DETECT: Feature extraction method for disease trajectory modeling in electronic health records.
PubMed(2023)
摘要
Modeling with longitudinal electronic health record (EHR) data proves challenging given the high dimensionality, redundancy, and noise captured in EHR. In order to improve precision medicine strategies and identify predictors of disease risk in advance, evaluating meaningful patient disease trajectories is essential. In this study, we develop the algorithm DiseasE Trajectory fEature extraCTion (DETECT) for feature extraction and trajectory generation in high-throughput temporal EHR data. This algorithm can 1) simulate longitudinal individual-level EHR data, specified to user parameters of scale, complexity, and noise and 2) use a convergent relative risk framework to test intermediate codes occurring between specified index code(s) and outcome code(s) to determine if they are predictive features of the outcome. Temporal range can be specified to investigate predictors occurring during a specific period of time prior to onset of the outcome. We benchmarked our method on simulated data and generated real-world disease trajectories using DETECT in a cohort of 145,575 individuals diagnosed with hypertension in Penn Medicine EHR for severe cardiometabolic outcomes.
更多查看译文
关键词
disease trajectory modeling,electronic health records,feature extraction method
AI 理解论文
溯源树
样例
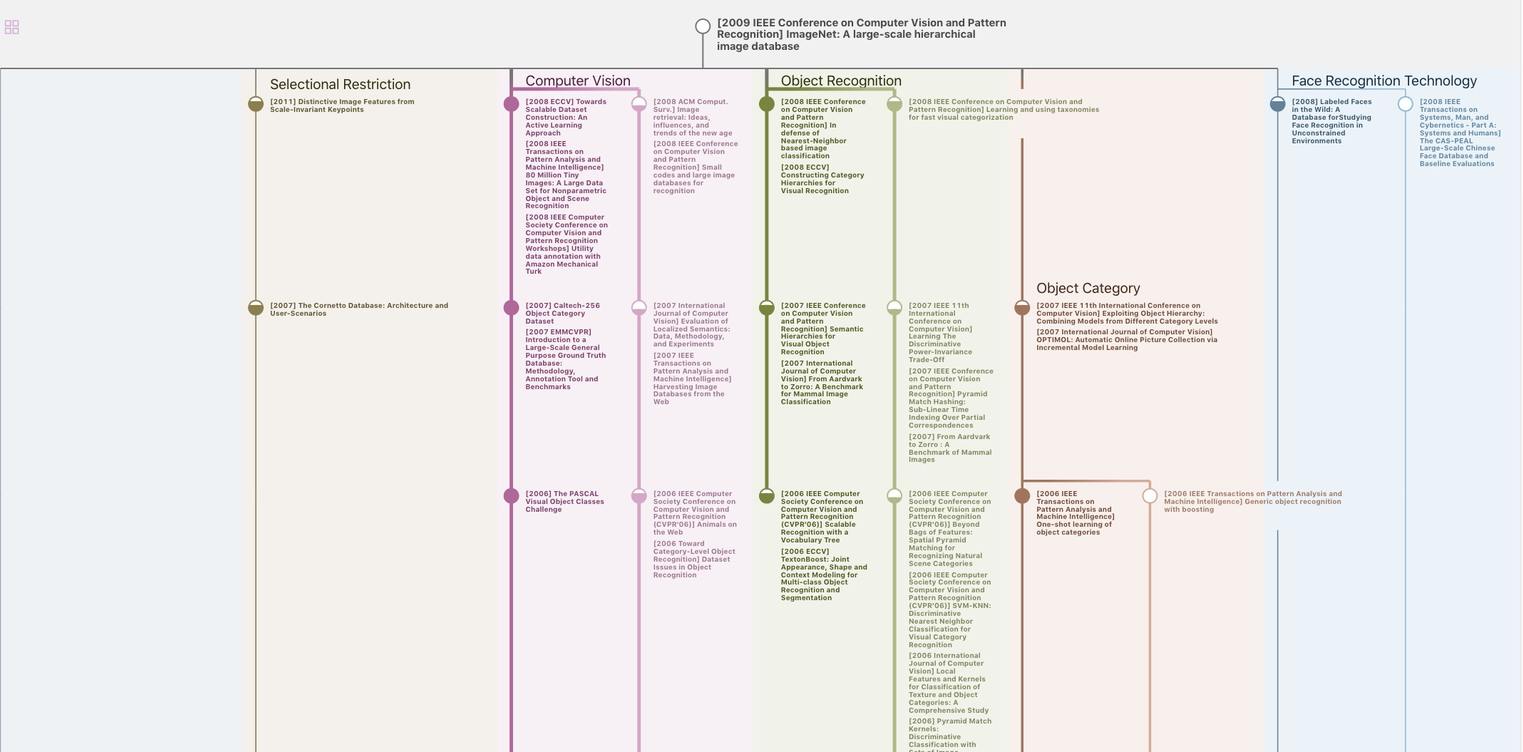
生成溯源树,研究论文发展脉络
Chat Paper
正在生成论文摘要