Benchmarking Continuous Time Models for Predicting Multiple Sclerosis Progression
arXiv (Cornell University)(2023)
摘要
Multiple sclerosis is a disease that affects the brain and spinal cord, it can lead to severe disability and has no known cure. The majority of prior work in machine learning for multiple sclerosis has been centered around using Magnetic Resonance Imaging scans or laboratory tests; these modalities are both expensive to acquire and can be unreliable. In a recent paper it was shown that disease progression can be predicted effectively using performance outcome measures and demographic data. In our work we build on this to investigate the modeling side, using continuous time models to predict progression. We benchmark four continuous time models using a publicly available multiple sclerosis dataset. We find that the best continuous model is often able to outperform the best benchmarked discrete time model. We also carry out an extensive ablation to discover the sources of performance gains, we find that standardizing existing features leads to a larger performance increase than interpolating missing features.
更多查看译文
关键词
multiple sclerosis
AI 理解论文
溯源树
样例
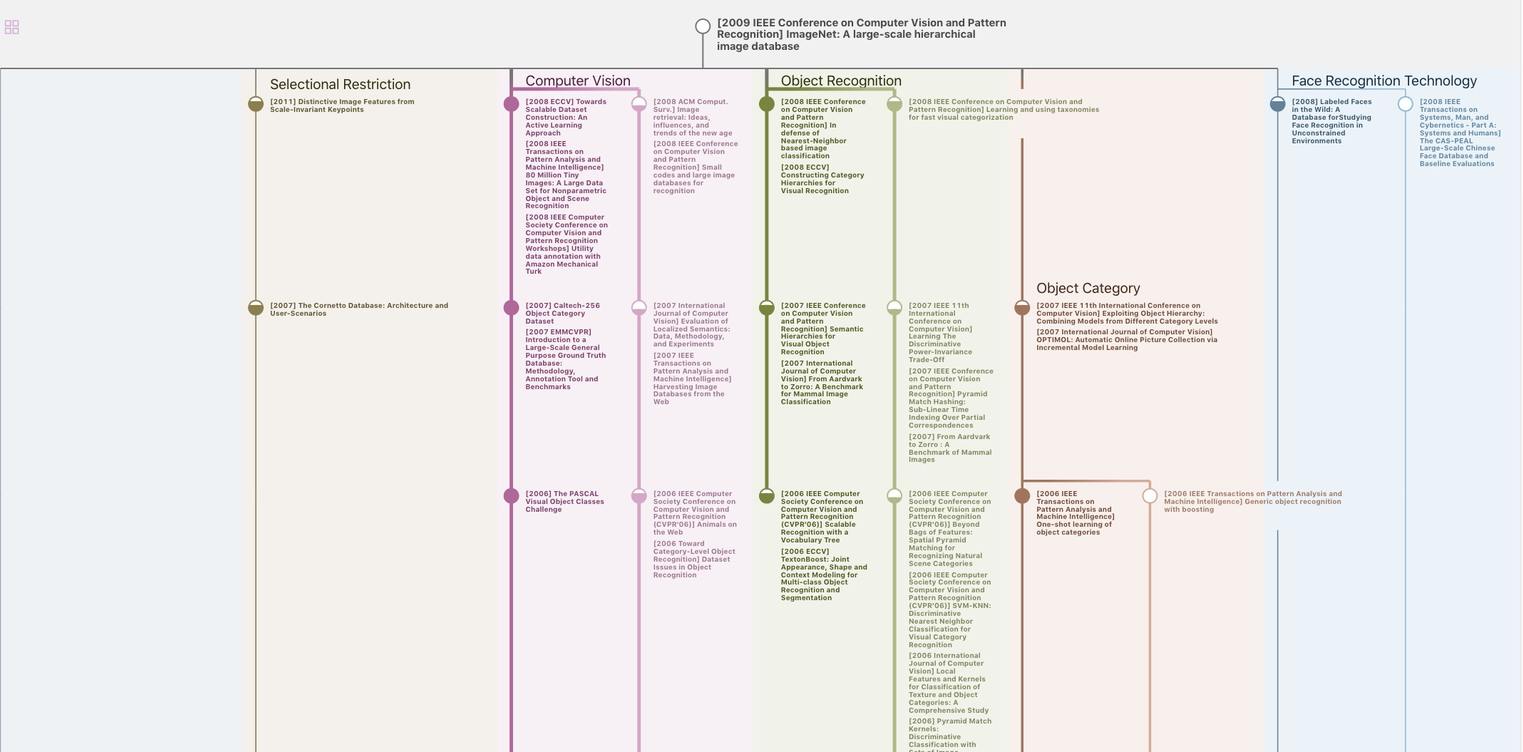
生成溯源树,研究论文发展脉络
Chat Paper
正在生成论文摘要