Leukocyte deep learning classification assessment using Shapley additive explanations algorithm
International Journal of Laboratory Hematology(2023)
摘要
A peripheral blood smear is a basic test for hematological disease diagnosis. This test is performed manually in many places worldwide, which requires both time and qualified staff. Large laboratories are equipped with digital morphology analyzers, some of which are based on deep learning methods. However, it is difficult to explain to scientists how they work. In this paper, we proposed to add an explanatory factor to enhance the interpretability of deep learning models in leukocyte classification.10 297 single images of leukocytes obtained from peripheral blood smears were included in this study. Pre-trained and fully trained VGG16 and VGG19 models were used to classify the leukocytes, and Shapley Additive Explanations (SHAP) DeepExplainer was applied to visualize the area of cells that were significant for classification. The output images from the DeepExplainer were compared with cellular elements that are essential to laboratory practice.The accuracy of our fully trained models was 99.81% for VGG16 and 99.79% for VGG19. It achieved slightly better results than the partially trained model, which scored 98.67% for VGG16 and 98.33% for VGG19. Their SHAP explanations indicated the significance of cellular structures in microscopic examination. Explanations in the pre-trained models have proved the cell and nucleus contours to be relevant to classification, while explanations in the fully trained models pointed to the cytoplasm area.Despite different SHAP DeepExplainer explanations for fully and partially trained models, this method appears to be helpful for the verification of leukocyte classification in automated peripheral blood smear examination.
更多查看译文
关键词
additive explanations,classification assessment,deep learning,shapley
AI 理解论文
溯源树
样例
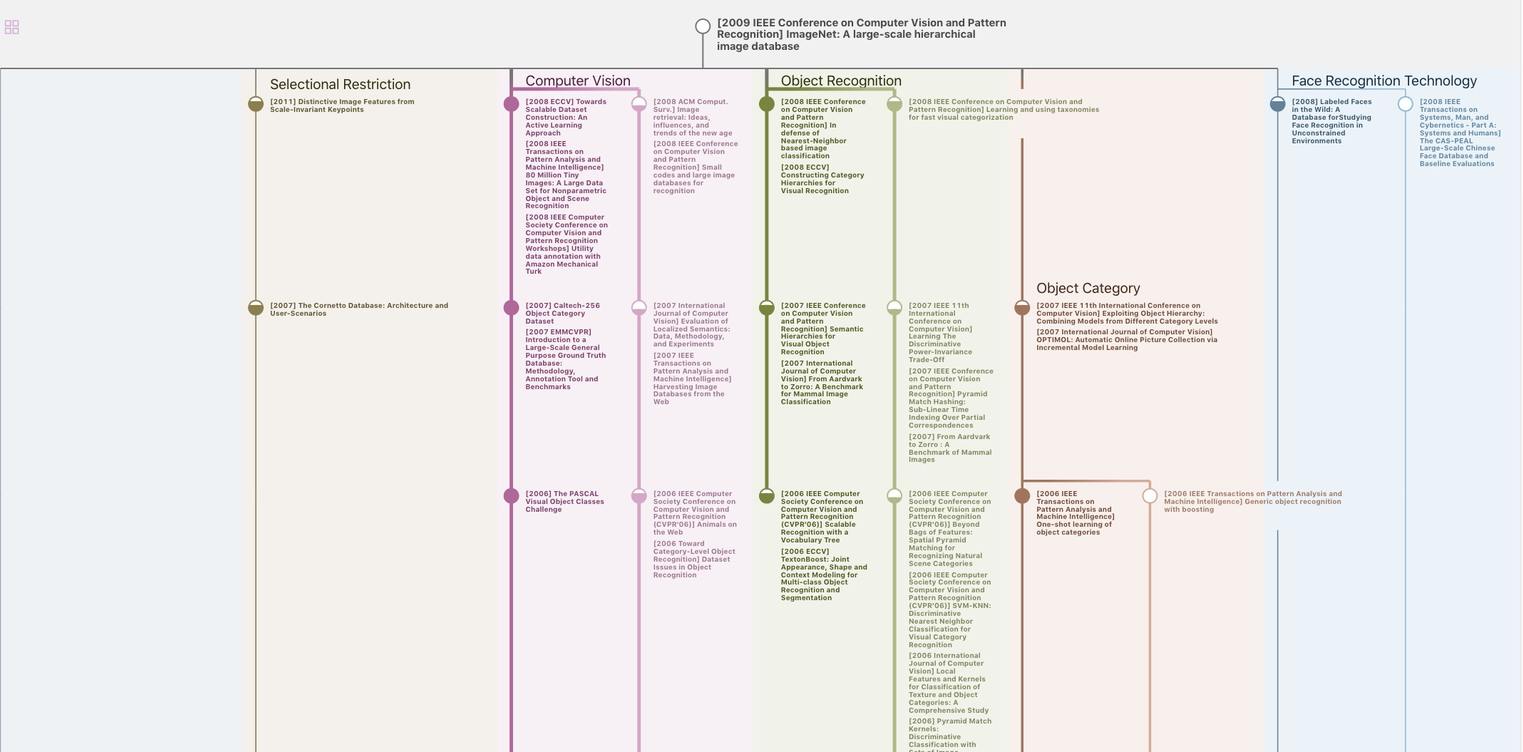
生成溯源树,研究论文发展脉络
Chat Paper
正在生成论文摘要