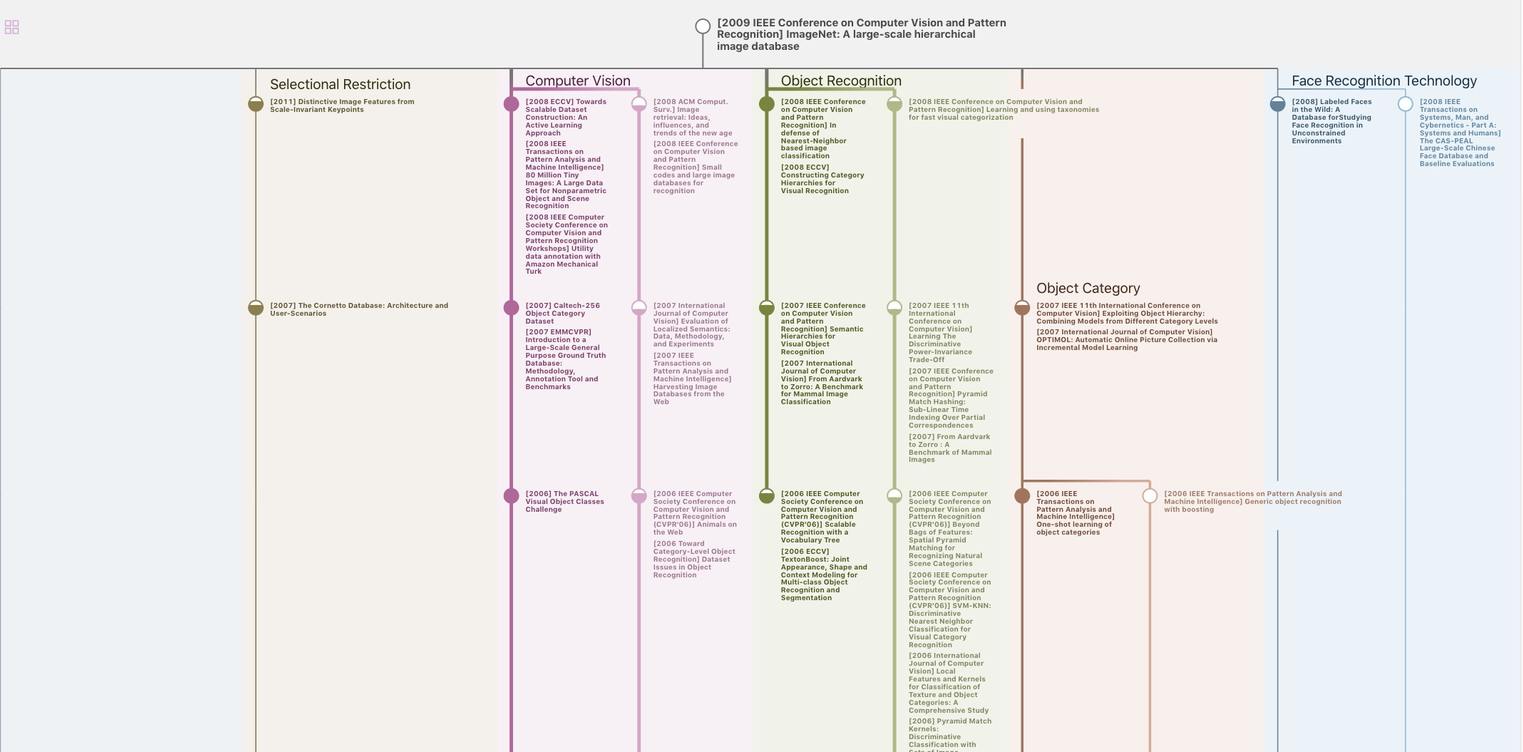
生成溯源树,研究论文发展脉络
Chat Paper
正在生成论文摘要