Amodal Intra-class Instance Segmentation: Synthetic Datasets and Benchmark
2024 IEEE/CVF Winter Conference on Applications of Computer Vision (WACV)(2024)
摘要
Images of realistic scenes often contain intra-class objects that are heavily occluded from each other, making the amodal perception task that requires parsing the occluded parts of the objects challenging. Although important for downstream tasks such as robotic grasping systems, the lack of large-scale amodal datasets with detailed annotations makes it difficult to model intra-class occlusions explicitly. This paper introduces two new amodal datasets for image amodal completion tasks, which contain a total of over 267K images of intra-class occlusion scenarios, annotated with multiple masks, amodal bounding boxes, dual order relations and full appearance for instances and background. We also present a point-supervised scheme with layer priors for amodal instance segmentation specifically designed for intra-class occlusion scenarios
1
. Experiments show that our weakly supervised approach outperforms the SOTA fully supervised methods, while our layer priors design exhibits remarkable performance improvements in the case of intra-class occlusion in both synthetic and real images.
更多查看译文
关键词
Algorithms,Image recognition and understanding,Algorithms,Datasets and evaluations
AI 理解论文
溯源树
样例
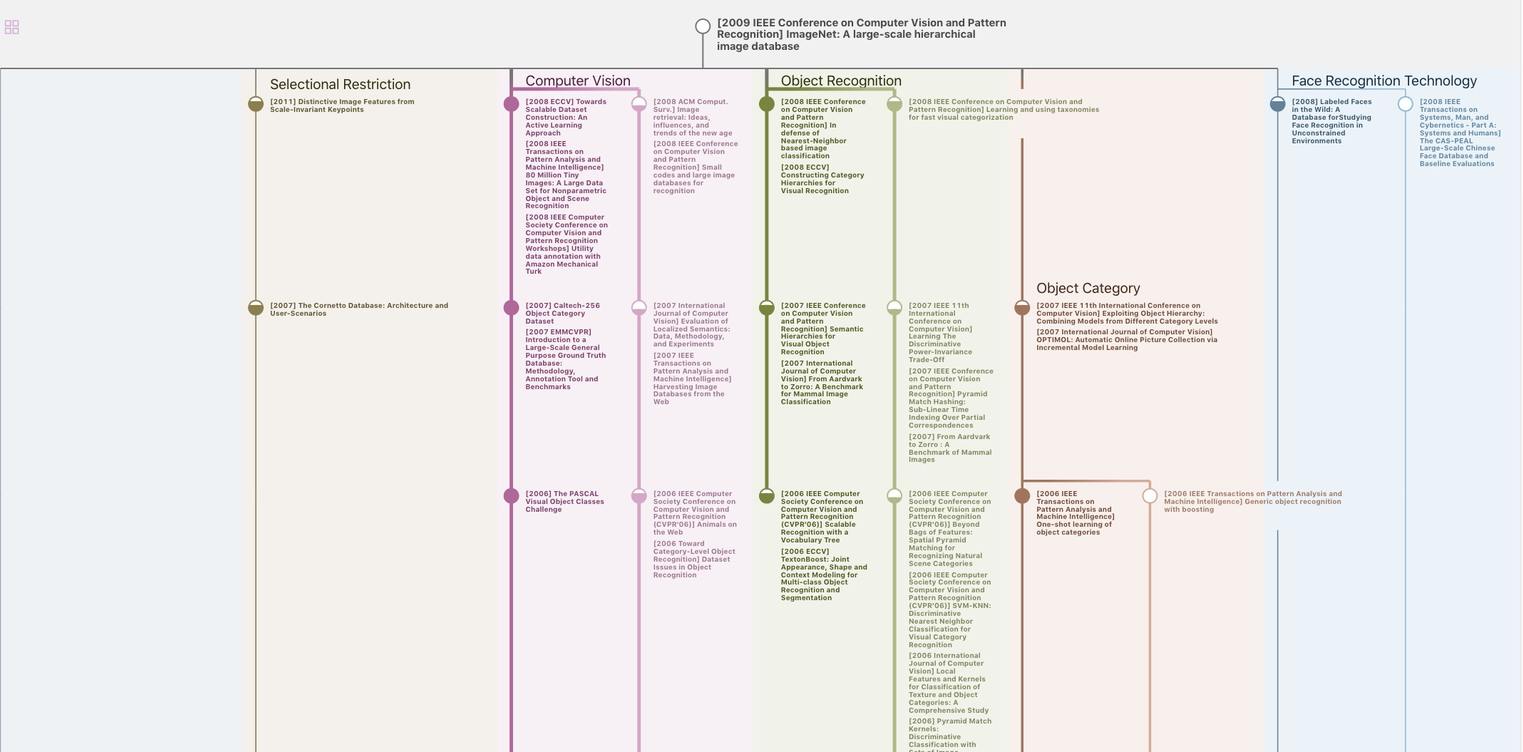
生成溯源树,研究论文发展脉络
Chat Paper
正在生成论文摘要