Entropy removal of medical diagnostics
Scientific Reports(2024)
摘要
Shannon entropy is a core concept in machine learning and information theory, particularly in decision tree modeling. To date, no studies have extensively and quantitatively applied Shannon entropy in a systematic way to quantify the entropy of clinical situations using diagnostic variables (true and false positives and negatives, respectively). Decision tree representations of medical decision-making tools can be generated using diagnostic variables found in literature and entropy removal can be calculated for these tools. This concept of clinical entropy removal has significant potential for further use to bring forth healthcare innovation, such as quantifying the impact of clinical guidelines and value of care and applications to Emergency Medicine scenarios where diagnostic accuracy in a limited time window is paramount. This analysis was done for 623 diagnostic tools and provided unique insights into their utility. For studies that provided detailed data on medical decision-making algorithms, bootstrapped datasets were generated from source data to perform comprehensive machine learning analysis on these algorithms and their constituent steps, which revealed a novel and thorough evaluation of medical diagnostic algorithms.
更多查看译文
关键词
diagnostics,removal
AI 理解论文
溯源树
样例
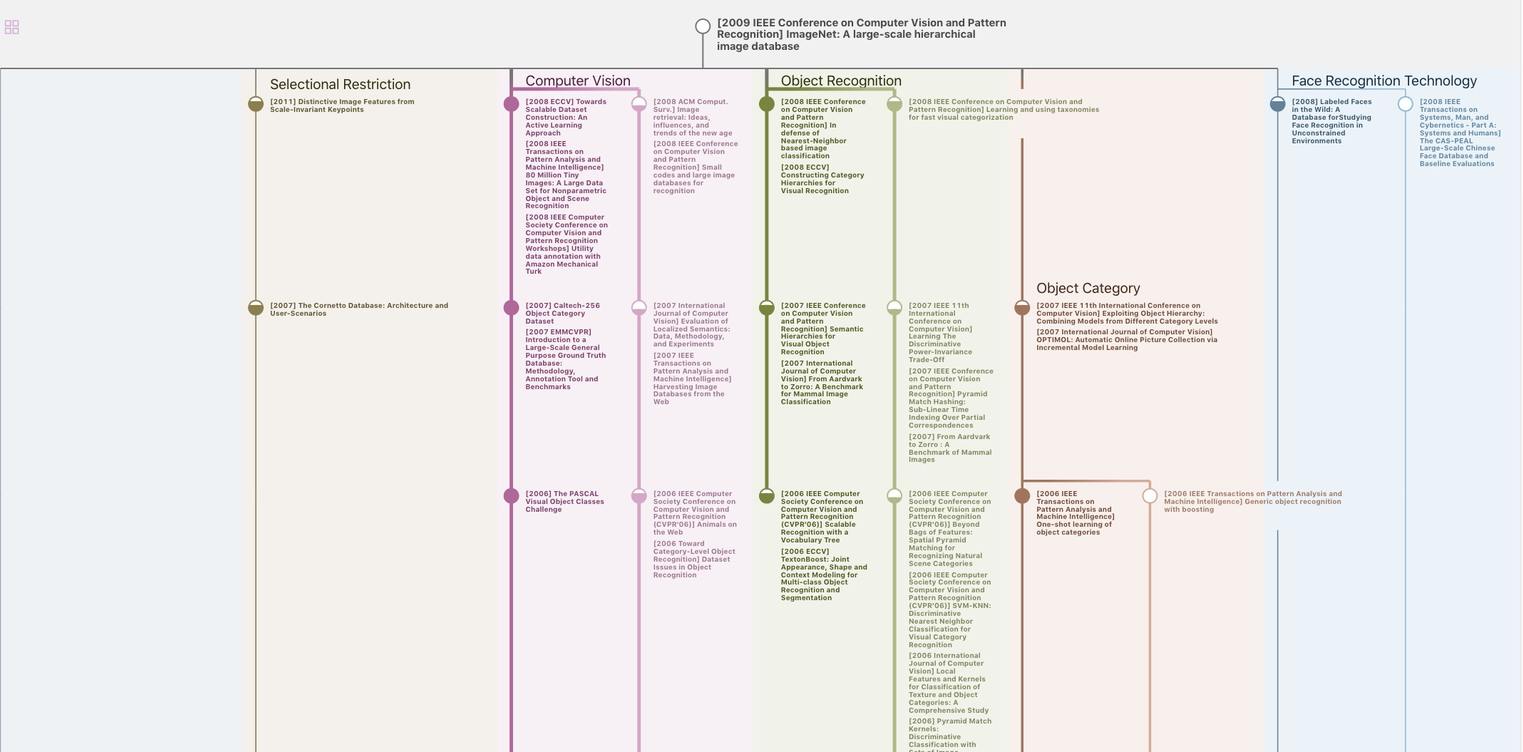
生成溯源树,研究论文发展脉络
Chat Paper
正在生成论文摘要