Inferring context-dependent computations through linear approximations of prefrontal cortex dynamics
bioRxiv (Cold Spring Harbor Laboratory)(2023)
摘要
Abstract The complex neural population activity of prefrontal cortex (PFC) is a hallmark of cognitive processes. How these rich dynamics emerge and support neural computations is largely unknown. Here, we infer mechanisms underlying the context-dependent selection and integration of sensory inputs by fitting dynamical models to PFC population responses of behaving monkeys. A class of models implementing linear dynamics driven by external inputs accurately captured the PFC responses within each context, achieving performance comparable to models without linear constraints. Two distinct mechanisms of input selection and integration were equally consistent with the data. One implemented context-dependent recurrent dynamics, as previously proposed, and relied on transient input amplification. The other relied on the subtle contextual modulation of the inputs, providing quantitative constraints on the attentional effects in sensory areas required to explain flexible PFC responses and behavior. Both mechanisms consistently revealed properties of inputs and recurrent dynamics missing in more simplified, incomplete descriptions of PFC responses. By revealing mechanisms consistent with rich cortical dynamics, our modeling approach provides a principled and general framework to link neural population activity and computation.
更多查看译文
关键词
linear approximations,context-dependent
AI 理解论文
溯源树
样例
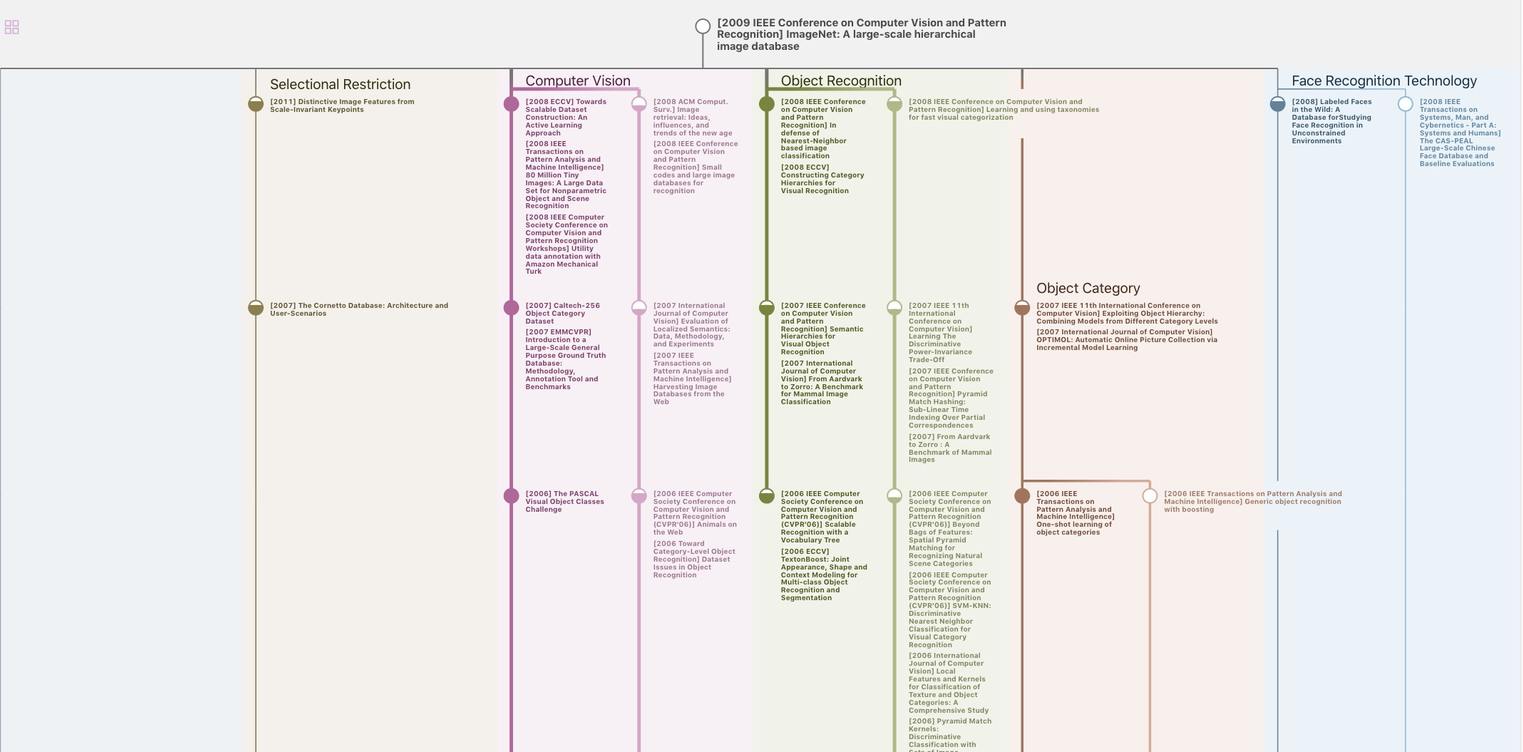
生成溯源树,研究论文发展脉络
Chat Paper
正在生成论文摘要