QoS Prediction and Adversarial Attack Protection for Distributed Services Under DLaaS
IEEE TRANSACTIONS ON COMPUTERS(2024)
摘要
Deep-Learning-as-a-service (DLaaS) has received increasing attention due to its novelty as a diagram for deploying deep learning techniques. However, DLaaS faces performance and security issues that urgently need to be addressed. Given the limited computation resources and concern of benefits, Quality-of-Service (QoS) metrics should be revised to optimize the performance and reliability of distributed DLaaS systems. New users and services dynamically and continuously join and leave such a system, resulting in cold start issues, and additionally, the increasing demand for robust network connections requires the model to evaluate the uncertainty. To address such performance problems, we propose in this article a deep learning-based model called embedding enhanced probability neural network, in which information is extracted from inside the graph structure and then estimated the mean and variance values for the prediction distribution. The adversarial attack is a severe threat to model security under DLaaS. Due to such, the service recommender system's vulnerability is tackled, and adversarial training with uncertainty-aware loss to protect the model in noisy and adversarial environments is investigated and proposed. Extensive experiments on a large-scale real-world QoS dataset are conducted, and comprehensive analysis verifies the robustness and effectiveness of the proposed model.
更多查看译文
关键词
Quality of service,Internet of Things,Computational modeling,Deep learning,Security,Performance evaluation,Predictive models,Adversarial attacks,dlaas,graph neural network,probability forecast,qos prediction
AI 理解论文
溯源树
样例
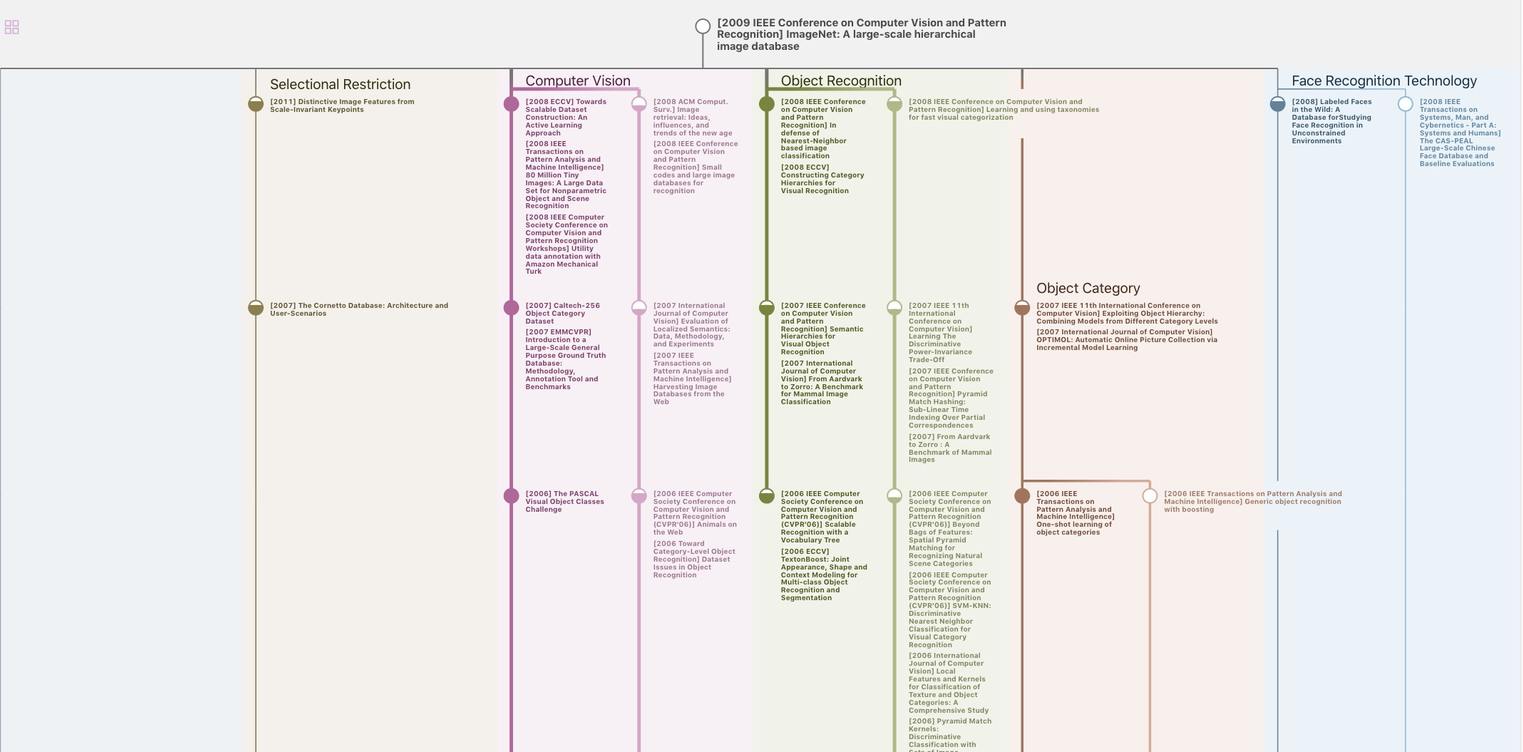
生成溯源树,研究论文发展脉络
Chat Paper
正在生成论文摘要