What's meant by explainable model: A Scoping Review
arXiv (Cornell University)(2023)
摘要
We often see the term explainable in the titles of papers that describe applications based on artificial intelligence (AI). However, the literature in explainable artificial intelligence (XAI) indicates that explanations in XAI are application- and domain-specific, hence requiring evaluation whenever they are employed to explain a model that makes decisions for a specific application problem. Additionally, the literature reveals that the performance of post-hoc methods, particularly feature attribution methods, varies substantially hinting that they do not represent a solution to AI explainability. Therefore, when using XAI methods, the quality and suitability of their information outputs should be evaluated within the specific application. For these reasons, we used a scoping review methodology to investigate papers that apply AI models and adopt methods to generate post-hoc explanations while referring to said models as explainable. This paper investigates whether the term explainable model is adopted by authors under the assumption that incorporating a post-hoc XAI method suffices to characterize a model as explainable. To inspect this problem, our review analyzes whether these papers conducted evaluations. We found that 81% of the application papers that refer to their approaches as an explainable model do not conduct any form of evaluation on the XAI method they used.
更多查看译文
关键词
explainable model,scoping review
AI 理解论文
溯源树
样例
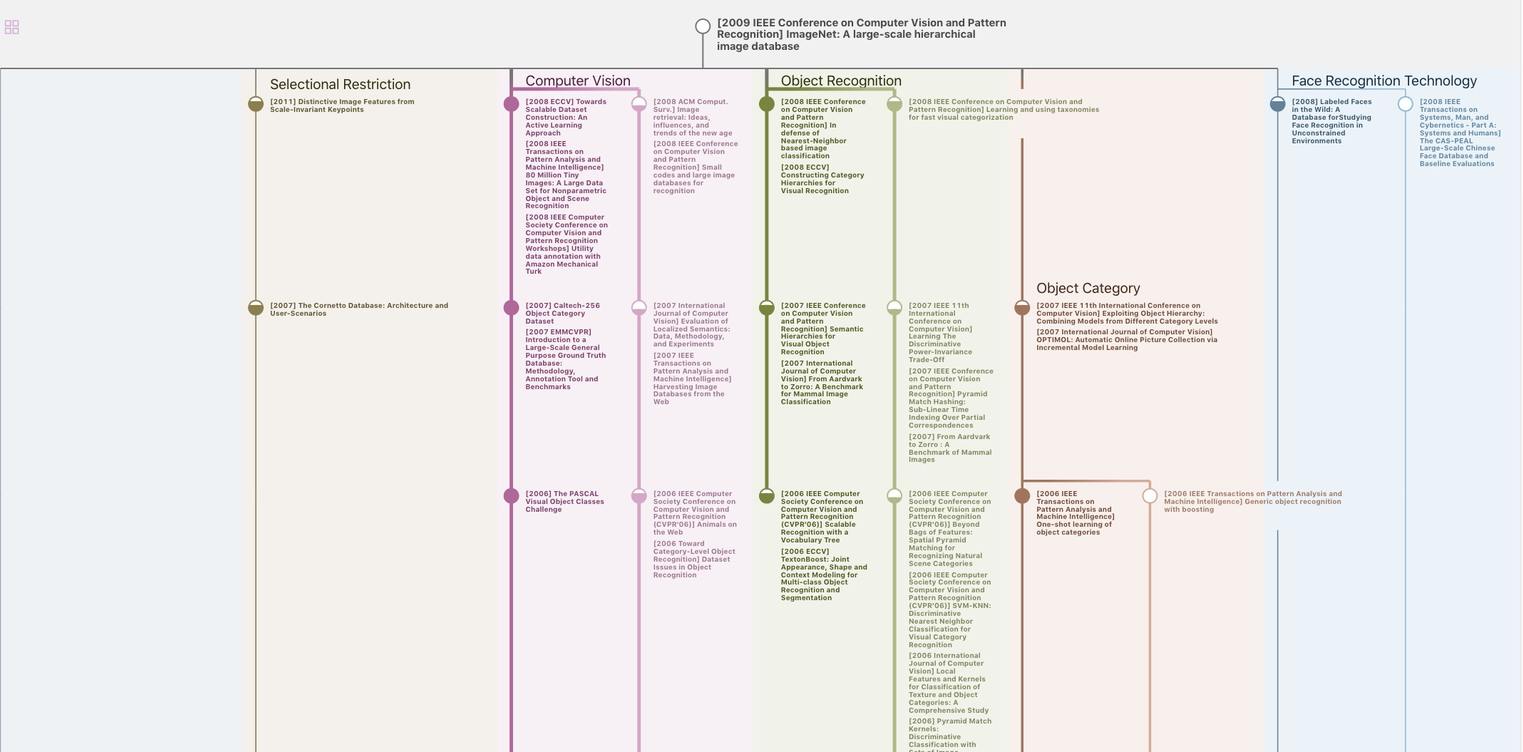
生成溯源树,研究论文发展脉络
Chat Paper
正在生成论文摘要