Probabilistic Electricity Price Forecast With Optimal Prediction Interval
IEEE TRANSACTIONS ON POWER SYSTEMS(2024)
摘要
The uncertainty of electricity prices poses a challenge to all market participants as their decisions highly depend on the accuracy of price forecasts. Prediction intervals become an efficient method to quantify the uncertainties that reside in electricity price forecasts. In this paper, we propose a probabilistic electricity price forecast with optimal prediction interval method that considers both reliability and sharpness requirements. Taking reliability and sharpness into account, we ensure the prediction interval has a narrow width without sacrificing reliability. In the proposed method, the quantile regression is utilized to estimate the upper and lower bounds of the prediction intervals to avoid electricity price distribution assumption. In addition, the extreme learning machine (ELM) method is embedded in the forecast method to capture the nonlinear relationship within price data, and its tuning parameters are optimized using the augmented Lagrangian relaxation method in this paper. The effectiveness of the proposed probabilistic forecast method is demonstrated using data from various electricity markets.
更多查看译文
关键词
Predictive models,Probabilistic logic,Reliability,Power system reliability,Neural networks,Gaussian distribution,Mathematical models,Electricity price forecast,optimal prediction interval,probabilistic forecast
AI 理解论文
溯源树
样例
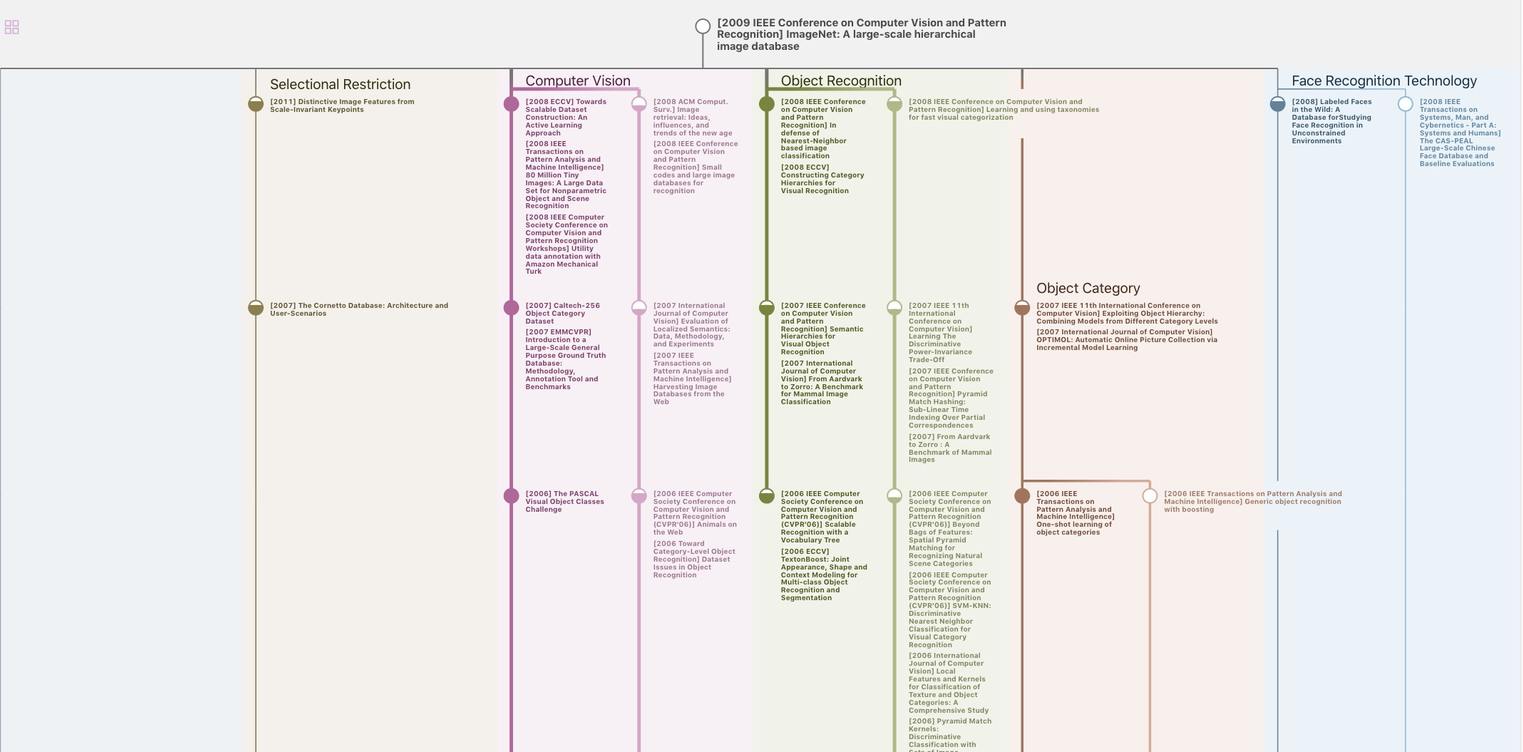
生成溯源树,研究论文发展脉络
Chat Paper
正在生成论文摘要