Skip Connection Aggregation Transformer for Occluded Person Reidentification
IEEE TRANSACTIONS ON INDUSTRIAL INFORMATICS(2024)
摘要
The occlusion problem is a significant challenge for person reidentification. Recently, transformer-based methods have been introduced to solve the occlusion problem and achieve performance improvements. However, the existing methods only apply the features of the last transformer layer and fail to consider the alignment of visible body parts. They also ignore fine-grained local features. Thus, they usually suffer from misalignment in occluded image matching. We observe that features from the high layers of the transformer focus on classification information and global features, while those from the middle layers pay more attention to pedestrians. We think that making full use of the features of different layers will facilitate alignment and then will promote reidentification accuracy. Therefore, we propose a novel skip connection aggregation transformer (SCAT) network by utilizing features from different transformer layers to increase the diversity of features and align visible body parts in occluded images. The diverse features include the following: first, features of the middle layer, which focus on the pedestrian in nonoccluded regions and favor alignment, second, features of high layers, which focus on global information, third fine-grained local features, which are obtained by the part pooling encoder and the fusion reconstruction module. The part pooling encoder and the fusion reconstruction module are proposed to obtain part-based local features and fused local features, respectively. The experimental results on the occluded, partial, and holistic benchmarks demonstrate that our method can significantly promote the accuracy of occluded person reidentification.
更多查看译文
关键词
Fine-grained local features,occluded person reidentification (ReID),pure vision transformer (ViT),skip connection aggregation
AI 理解论文
溯源树
样例
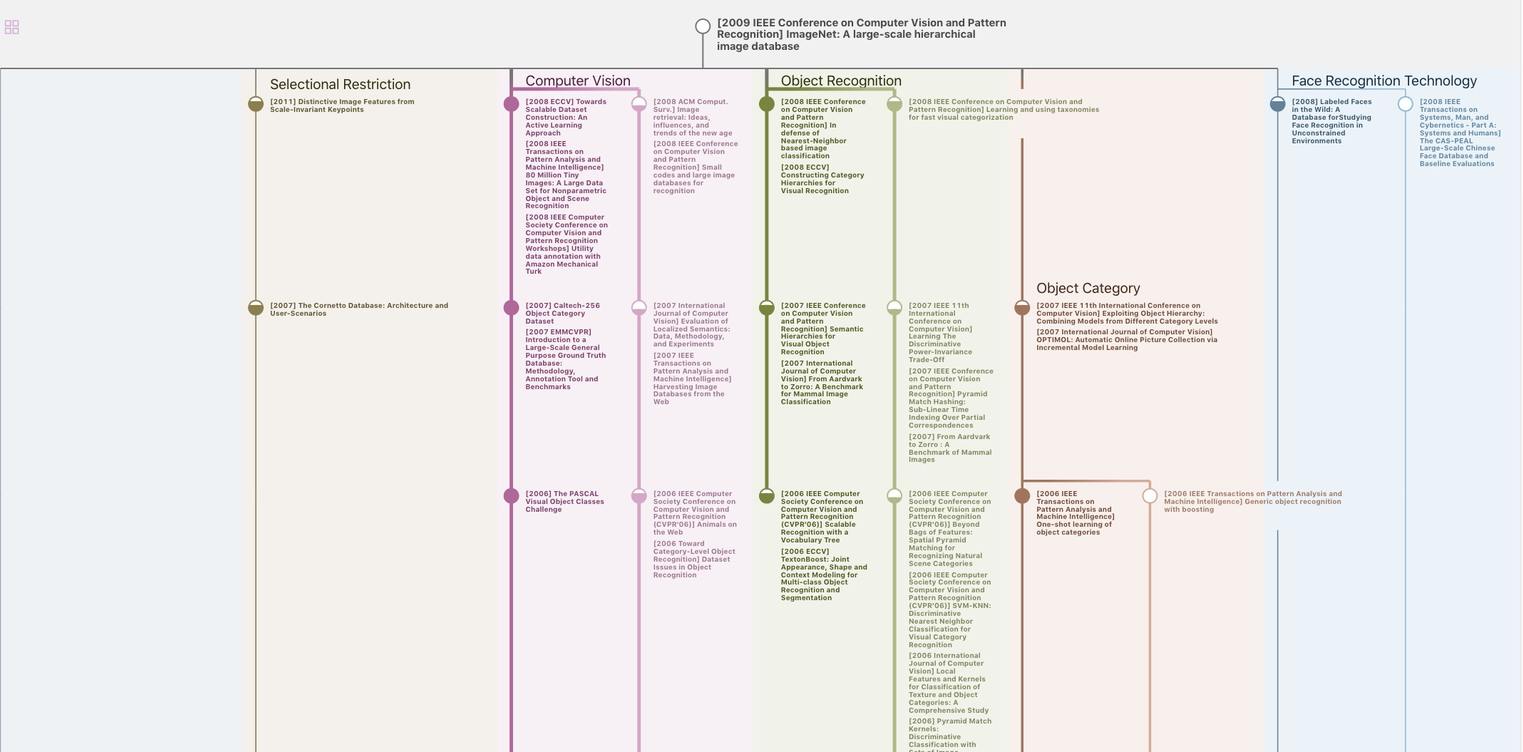
生成溯源树,研究论文发展脉络
Chat Paper
正在生成论文摘要