Polar Grid Based Point Cloud Ground Segmentation
Communications in computer and information science(2023)
摘要
The processing of 3D point clouds from laser scanning is still challenging in self-driving perception and positioning community. Ground segmentation is the key topic, splitting out the ground point cloud effectively reduces the amount of data and increases the speed of subsequent point cloud clustering and feature point extraction. The ground points segmented in SLAM can be used as constraints for back-end optimisation, improving the accuracy of map building and localisation. After the ground segmentation is completed, it can be used as a passable area for vehicle path planning. Existing approaches are based on the assumption that the ground is plane, but unfortunately the ground is not a plane, with a large number of slopes, roadsides, and parts of the ground even rugged and full of obstacles. In order to solve the ground segmentation problem, this paper proposes a ground segmentation method based on polar grid. The main contributions of this paper include: (1) We divide a frame of point cloud space into several regions, each of which is divided into several grids, based on the scanning characteristics of the LIDAR. (2) The plane was fitted based on the improved RANSAC algorithm for each of the previously divided grids. Experiments with KITTI and campus real environment datasets show that the sensitivity of our proposed method can reach more than 98% and the specificity is below 10%. The proposed algorithm can effectively and correctly separate the ground from the point cloud, even on slopes and the ground with many obstacles.
更多查看译文
关键词
segmentation,grid,ground,cloud
AI 理解论文
溯源树
样例
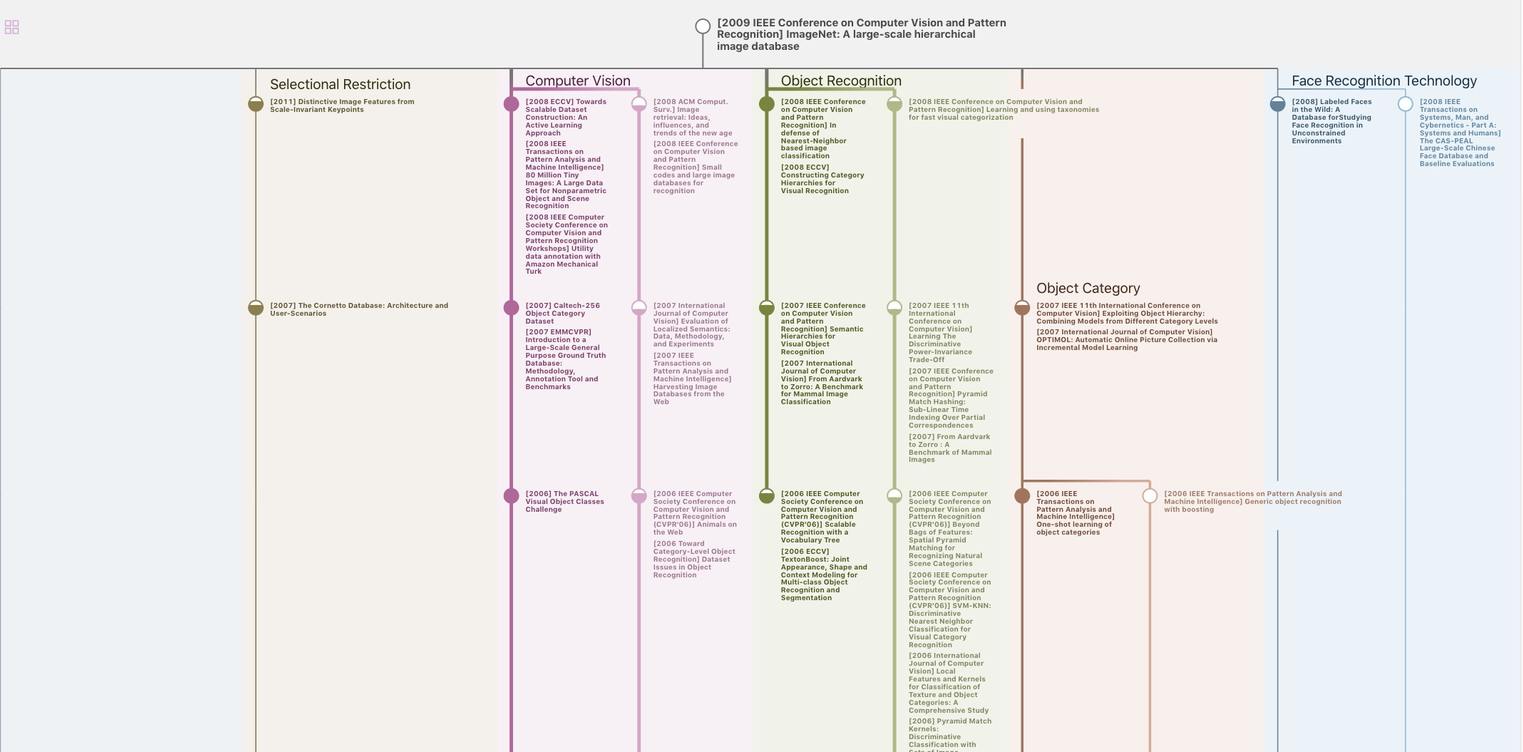
生成溯源树,研究论文发展脉络
Chat Paper
正在生成论文摘要