Deep Self-Supervised Graph Attention Convolution Autoencoder for Networks Clustering
IEEE TRANSACTIONS ON CONSUMER ELECTRONICS(2023)
摘要
In recent years, graph-based deep learning algorithms have attracted widespread attention in the field of consumer electronics. Still, most of the current graph neural networks are based on supervised learning or semi-supervised learning, which often relies on the true labels of the given samples as auxiliary information. To solve this problem, we propose a Deep Self-Supervised Attention Convolution Autoencoder Graph Clustering (DSAGC) model and use it for social networks clustering. We divide the proposed model into two parts: a pretext task and a downstream task. In the pretext task, we obtain pseudo-labels of samples by graph attention autoencoder and clustering, then adopt the proposed reliable sample selection mechanism to gain a high confidential sample. These samples and corresponding pseudo-labels are selected as input of downstream task for helping the downstream task to finish training. The model obtains the predictive labels of the sample from the downstream task. This model does not depend on the true label of the sample for training and uses the pseudo-labels produced by unsupervised learning to improve the clustering performance. We apply the proposed model to three commonly used public social network datasets to test its performance. Compared with the presented unsupervised clustering algorithms and other deep graph neural network algorithms, various metrics have shown that the proposed model consistently outperforms the state-of-the-art. The proposed method can be used for consumer behavior analysis. We release the source code at https://github.com/hulu88/DSAGC.
更多查看译文
关键词
Self-supervised,autoencoder,reliable sample selection,social networks clustering
AI 理解论文
溯源树
样例
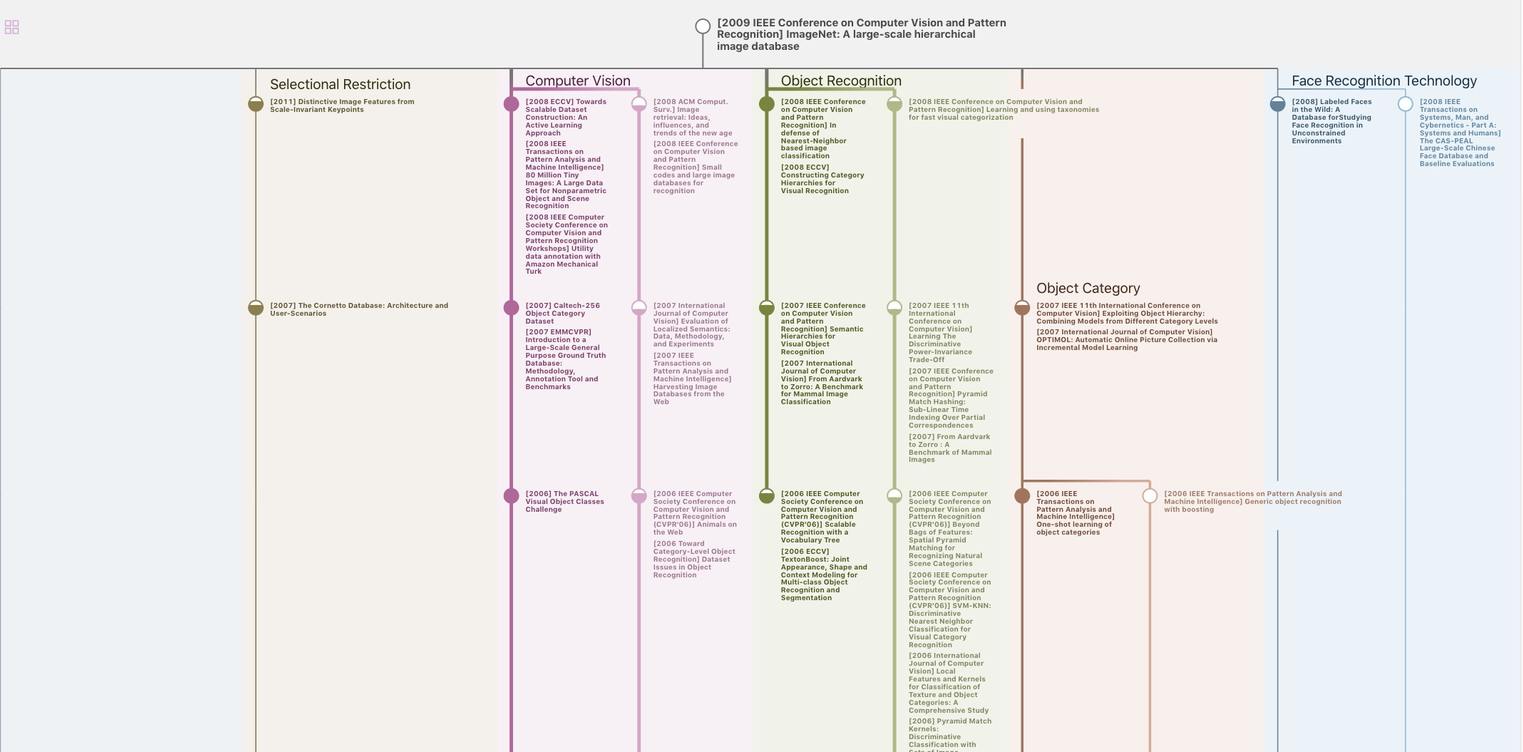
生成溯源树,研究论文发展脉络
Chat Paper
正在生成论文摘要