Survival prediction of NLST lung cancer patients from radiomics and clinical data
Research Square (Research Square)(2023)
摘要
Abstract Background : It is critical to accurately predict the survival likelihood of cancer patients to allow the best care and treatment. Publicly available datasets have emerged recently, such as the National Lung Screening Trial (NLST) data with low-dose computed tomography (LDCT) scans of at-risk populations. Recent research focuses primarily on improving survival prediction performance by developing more complex models without sufficient focus on interpretability. In contrast, this study focuses on identifying and analyzing the importance of different radiology features and clinical variables in survival prediction. Methods : This research used the NLST data with two widely-used predictive models - the Cox proportional hazards (CPH) model and the random survival forests (RSF). The first step was to generate semi-automated primary nodule annotations for an NLST subset of adenocarcinoma patients with the close help of a radiologist and extract commonly-used radiomic features to characterize these primary nodules. By coupling the radiomic features with the patient's clinical data, the models predict death by lung cancer from the first LDCT scan. The next step is to construct smaller subsets of influential features and demonstrates that these subsets preserve survival performance. Additionally, this study investigated the potential of combining radiomic features and clinical data in survival prediction. Lastly, to make similar studies on the NLST more feasible, the semi-automated nodule segmentations for the NLST subset used in this study were provided for public use along with the code for the experiments at https://github.com/hleu/survival_nlst. Results : The best result of 67.06 C-index and a mean time-dependent area under the receiver operating characteristic (TD-AUC) of 71.27 was obtained by using CPH models with a combination of a subset of clinical features and shape-based radiomic features. Conclusions : The first contribution is the nodule annotation, segmentation, and exact feature extraction from the NLST dataset. This annotated dataset increases the model performance for lung cancer prognosis. Secondly, this study applied two different survival analysis methods to radiology and clinical features and compared the results obtained from different techniques. The models with a combination of features can outperform the model with only radiomics or clinical features.
更多查看译文
关键词
nlst lung cancer patients,lung cancer,radiomics,cancer patients
AI 理解论文
溯源树
样例
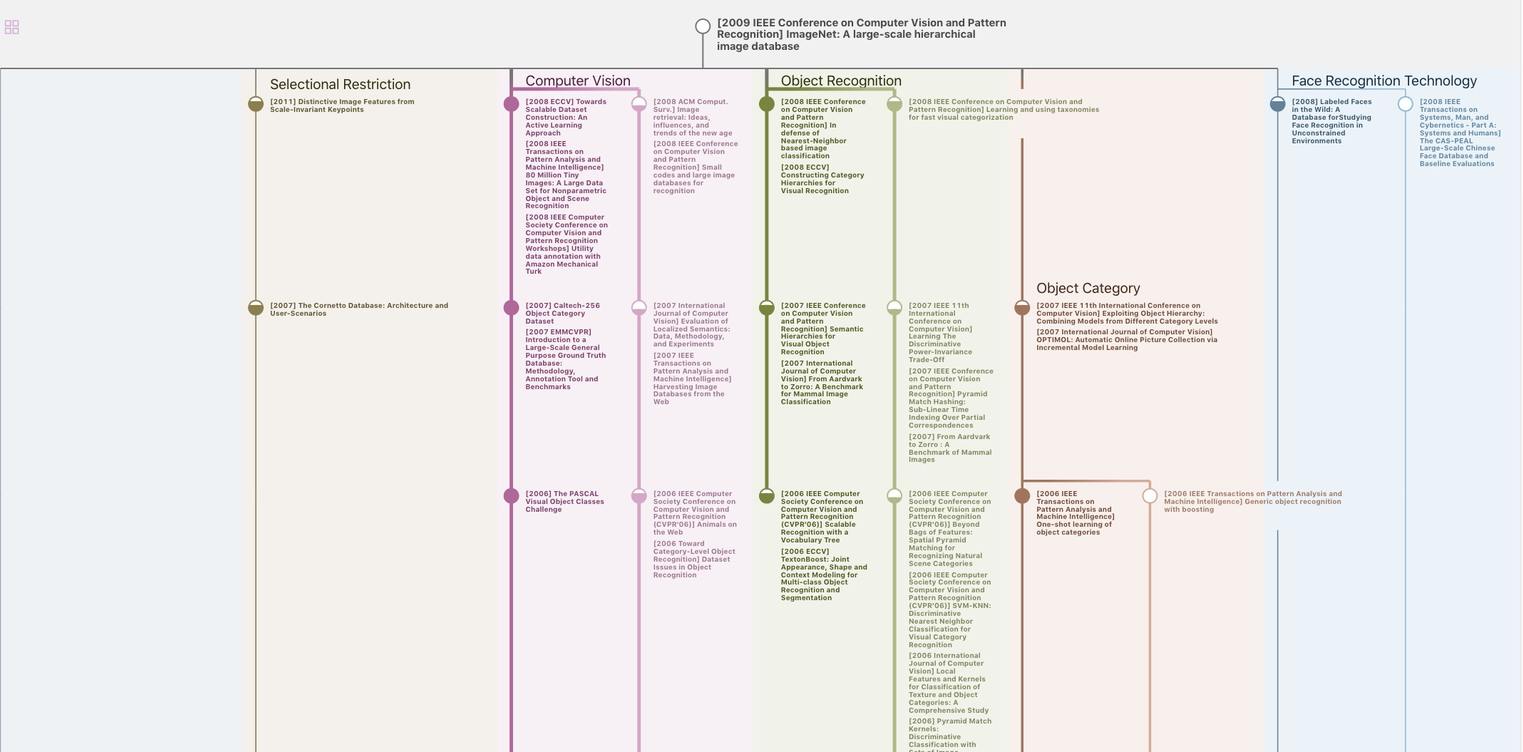
生成溯源树,研究论文发展脉络
Chat Paper
正在生成论文摘要