Hyper-Local Temperature Prediction Using Detailed Urban Climate Informatics
JOURNAL OF ADVANCES IN MODELING EARTH SYSTEMS(2024)
摘要
The accurate modeling of urban microclimate is a challenging task given the high surface heterogeneity of urban land cover and the vertical structure of street morphology. Recent years have witnessed significant efforts in numerical modeling and data collection of the urban environment. Nonetheless, it is difficult for the physical-based models to fully utilize the high-resolution data under the constraints of computing resources. The advancement in machine learning (ML) techniques offers the computational strength to handle the massive volume of data. In this study, we proposed a modeling framework that uses ML approach to estimate point-scale street-level air temperature from the urban-resolving meso-scale climate model and a suite of hyper-resolution urban geospatial data sets, including three-dimensional urban morphology, parcel-level land use inventory, and weather observations from a sensor network. We implemented this approach in the City of Chicago as a case study to demonstrate the capability of the framework. The proposed approach vastly improves the resolution of temperature predictions in cities, which will help the city with walkability, drivability, and heat-related behavioral studies. Moreover, we tested the model's reliability on out-of-sample locations to investigate the modeling uncertainties and the application potentials to the other areas. This study aims to gain insights into next-gen urban climate modeling and guide the observation efforts in cities to build the strength for the holistic understanding of urban microclimate dynamics. Estimating air temperature at street-level is a difficult task because of the complex environment in cities and the limitations of the current urban numerical models. In recent years, with the rapid development of data collection and analysis techniques, it is possible to fully utilize the hyper-local data harvested from urban areas by advanced machine learning algorithms. In this study, we present a modeling pipeline to estimate point-scale street-level air temperature from conventional urban weather model and a suite of hyper-resolution urban data sets. These data sets were collected using state-of-art techniques, such as sub-meter level Light Detection and Ranging technology and wireless weather observation network. We demonstrated the use of this modeling method over the City of Chicago and estimated the street-level temperature. The modeling results have multiple real-world applications, such as to provide navigation suggestions to reduce thermal discomfort of the pedestrians as an example. Moreover, it is possible to expand the use of our model to other areas given the current data availability. The results of this study can also help the development of the next-generation urban climate and weather models and guide observation efforts in cities. These together can build the strength for the holistic understanding of urban microclimate dynamics. The study presents a modeling framework to estimate street-level air temperature using a suite of detailed urban climate informatics Model results showed hyper-local urban features have significant impacts on street-level temperature but with a limited influence radius The investigations on model sensitivity implies the existence of the optimum scale in urban modeling and critical locations in observation
更多查看译文
关键词
urban climate informatics,hyper resolution,Weather Research and Forecast model,Gaussian process regression,street-level temperature
AI 理解论文
溯源树
样例
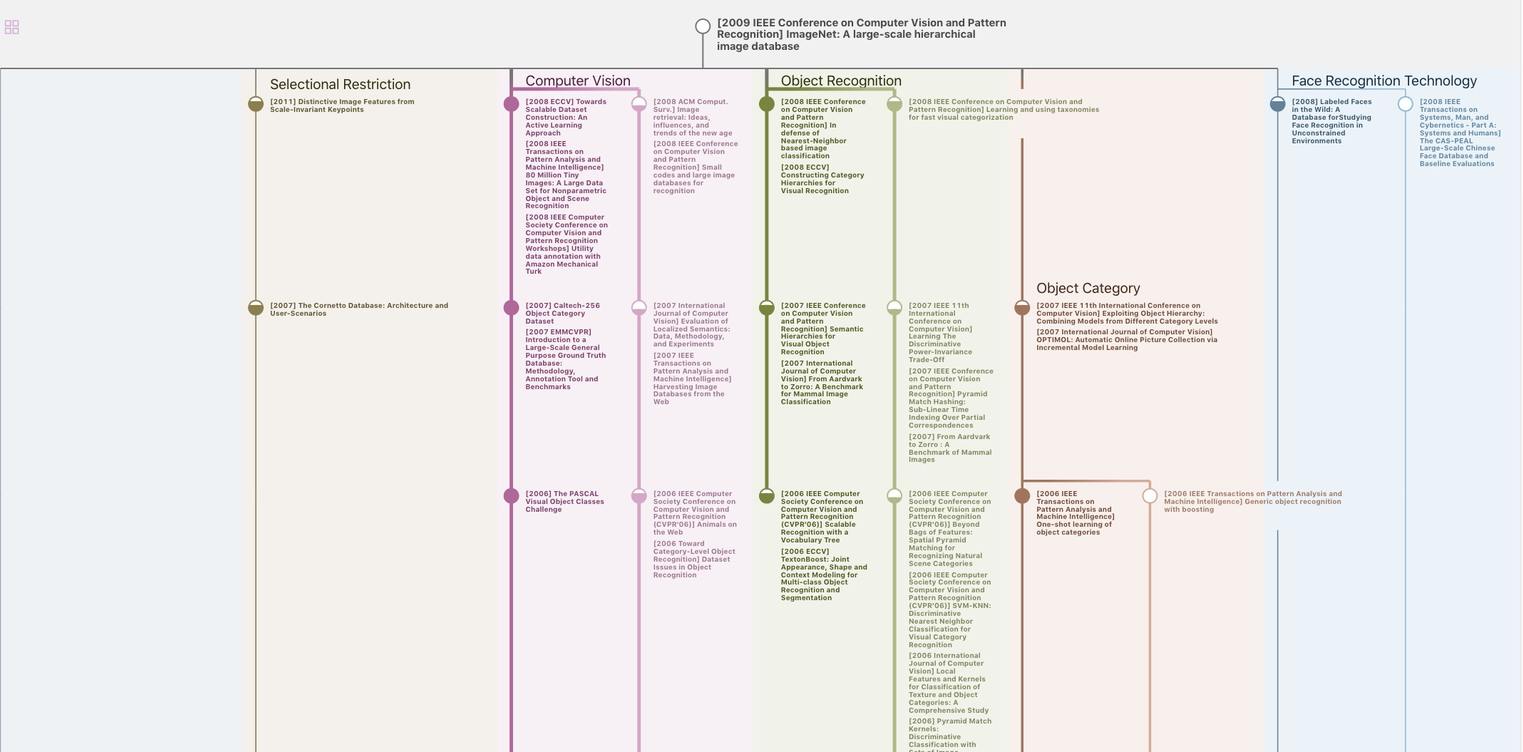
生成溯源树,研究论文发展脉络
Chat Paper
正在生成论文摘要