An Anti-Noise, Transferable, and Privacy-Preserving Real-Time Mobile User Authentication System With High Accuracy
IEEE Transactions on Mobile Computing(2023)
摘要
The authentication technology of mobile device users has been studied for decades. To balance security, privacy, and usability, motion sensors-based user authentication methods are widely investigated in recent years. However, existing studies meet the problems such as scarcity of training samples, underutilization of data, poor de-noising ability, insufficient transferability, privacy leakage, and low accuracy. To overcome these difficulties, we propose a system, called for Transferable, Real-time, Anti-noise, and Privacy-preserving mobile user COGnition., with the following capabilities: 1) In the phase of data collection, can eliminate man-made noise (mislabeling) through differential training based on down-sampling. 2) In the model training stage, the siamese neural network with Long Short-Term Memory (LSTM) as the sub-network is used to achieve sufficient coverage of sample patterns and the transferability of the model. 3) In the phase of real-world authentication, the privacy of the user is tremendously protected through end-side model deployment and local authentication. Experimental results on a dataset composed of 1,513 users with real-world noise show that TRAP COG has high accuracy and strong transferability, which is much better than state-of-the-art studies.
更多查看译文
关键词
anti-noise,privacy-preserving,real-time
AI 理解论文
溯源树
样例
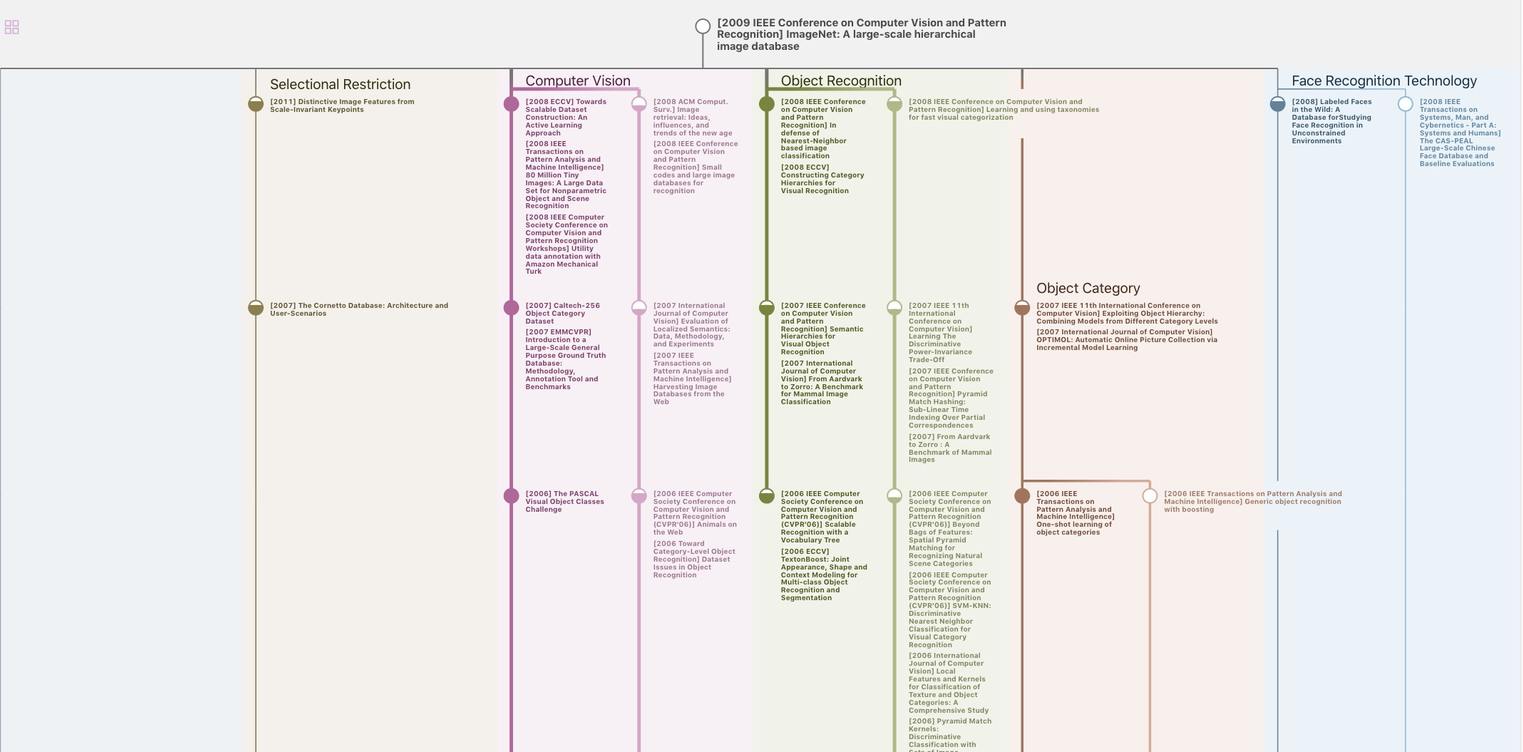
生成溯源树,研究论文发展脉络
Chat Paper
正在生成论文摘要