Abstract 5721: Automated annotation for large-scale clinicogenomic models of lung cancer treatment response and overall survival
Cancer Research(2023)
摘要
Abstract The digitization of health records and prompt availability of tumor DNA sequencing results offer a chance to study the determinants of cancer outcomes with unprecedented richness; however, abstraction of key attributes from free text presents a major limitation to large-scale analyses. Using natural language processing (NLP), we derived sites of metastasis, prior treatment at outside institutions, programmed death ligand 1 (PD-L1) levels, and smoking status from records of patients with tumor sequencing to create a richly annotated clinicogenomic cohort. We sought to define whether combining features would improve models of overall survival (OS) and treatment response as validated in a multi-institution, manually curated cohort. We leveraged the manually curated AACR GENIE Biopharma Collaborative (BPC) dataset to train NLP algorithms to abstract the aforementioned features from overlapping records available at Memorial Sloan Kettering (MSK). All models achieved precision and recall > 0.85. We deployed these algorithms to records of all MSK patients with non-small cell lung cancer (NSCLC) and tumor profiling with our FDA-authorized institutional targeted sequencing platform (N=7,015). These labels were combined with genomic, demographic, histopathologic, internal treatment and staging data to train random survival forests (RSF) to predict OS and time-to-next-treatment (TTNT) for molecularly targeted and immunotherapies. RSFs trained on the MSK NSCLC cohort were validated with the curated, non-MSK BPC NSCLC cohort (N=977). The addition of NLP-derived variables to genomic features enhanced RSF predictive power for OS (c-index, 10x bootstrap 95%CI: 0.58, 0.57-0.59 vs 0.75, 0.74-0.76 combined) and targeted and immunotherapy TTNT. The size of the MSK NSCLC cohort enabled discovery of associations between metastatic sites, PD-L1 status, genomics, and TTNTs not apparent in the smaller BPC cohort. We measured the added predictive value of variables not available in BPC with MSK-only cross-validation analyses. White blood cell differential counts and additional tissue genomic features including tumor mutational burden and fraction genome altered added minimally, while circulating tumor DNA sequencing added prognostic power for OS over other factors including disease burden Using NLP we present a large NSCLC cohort with rich clinicoradiographic annotation, leading to superior models of patient outcomes. Our data uncovers associations not observed in smaller, manually curated cohorts and provides a foundation for further research in therapy choice and prognostication. Citation Format: Justin Jee, Chris Fong, Karl Pichotta, Thinh Tran, Anisha Luthra, Mirella Altoe, Steven Maron, Ronglai Shen, Si-Yang Liu, Michele Waters, Joseph Kholodenko, Brooke Mastrogiacomo, Susie Kim, A Rose Brannon, Michael F. Berger, Axel Martin, Jason Chang, Anton Safonov, Jorge S. Reis-Filho, Deborah Schrag, Sohrab P. Shah, Pedram Razavi, Bob T. Li, Gregory J. Riely, Nikolaus Schultz. Automated annotation for large-scale clinicogenomic models of lung cancer treatment response and overall survival. [abstract]. In: Proceedings of the American Association for Cancer Research Annual Meeting 2023; Part 1 (Regular and Invited Abstracts); 2023 Apr 14-19; Orlando, FL. Philadelphia (PA): AACR; Cancer Res 2023;83(7_Suppl):Abstract nr 5721.
更多查看译文
关键词
lung cancer treatment response,automated annotation,lung cancer,clinicogenomic models,large-scale
AI 理解论文
溯源树
样例
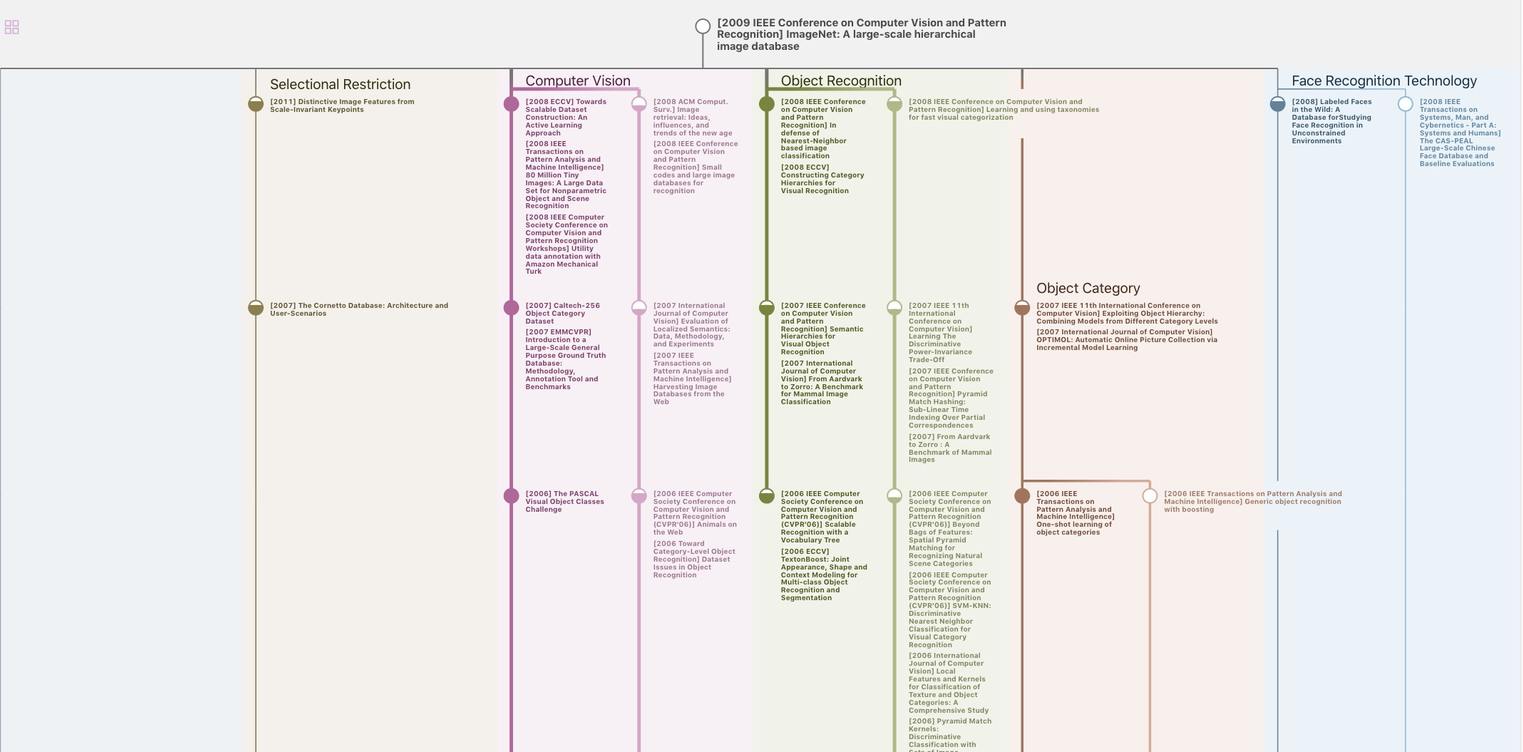
生成溯源树,研究论文发展脉络
Chat Paper
正在生成论文摘要