A Hybrid Bearing Prognostic Method With Fault Diagnosis and Model Fusion
IEEE TRANSACTIONS ON INDUSTRIAL INFORMATICS(2024)
摘要
Accurate bearing fault diagnosis and prognosis (FDP) is critical for optimal maintenance schedules, safety and reliability. The existing methods face some problems and challenges in detecting the starting time for prognosis and using a single model to describe fault dynamics. With these motivations, this article presents a hybrid bearing FDP framework with fault detection and automatic fault model selection. In the proposed approach, the convolutional neural network is used to detect fault and select the appropriate fault dynamic model. To improve the performance, power spectrum of vibration signals are fused with operating conditions to built information maps for fault detection and model selection. After a fault is detected, a Bayesian method is triggered to estimate the fault state and predict the remaining useful life. In the prognosis, the Dempster-Shafer theory is employed to fuse prediction results from different models if necessary. The proposed approach is verified with bearings under different operating conditions. Experimental results and comparison studies verify the high accuracy and efficiency of the proposed method.
更多查看译文
关键词
Bearing,continuous wavelet transform (CWT),convolutional neural network (CNN),Dempster-Shafer theory (DST),fault model selection,particle filtering
AI 理解论文
溯源树
样例
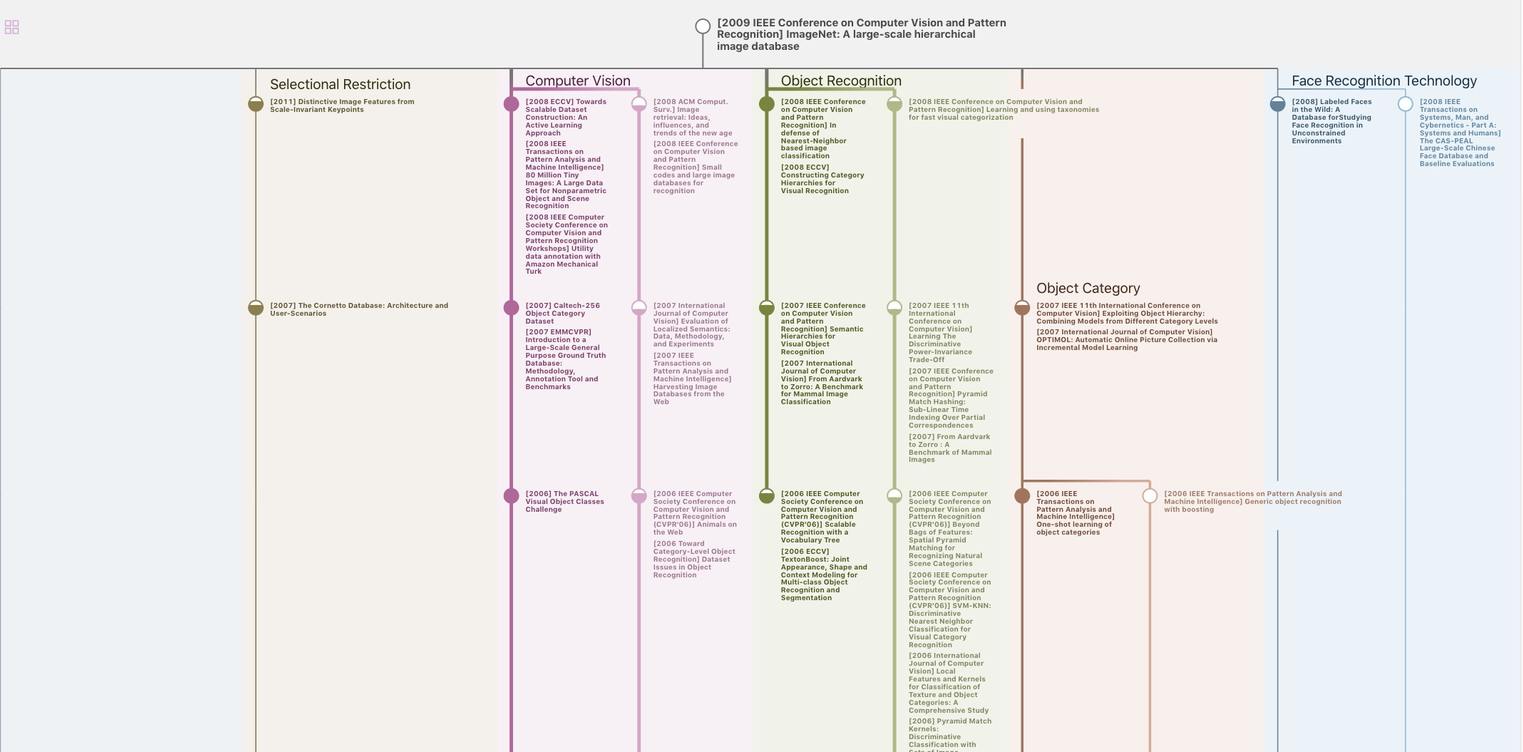
生成溯源树,研究论文发展脉络
Chat Paper
正在生成论文摘要